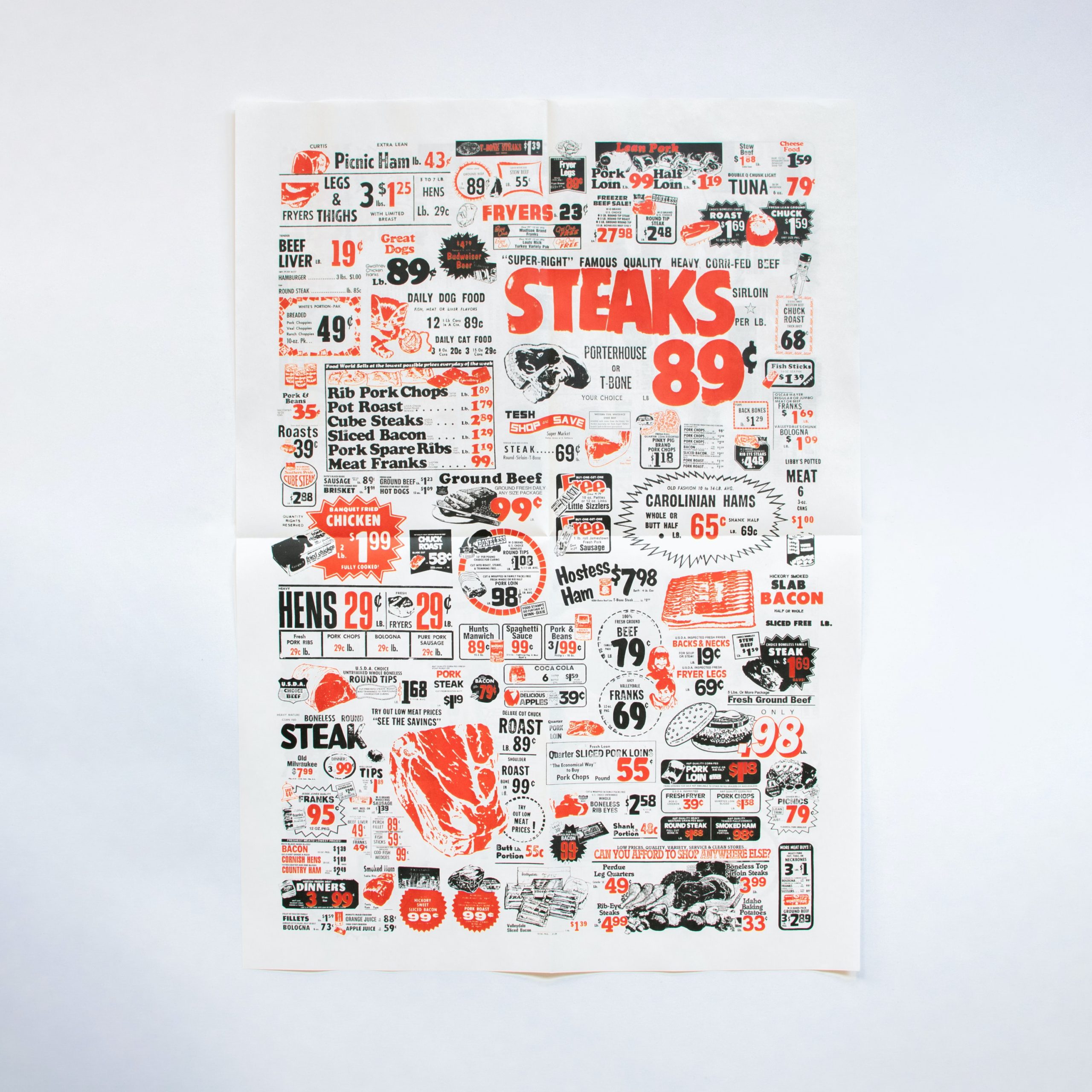
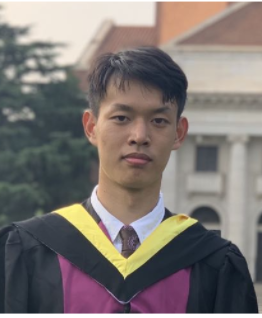
š¬ Research Summary by Renzhe Xu, a third year PhD student in Computer Science and Technology at Tsinghua University and his research interests include fair machine learning, causal inference, and data mining.
[Original paper by Renzhe Xu, Xingxuan Zhang, Peng Cui, Bo Li, Zheyan Shen, Jiazheng Xu]
Overview: Ā How can we regulate personalized pricing to ensure consumersā benefits? In this work, we propose two simple but effective regulatory policies under an idealized circumstance. Our findings and insights shed light on regulatory policy design for the increasingly monopolized business in the digital era.
Introduction
Personalized pricing is a business strategy to charge different prices to individual consumers based on their characteristics and behaviors. It has become common practice in many industries nowadays due to the availability of a growing amount of high granular consumer data. The discriminatory nature of personalized pricing has triggered heated debates among policymakers and academics on how to design regulation policies to balance market efficiency and equity. In this work, we propose two sound policy instruments and investigate the optimal pricing strategy of a profit-maximizing monopoly under both regulatory constraints. We analyze the impact of imposing the proposed policies on consumer surplus, producer surplus, and social welfare. We show that both proposed constraints can help balance consumer surplus and producer surplus at the expense of total surplus for common demand distributions.
Background
Personalized pricing, once considered the idealized construction of economic theories, has become common practice in many industries due to the availability of the increasing amount of consumer data. The main concern of personalized pricing is that it transfers value from consumers to shareholders, increasing inequality and ineļ¬ciency from a utilitarian standpoint. As a result, eļ¬ective regulatory policies are required to balance the beneļ¬ts between consumers and companies, which are measured as consumer surplus and producer surplus, respectively.
In this work, we study in designing eļ¬ective policy instruments to balance consumer surplus and producer surplus. We consider the most straightforward scenario where a monopoly sells a single product with ļ¬xed marginal cost to diļ¬erent consumers. In addition, we assume that the monopoly can precisely estimate each consumersā willingness to pay and the purpose of the monopoly is to ļ¬nd a personalized pricing strategy to maximize its revenue while remaining compliant with the policy instruments.
Proposed Policy instruments
We propose two sound policy instruments and prove their eļ¬ectiveness in balancing consumer surplus and producer surplus. These two policies, named the diļ¬erence and ratio constraints, are introduced to regulate the range of personalized prices by constraining the diļ¬erence and ratio between the maximal price and minimal price, respectively. For example, a $2-difference constraint allows a pricing range [$1, $3] (3 – 1 = 2) but disallows [$1, $4] (4 – 1 = 3 > 2) while, a 2-ratio constraint allows [$2, $4] (4 / 2 = 2) but disallows [$2, $5] (5 / 2 = 2.5 > 2).
The constant in the constraints (such as $2 in the $2-difference constraint shown above) determines the intensity of the regulation. A smaller constant will lead to a stricter regulatory intensity in both constraints. Now consider the most extreme cases. On the one hand, when the constant becomes 0 in the difference constraint or becomes 1 in the ratio constraint, the regulatory intensity is the most strict and only uniform pricing (the price is fixed for all consumers) is allowed. On the other hand, when the constant becomes big enough, the regulation is very slight and perfect price discrimination is possible.
Theoretical results on consumer surplus, producer surplus, and social welfare
The results on social welfare analysis under both constraints are threefold. Graphical explanations of these findings are shown in the figure.
1. Both constraints can effectively balance the consumer surplus and producer surplus, which means the consumer surplus increases while the producer surplus decreases as the constraints become stricter.
2. We compare the trade-off between consumer surplus and producer surplus achieved by the two constraints and show that the difference constraint outperforms the ratio constraint. This means that the consumer surplus under the ratio constraint is smaller than that under the difference constraint if the producer surplus under the two constraints is equal.
3. Imposing either of the constraints will inevitably, to some extent, harm the total surplus. This result is reasonable, given that the efficiency-equity trade-off is largely recognized in practice. In addition, the perfect price discrimination achieves the maximal market efficiency and any regulatory policies to avoid it will inevitably harm the total surplus.
Empirical results
We run experiments on several real-world datasets to verify our theoretical results. The details of the datasets are provided as follows.
1. Coke and cake. Wertenbroch and Skiera [2002] adopted Becker, DeGroot, and Marschakās method to estimate willingness-to-pay for a can of Coca-Cola on a public beach and a piece of pound cake on a commuter ferry in Kiel, Germany.
2. Elective vaccine. Slunge [2015] studied willingness to pay for vaccination against tick-borne encephalitis in Sweden. They asked individuals with personal information about take-up at a random price of 100, 250, 500, 750, or 1000 SEK.
3. Auto loan. The dataset records 208,085 auto loan applications received by a major online lender in the United States with loan-speciļ¬c features. Following prior works, we adopt the feature selection results and consider only four features. The price of a loan is computed as the net present value of future payment minus the loan amount.
The trade-off curves between consumer surplus and producer surplus under our proposed constraints in these datasets are shown in the figure. The figure verifies that both constraints can help balance consumer surplus and producer surplus at the expense of total surplus. In addition, the curve of the ratio constraint is on top of the diļ¬erence constraint.
Between the lines
We should notice that it is important to analyze the impacts of policies in fair personalized pricing theoretically. Improperly designed policies may harm both consumers and producers. For example, DubĆ© and Misra [2021] found that ļ¬ner-grained personalized pricing in third-degree price discrimination can increase consumer welfare, which is contrary to the common belief that personalized pricing will always harm consumers.
In addition, comparatively, the ratio constraint is more suitable for designing policies because ratios could be easily adapted to various scenarios. The difference constraint has better performance on the trade-oļ¬ between consumer surplus and producer surplus. As a result, the two constraints could be adopted in different applications in practice.