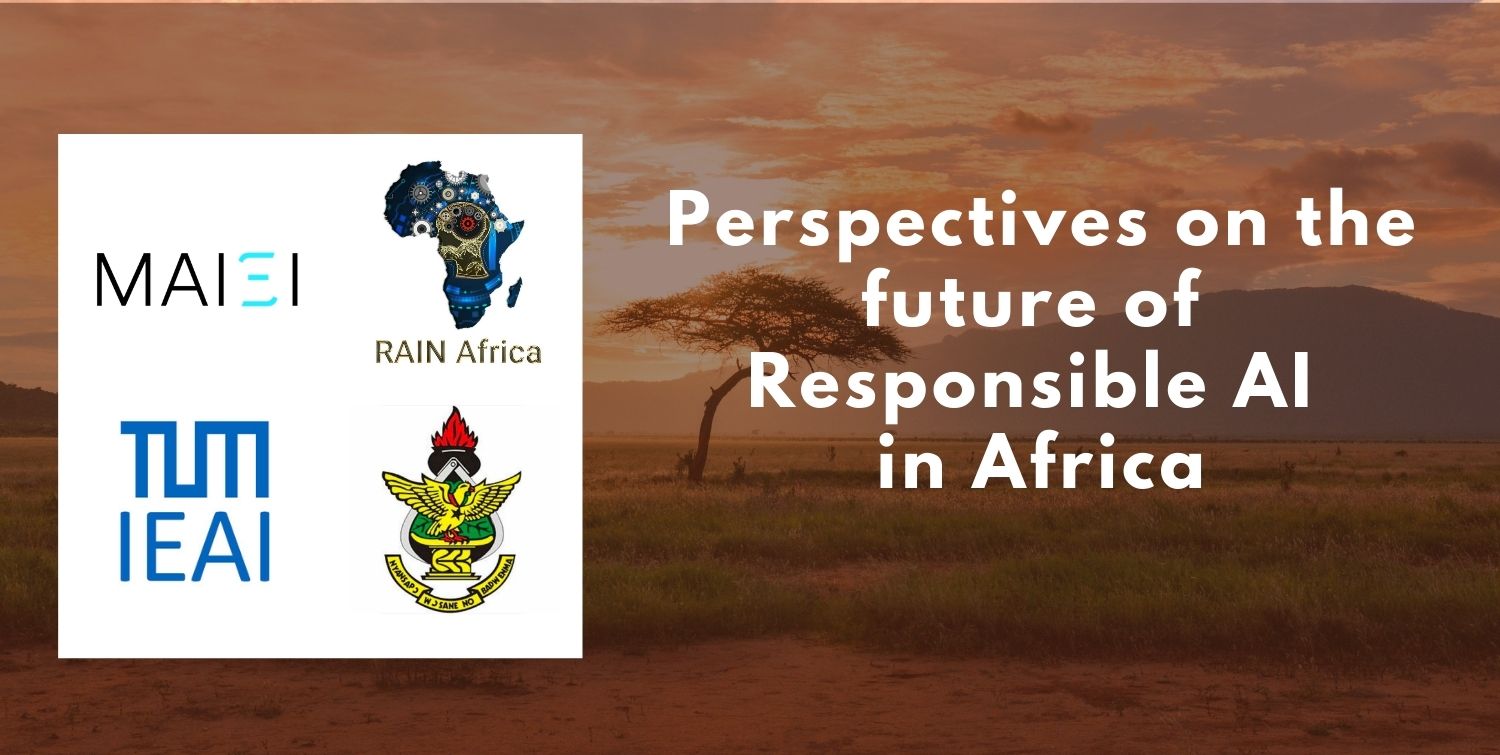
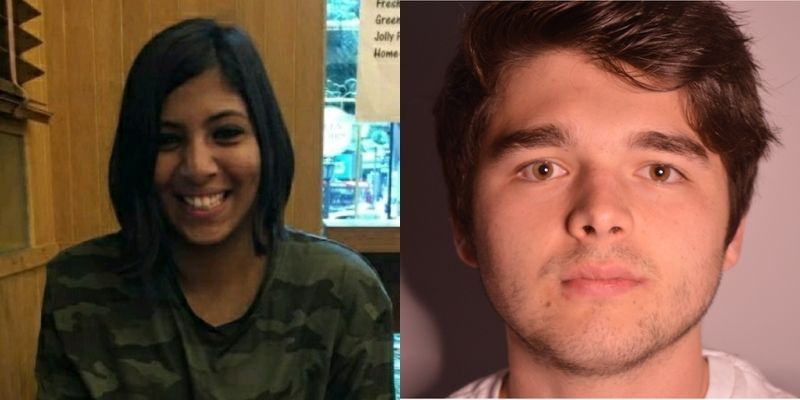
Summary contributed by our researchers Falaah Arif Khan and Connor Wright
Overview: To close out the year, MAIEI teamed up with RAIN Africa to host the âFuture of Responsible AI in Africaâ Workshop, which saw participation from people across law, policy, ethics and computer science. Key insights from the discussions have been summarized in this piece.
Incentives â economic vs social
From the get-go participants called out the need to shift from a solely economic mindset around AI, towards one that emphasized social impact. With this in mind, we touched upon how AI ethics needs to move beyond being a PR exercise for businesses and instead must command serious commitment on their behalf. For example, public declarations on social media by companies to affirm their noble and virtuous plans for the development of AI for populations who donât have access to a computer nor the cultural or linguistic context to understand such declarations, betray themselves as empty and ceremonious. The way forward must be to expand beyond the traditional triple bottom line of business (deepening social, environmental and financial considerations) and to generate the right incentives for the development of AI, focusing more on social impact than on profit margins.
Value systems and Capacity building
We quickly realized that most of our discussion was coalescing around a complete overhaul of the human value system. The idea that we need to rethink corporate responsibility and the impact of technology led us to question whether what needed to be fine-tuned was the human value system itself. To ensure the participation of the African public in such an endeavour, the discussion underscored the need for governments to build capacity and cultivate adequate resources within communities to better equip their citizens in understanding and using the technology available to them. Technical education also needs to include sections on AI ethics. Participants also pointed out that capacity building and data democratization would need investments, for which international relationships with African communities need to be cultivated.
Local contexts and Africa-specific solutions
However, within such considerations, we cannot group all African nations into the same contexts. Each community (whether a nation or region) faces different local challenges, which require different approaches, keeping in mind local cultural sensitivities. For example, Nigeria possesses over 200+ ethnicities, all with different linguistics and practices to take into account. Hence, a locally sourced and multidisciplinary research effort needs to be realized. In this sense, participants pointed out that popular scholarship (such as the Brookings article, which was background reading for the workshop) doesnât adequately recognize the regional and social aspects of technological challenges, which are acutely sensitive throughout Africa.
For example, the issue of a lack of different African-specific datasets was brought up in the discussion. Tackling a lack of African-specific data sets for systems such as Facial Recognition Technology (FRT) is a pressing problem since African communities are not adequately represented in Western-centric data sets that dominate current AI scholarship. A solution to this could take the form of unique data sets for each community.
Another dimension to this problem is the novel technical problems that arise due to a lack of Africa-specific datasets. Results that are deemed to be âstate of the artâ in AI today have only been tested on Western-centric datasets and do not transfer seamlessly into the African context. For example, most African languages are low-resource, which presents a technical challenge in machine translation of African languages. Voice recognition software also performs remarkably poorly on various native African accents.
Regulation and Policy
In the West, the rapid adoption and development of AI has left attempts at policy and regulation in the dust. Participants pointed out that we have a unique opportunity for the responsible development of AI in Africa â the technology is still in a fledgling state and so policy isnât playing catch-up. From a policy perspective, participants concurred on the fact that we can and must enforce the application of a dataset and a model within the specific context for which it was created.
Democratization and accessibility â AI literacy
We unanimously agreed that AI has the potential to bring about tremendous good for the African continent. A key prerequisite for the successful adoption of new technology is public trust, which requires awareness about the technology. In the context of the labor market in Africa, we discussed how the average citizen has little to no idea about the impact that algorithmic interventions can have on their livelihood. AI literacy needs to be a priority â building public competency by making technical knowledge more accessible. For example, in the case of auditing AI systems, explainability to non-specialist audiences is especially difficult and so we need novel methods and mediums for the dissemination of technical knowledge.
Data ownership
Participants wholeheartedly endorsed the idea that we need African solutions to African problems. With this in mind, the question of ownership is a key consideration. Data is set to become the most important resource and so those from whom data has been collected, need to have access and ownership over the data. Issues of privacy and security of data come to the forefront and this ties back to the idea that AI policy needs to be in place so that when we collect data or use the predictions from a model to make decisions, we do it within a specific context and policy regime.