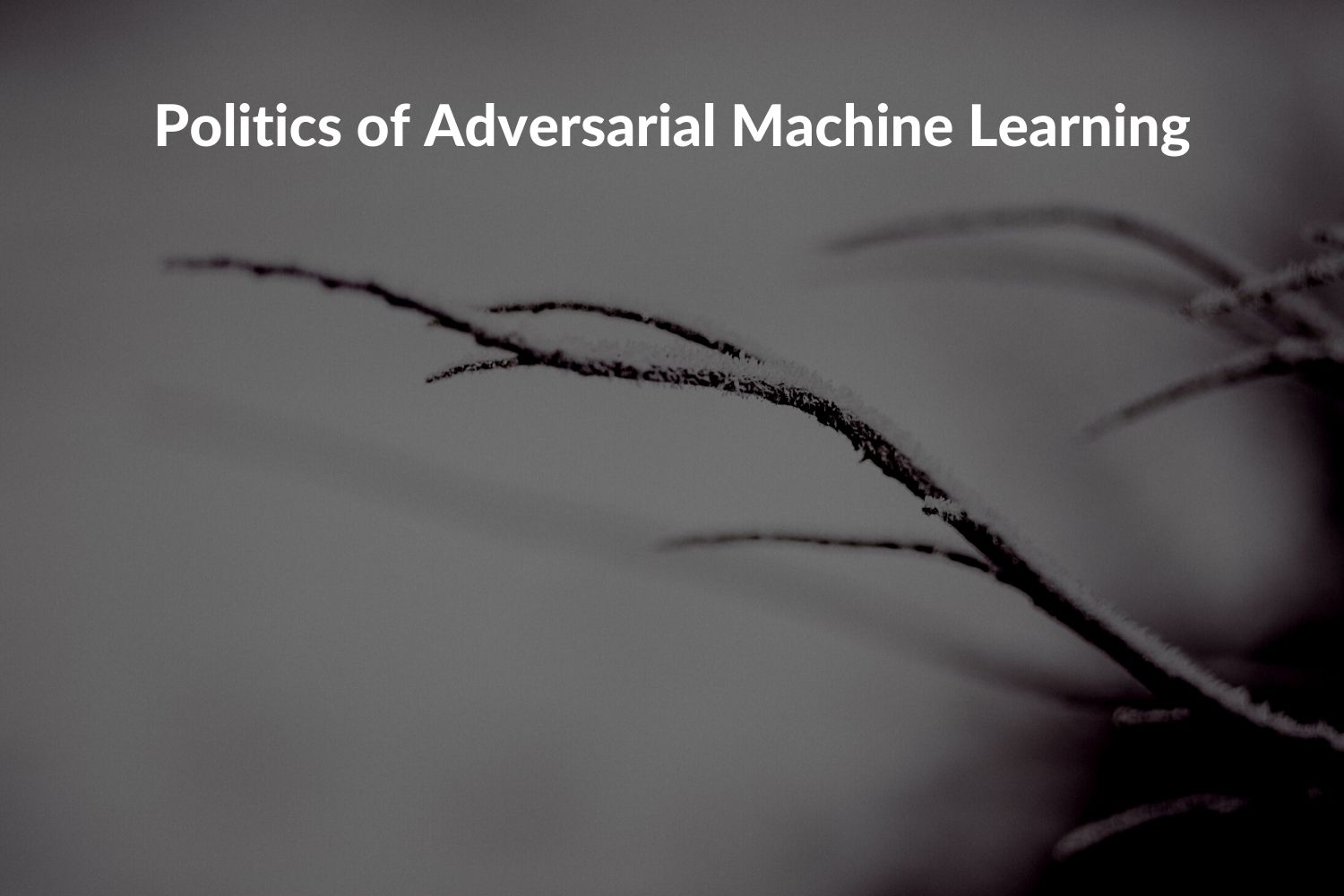
Mini summary (scroll down for full summary):
A very timely paper that in the post-ClearviewAI world brings forth some important issues about how to think about the societal implications of the work done in the ML Security space. (If this is your first encounter with this term, please take a look through our learning community at the Montreal AI Ethics Institute to know more, we believe that this emergent area is of prime importance as ML systems become more widely deployed.) The paper takes the case study of facial recognition technology as model for reasoning about some of the challenges that we encounter when we harden ML systems against adversarial attacks. It provides an insightful reframing of the meaning of attacks against a system, moving away from the typical notions of cybersecurity wherein an attacker is an entity that compromises the confidentiality, integrity and availability of a system for some malicious purpose.
Examples of when that’s not the case include someone trying to learn whether there image was used in a dataset that the system was trained on, determining whether an opaque ML system has bias issues and protecting identities of protestors and other vulnerable populations who are fighting for their civil liberties and human rights in states where they might be persecuted if they are recognized. Drawing on lessons learned from the work done by civil society organizations and others to combat ethical, safety and inclusivity issues from the commercial spyware industry, the authors urge developers and vendors of ML systems to consider human rights by design principles and other recommendations when thinking about hardening their ML systems against adversarial attacks.
Full summary:
All technology has implications for civil liberties and human rights, the paper opens with an example of how low-clearance bridges between New York and Long Island were supposedly created with the intention of disallowing public buses from crossing via the underpasses to discourage the movement of users of public transportation, primarily disadvantaged groups from accessing certain areas.
In the context of adversarial machine learning, taking the case of Facial Recognition Technology (FRT), the authors demonstrate that harm can result on the most vulnerable, harm which is not theoretical and is gaining in scope, but that the analysis also extends beyond just FRT systems.
The notion of legibility borrowing from prior work explains how governments seek to categorize through customs, conventions and other mechanisms information about their subjects centrally. Legibility is enabled for faces through FRT, something that previously was only possible as a human skill. This combined with the scale offered by machine learning makes this a potent tool for authoritarian states to exert control over their populations.
From a cybersecurity perspective, attackers are those that compromise the confidentiality, integrity and availability of a system, yet they are not always malicious, sometimes they may be pro-democracy protestors who are trying to resist identification and arrest by the use of FRT. When we frame the challenges in building robust ML systems, we must also pay attention to the social and political implications as to who is the system being made safe for and at what costs.
Positive attacks against such systems might also be carried out by academics who are trying to learn about and address some of the ethical, safety and inclusivity issues around FRT systems. Other examples such as the hardening of systems against doing membership inference means that researchers can’t determine if an image was included in the dataset, and someone looking to use this as evidence in a court of law is deterred from doing so. Detection perturbation algorithms permit an image to be altered such that faces can’t be recognized in an image, for example, this can be used by a journalist to take a picture of a protest scene without giving away the identities of people. But, defensive measures that disarm such techniques hinder such positive use cases. Defense measures against model inversion attacks don’t allow researchers and civil liberty defenders to peer into black box systems, especially those that might be biased against minorities in cases like credit allocation, parole decision-making, etc.
The world of security is always an arms race whether that is in the physical or cyberspace. It is not that far-fetched to imagine how a surveillance state might deploy FRT to identify protestors who as a defense might start to wear face masks for occlusion. The state could then deploy techniques that bypass this and utilize other scanning and recognition techniques to which the people might respond by wearing adversarial clothing and eyeglasses to throw off the system at which point the state might choose to use other biometric identifiers like iris scanning and gait detection. This constant arms battle, especially when defenses and offenses are constructed without the sense for the societal impacts leads to harm whose burden is mostly borne by those who are the most vulnerable and looking to fight for their rights and liberties.
This is not the first time that technology runs up against civil liberties and human rights, there are lessons to be learned from the commercial spyware industry and how civil society organizations and other groups came together to create “human rights by design” principles that helped to set some ground rules for how to use this technology responsibly. Researchers and practitioners in the field of ML Security can borrow from these principles. We’ve got a learning community at the Montreal AI Ethics Institute that is centered around these ideas that brings together academics and others from around the world to blend the social sciences with the technical sciences.
Recommendations on countering some of the harms centre around holding the vendors of these systems to the business standards set by the UN, implementing transparency measures during the development process, utilizing human rights by design approaches, logging ML system uses along with possible nature and forms of attacks and pushing the development team to think about both the positive and negative use cases for the systems such that informed trade-offs can be made when hardening these systems to external attacks.
Original piece by Kendra Albert, Jonathon Penney, Bruce Schneier and Ram Shankar Siva Kumar: https://arxiv.org/abs/2002.05648