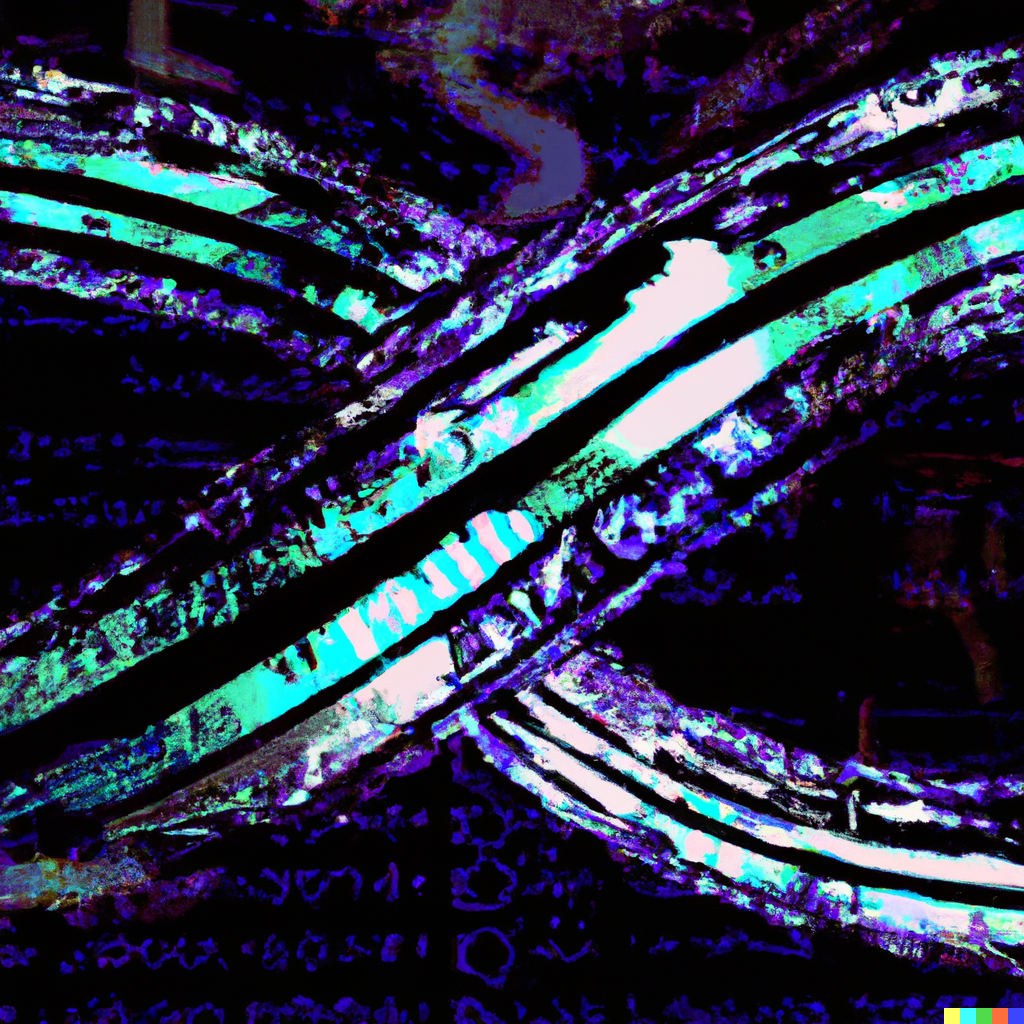
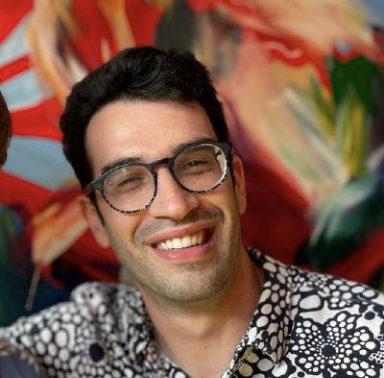
🔬 Research summary by Bernard Koch, a sociologist of science who studies organizational issues in scientific fields and their epistemic and ethical repercussions. He is currently a Postdoctoral Fellow at Northwestern Kellogg School of Management and will be an Assistant Professor at the University of Chicago in 2024.
[Original paper by Bernard Koch, Emily Denton, Alex Hanna, Jacob Gates Foster]
Overview: In AI research, benchmark datasets coordinate researchers around shared problems and measure progress toward shared goals. This paper explores the dynamics of benchmark dataset usage across 43,140 AI research papers published between 2015 and 2020. We find that AI research communities are increasingly concentrated on fewer and fewer datasets and that these datasets have been introduced by researchers situated within a small number of elite institutions. Concentration on research datasets and institutions has implications for the trajectory of the field and the safe deployment of AI algorithms.
Introduction
We’ve known about gender biases in NLP datasets since 2016, yet the same issues still surfaced in generative, multi-modal models like DALL-E and Midjourney. In computer vision, there have been multiple incidents of Black men wrongfully arrested due to facial recognition technology since 2019. To understand why these biases persist in deployed technologies, it’s helpful to think about how they can originate in AI research datasets.
Datasets form the backbone of AI research. They served as training resources for AI models and “benchmarking” tools to evaluate collective progress on a problem. When a research community adopts a dataset as a standard benchmark for testing their algorithms (e.g., ImageNet), they implicitly endorse that dataset as representative of the type of data algorithms should expect to find in the real world. This institutionalization signals to industry adopters that models can be expected to perform similarly to how they do on benchmark datasets.
Theoretically, it’s important that research communities have enough datasets to collectively capture the breadth of real-world data for both scientific and ethical reasons. Scientifically, using too few datasets could lead researchers to “overfit” the models they design to perform well on non-representative data. Ethically, overfitting datasets can create representation biases that lead to unexpected behavior and social harm when models are deployed. To assess these risks, this paper uses population-scale data to quantify the diversity of benchmark datasets used in AI research, across 137 task communities and over time.
Methods and Findings
Measuring Dataset Diversity in AI Research Communities
To quantify dataset diversity in machine learning research, we identified the ~4,000 most widely-cited datasets in the field and the ~40,000 papers that cited those datasets as of June 2021 from a benchmarking repository called PapersWithCode. We separated these papers into 137 research communities, each focused on a specific task. We then measured the diversity of datasets used in research papers using the Gini Index over time (Figure 1).
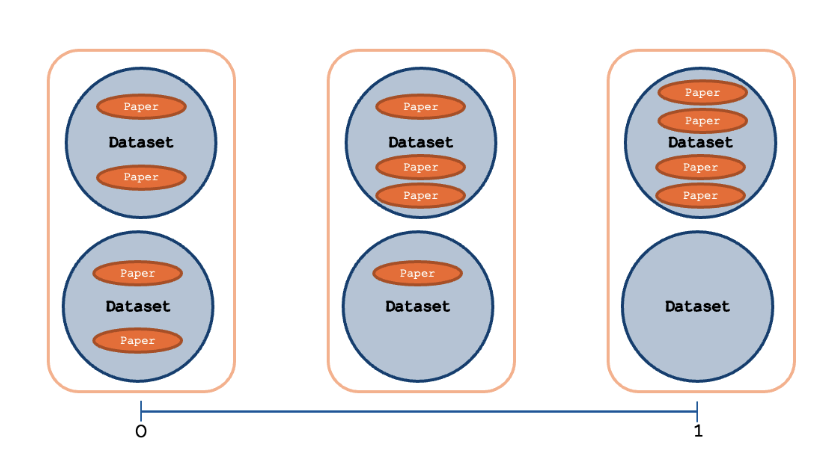
Figure 1: Measuring inequality in datasets usage across papers with the Gini index. To measure the diversity of datasets in a task community, we looked at how evenly dataset-using papers leveraged the full set of datasets available to them using the Gini index. Gini is a scalar metric ranging between 0 and 1. A Gini of 0 means that research papers use all available datasets equally. If the Gini index is 1, every paper in the research community is using the same dataset.
The Diversity of Research Datasets Is Decreasing Over Time
Overall, we found that the diversity of datasets used within AI research communities is surprisingly low, and concentration is increasing over time (Figure 2). We also found that datasets were specifically designed for tasks, but these datasets were often ignored; AI researchers borrow 53% of the time, on average, from other task communities anyway. Interestingly, these trends were much weaker in NLP than in AI as a whole.
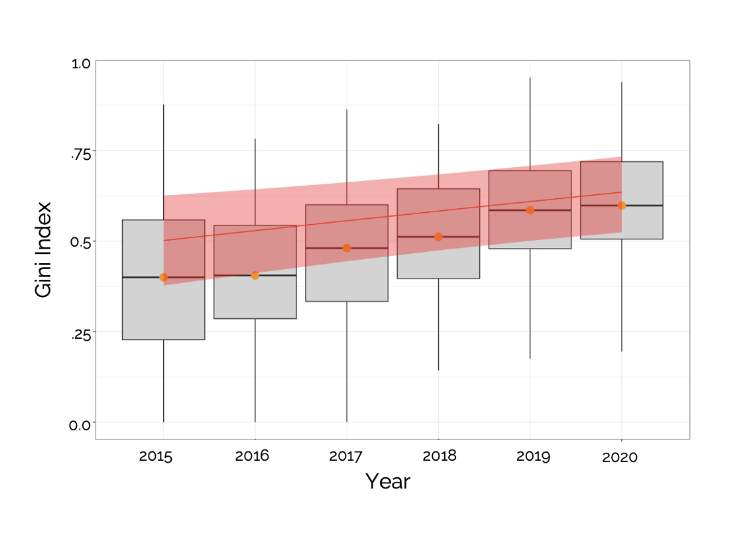
Figure 2: Increasing concentration on fewer datasets across task communities. Each box and whisker plot captures the distribution of Gini indices across tasks in that year. The orange dot is the median Gini. The red ribbon shows that the trend is robust to possible confounders, like the growth of AI research overall.
Datasets in AI Research Are Created by A Handful of Elite Institutions
Lastly, we found that widely-used datasets are introduced by only a handful of elite institutions. In fact, over 50% of dataset usage in PWC as of June 2021 can be attributed to datasets created at just twelve elite institutions (Figure 3). This concentration on elite institutions, as measured through Gini, has increased to over 0.80 in recent years.
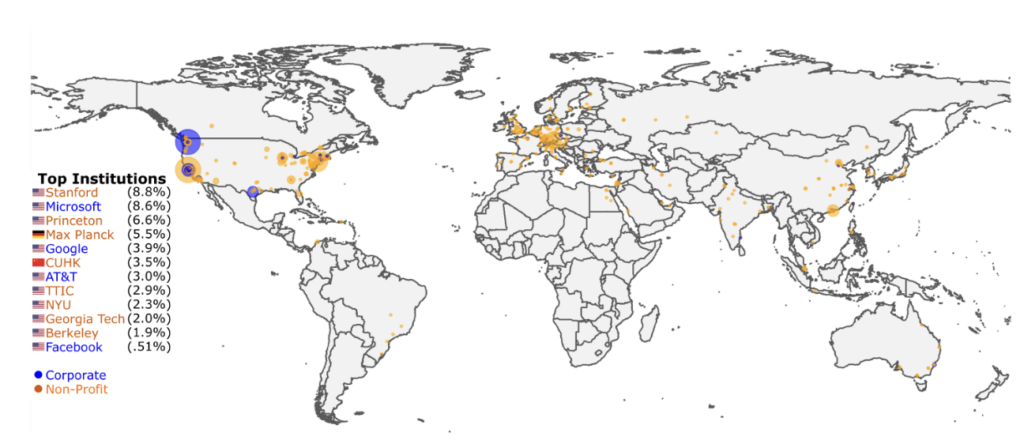
Figure 3: Map of dataset usage per institution as of June 2021. The dot size indicates the number of usages. Blue dots indicate for-profit institutions, and orange dots indicate not-for-profit. Institutions accounting for 50%+ of usage labeled.
Discussion
Dataset Borrowing and Concentration Poses Epistemic and Ethical Risks
A certain degree of research focuses on a single benchmark dataset is both necessary
and healthy for that dataset to be a meaningful measure of scientific progress on a task. Curating large-scale datasets can also be costly and require unique or privileged data (e.g., anonymized medical records, self-driving car logs) accessible to only a few elite academic and corporate institutions. Nevertheless, our findings of widespread concentration on fewer and fewer datasets pose a risk that task communities are “overfitting” benchmarks, leading them to believe they are making better (or worse) progress on problems than they think.
Heavy concentration and borrowing of datasets also pose privacy and representation bias risks. Consider the facial recognition community, where eight datasets accounted for 72.3% of dataset usage as of 2021. Two of the most widely used facial recognition datasets (14.6% of usages), Megaface and MS-Celeb-1M, were retracted because they contained people’s faces without consent. These faces have been used in commercial and government surveillance technologies across the US and China. Because the retractions were poorly publicized, researchers continued to use these datasets long after their retraction. Representation issues in facial recognition datasets have also had real consequences (e.g., wrongful arrests due to facial recognition algorithms). With heavy borrowing across tasks, these issues can potentially propagate to other communities like Image Generation. Our study found that more than 50% of Image Generation papers borrow datasets from the Facial and Object Recognition communities.
Elite Institutions Have Potential to Shape the Agenda of the Field Through Datasets
Insofar as benchmarks shape the types of questions that get asked and the algorithms that get produced, low dataset diversity offers a mechanism through which a small number of elite corporate, government, and academic institutions shape the research agenda and values of the field (Figure 3). Half of the eight facial recognition datasets (33.69% of total usage) were exclusively funded by corporations, the US military, or the Chinese government. There is nothing a priori invalid about powerful institutions being interested in datasets or research agendas that benefit them. However, issues arise when corporate and government institutions have objectives that conflict with other stakeholders’ values (e.g., surveillance versus privacy).
Between the Lines
This paper demonstrates increasing concentration around a core set of datasets and institutions in AI research. I want to emphasize that the epistemic and ethical risks discussed above are potential implications of low dataset diversity, not empirical findings. There has been some interesting work starting to explore these implications, but there is definitely space for further research. From a policy perspective, the paper suggests the need for greater financial and social investment in dataset creation. This would promote diversity in both datasets and dataset-creating voices.
It would be interesting to look at how dataset diversity has changed in the two years since publication. Now that the ability to scale models is hitting hardware limits, there has been a renewed interest in improving AI performance through data quality (“data-centric AI”). At the same time, architectural innovations that improve training efficiency (see the LLAMA paper) have democratized model creation, at least in the short run. I am optimistic that cleaner data and more voices in the field can also build safer, more ethical datasets.
Lastly, a shameless plug: if you found this interesting, please watch for a long-form piece I’m writing about the historical relationship between benchmarking and deep learning. I should have a pre-print up in the next month or two!