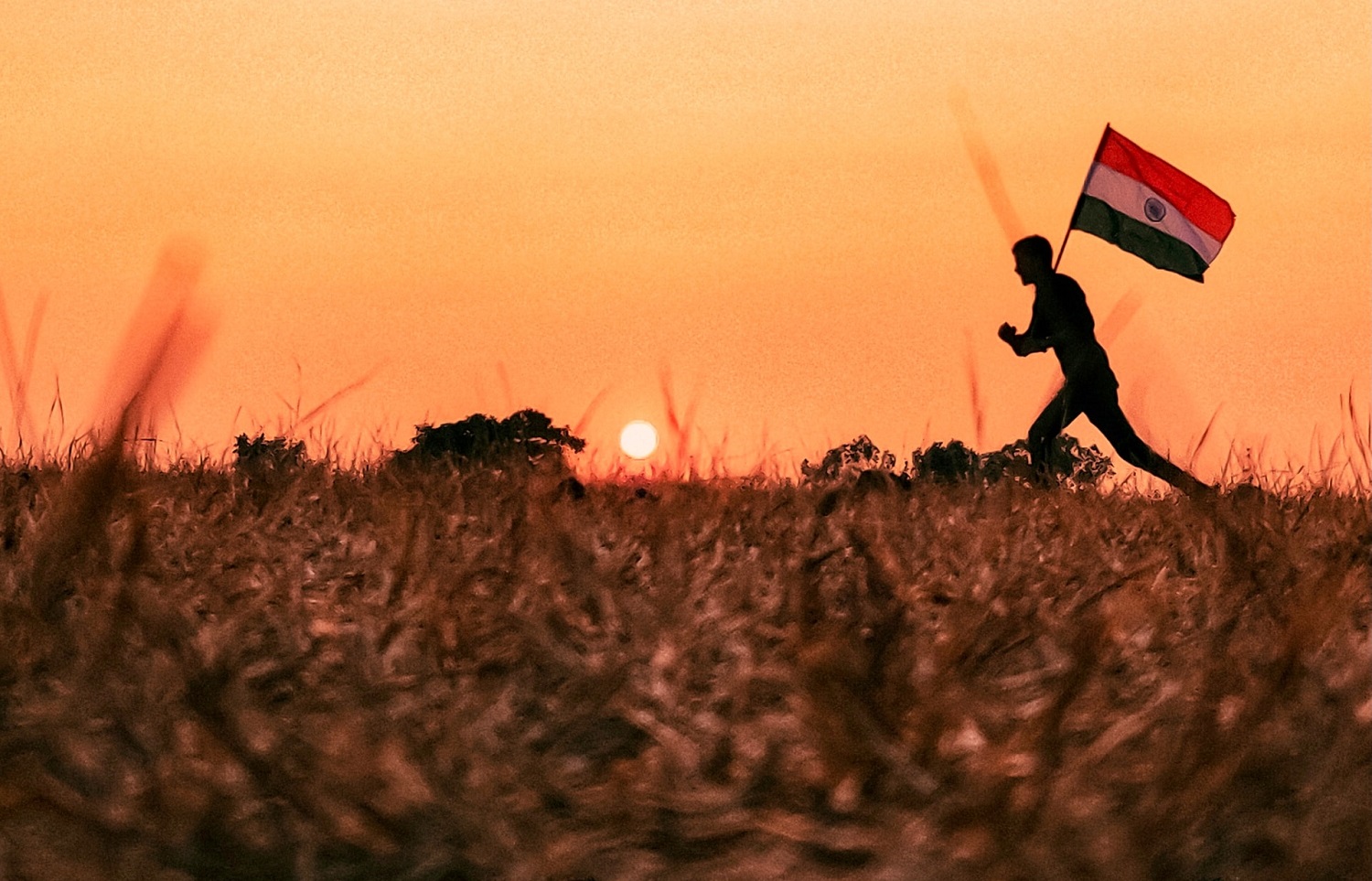
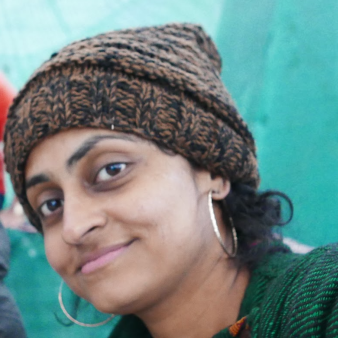
đŹ Research summary contributed by Nithya Sambasivan (@autopoietic), Staff Researcher at PAIR, the lead for the HCI-AI group at Google Research India (Bangalore), and the lead author of the original paper being summarized.
[Link to original paper + authors at the bottom]
Overview: The authors argue that several assumptions of algorithmic fairness are challenged in India. The distance between models and dis-empowered communities is large, and a myopic focus on localising fair model outputs alone can backfire in India. They point to 3 themes that require us to re-examine ML fairness: data & model distortions, double standards & distance by ML makers, and unquestioning AI aspiration.
Algorithmic fairness is West-centric, evidenced in its choice of sub-groups like race and gender, or civil laws in fairness optimisations. However, algorithmic fairness is becoming a universal ethical framework for AI for countries of the Global South. Sambasivan et al. argue that without engaging with the conditions, values, politics, and histories of the non-West, AI fairness can be a tokenism, at bestâpernicious, at worst. As algorithmic fairness emerges as the ethical compass of AI systems, the field needs to examine its own defaults, biases, and blindspots.
In this paper, Sambasivan et al. examine algorithmic power and present a new, holistic framework for algorithmic fairness in India, the worldâs largest democracy. Their method involved semi-structured interviews with India-focused scholars and activists from law to LGBTQ rights to disability rights and a systematic review of algorithmic deployments and policies in India, all using feminist, decolonial, and anti-caste lenses. The authors argue that several assumptions of algorithmic fairness are challenged in India. The distance between models and dis-empowered communities is large, and a myopic focus on localising fair model outputs alone can backfire in India. They point to three themes that require us to re-examine ML fairness:
1) Data and model distortions: Datasets may not faithfully correspond to people and phenomena in India due to socio-economic factors. Models are over-fitted for digitally-rich, middle-class men. Caste, tribe, and religion present new bias vectors. Social justice mechanisms like reservations present new fairness conditions.
2) Double standards and distance by ML makers: Indian users are perceived as âbottom billionâ data subjects, Petri dishes for intrusive models, and given poor recourseâeffectively limiting their agency. While Indians are part of the AI workforce, a majority work in services and the minority engineers often come from privileged class and caste backgrounds, limiting re-mediation of distances.Â
3) Unquestioning AI aspiration: AI is aspirational and readily adopted in high-stakes domains, often too early in India. Lack of an ecosystem of tools, policies, and stakeholders to interrogate high-stakes AI limits meaningful fairness in India.
Call to action
The authors propose an AI fairness research agenda in India along three critical and contingent pathways, calling for going beyond model fairness.
Re-contextualising data and models:Â
Due to the data and model distortions in India, we must be careful with data until they are trustworthy and combine datasets with an understanding of context. The vibrant human infrastructures point to new ways of looking at data as dialogue. Categories, ontologies, and behaviours are context-specific and need to be questioned. The axes of discrimination in India listed could be a starting point to detect and mitigate unfairness issues in models. Fairness criteria should be adopted to social justice mechanisms appropriate to the context.
Empowering communities
Marginalised communities need to be empowered in identifying problems, specifying fairness expectations, and designing systems to avoid top-down fairness. Indiaâs heterogeneity means that Fair-ML researchersâ commitment should go beyond model outputs, to creating accessible systems. Like the fatal Union Carbide gas leak in 1984, unequal standards, inadequate safeguards, and dubious applications of AI in the non-West can lead to catastrophic effects. Fair-ML researchers should understand the systems into which they are embedding, engage with Indian realities, and whether the recourse is meaningful.
Enabling a Fair-ML ecosystem
For Fair-ML research to be impactful and sustainable, it is crucial for researchers to enable a critically conscious Fair-ML ecosystem through solidarity with various stakeholders through partnerships, policy makers, and journalists.
Context matters. We must take care to not copy-paste the western-normative ML fairness everywhere. This paperâs considerations are certainly not limited to India; likewise, they call for inclusively evolving global approaches to Fair-ML.
Original paper by Nithya Sambasivan, Erin Arnesen, Ben Hutchinson, Tulsee Doshi, Vinodkumar Prabhakaran: https://arxiv.org/pdf/2101.09995.pdf