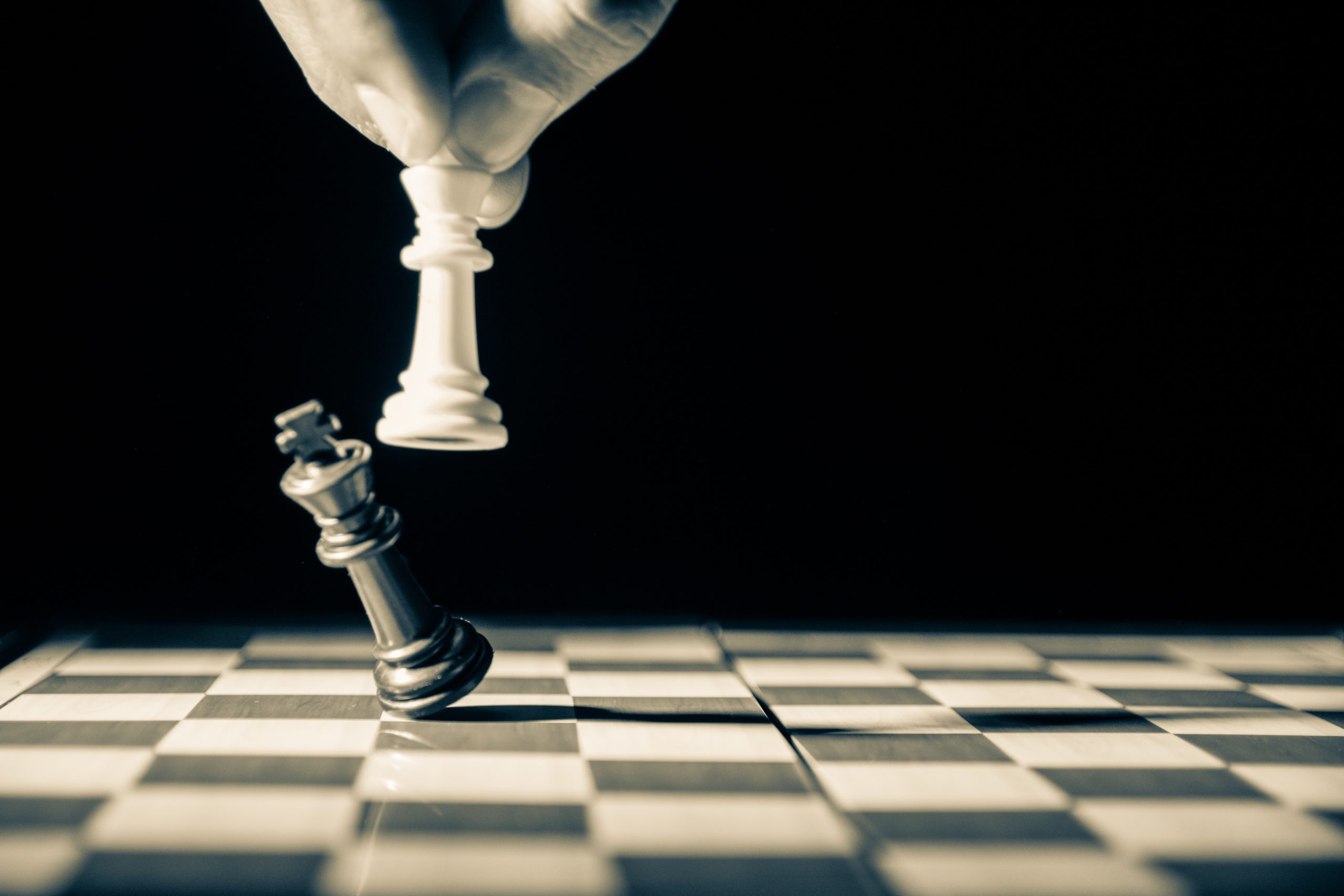
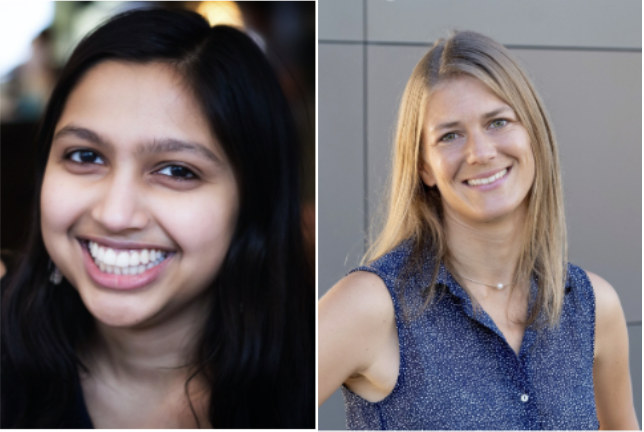
🔬 Research Summary by Meena Jagadeesan and Celestine Mendler-Dünner.
Meena Jagadeesan is a PhD student in Computer Science at UC Berkeley. Her interests lie in the theoretical foundations of machine learning and algorithmic decision-making, including intersections with economics.
Celestine Mendler-DĂĽnner is a research group lead at the Max Planck Institute for Intelligent Systems in TĂĽbingen, Germany. Her research focuses on machine learning algorithms and their interplay with society. She spent two years as a postdoc at UC Berkeley and holds a PhD in Computer Science from ETH Zurich.
[Original paper by Moritz Hardt, Meena Jagadeesan, Celestine Mendler-DĂĽnner]
Overview: We propose performative power as a measure to assess the power of a firm within a digital economy. In contrast to classical measures of market power, performative power does not rely on a specific market definition; it instead uses observational data to directly measure the effect an algorithmic system can have on the behavior of its participants.Â
Introduction
This work centrally deals with the question of the power of an algorithmic system within a digital economy. How does its power surface, and how can we quantify it?
In the Oxford Lexico, “power” is defined as “the ability to direct or influence the behavior of others or the course of events.” To instantiate this definition more concretely within digital economies, we focus on algorithmic predictions as the centerpiece of the service of many businesses and as a means to direct consumer behavior. Algorithmic predictions filter the products that appear in our personalized search results, inform the allocation of police in our neighborhood, pre-screen our job applications, and decide whether banks approve or reject our next loan. Such predictions are pervasive; they steer markets, drive consumption patterns, and impact many aspects of our lives.
The key principle behind our proposal is the following: the extent to which changes in the firm’s predictions impact participants’ behavior in a digital economy reveals a salient power relationship between the firm making the predictions and the participants. In our recent work, we translate this intuition into a formal definition of performative power, stress-test its qualitative behavior in different market models, and sketch the potential role we envision this new measure could play in the context of antitrust enforcement.
As a running example, consider a firm offering a traffic prediction app that recommends routes to drivers. An investigator might be interested in understanding how much power such a firm has over the realized traffic in a particular city. Now a natural question to ask is: what would happen if the app changed its route recommendations? Would the traffic patterns in the city change? Performative power constitutes the answer to this causal question that reveals valuable information about the economic power of the firm.
Key Insights
Performativity in predictive systems
The phenomenon that mathematical models can influence and shape the world they seek to describe and understand is widely acknowledged in modern economics and finance. In the 1960s, Donald MacKenzie discussed in his book “An engine not a camera” how option pricing models designed to predict financial markets fundamentally alter economic realities in that they inform investment decisions and impact the way markets are studied. Such predictive models are said to be performative: they shape the aspect of reality that they seek to describe.
In the context of predictive systems, performativity has been documented in various contexts. Algorithmic recommendation systems impact user behavior by steering what users watch and like. Automated credit scoring algorithms impact consumer behavior by incentivizing users to present themselves strategically in the hope of receiving a more favorable predictive outcome. Traffic prediction services directly influence which route divers take, and the outcome of credit scoring algorithms directly impacts the financial situation of the applicant. These performative phenomena arise because prediction-driven services play an ever more important role in various aspects of our lives. The pervasive evidence of these performative phenomena motivated our proposal of performative power.
Towards a formal definition
Performative power estimates the impact a prediction algorithm deployed by a firm has, not the behavior of its participants. In the following, we attempt to make this proposal more formal without going into all the technical details of the paper. Performative power is a causal statistical measure that quantifies the average effect of a platform’s prediction on a population of interest. This population specifies the market participants we care about. Each participant is associated with a set of attributes, and performative power measures the extent to which the platform can change these attributes by deploying changes to the predictive model. It directly compares the current market situation to the counterfactual situation surfacing from the change. As such, it is a data-driven statistic. Similar to classical causal effect measures, performative power can be assessed through both an experimental or observational causal design.
Connections to economic power
The core contribution of this work is to draw a formal connection between performativity in predictive systems and the economic concept of power. Predictive systems play a central role in many businesses, and performative power assesses the effect they can have on the behavior of their participants. While our measure is purely observational, it is sensitive to salient aspects of the market – the effect of a firm’s predictions is more pronounced when the firm has a strong market position, a large user base, and high consumer retention; on the other hand, the effects are less pronounced when competition is present, and close substitutes to the firm’s predictive service are available. This aligns with the qualitative behavior we expect from any measure of economic power. By establishing this link, performative power turns the original question of assessing the power of a firm in a digital economy into a causal inference problem that is more familiar to computer scientists.
Consequences of high performative power
A natural question to ask is: what does it mean if a firm is found to have high performative power?
It means that the firm can steer a population. In analogy with a price-maker in economics, the firm can shape the market. This ability offers the firm a lever they can exploit when optimizing their predictive models in light of their chosen profit metric. Concretely, high performative power means that the firm can steer towards behavior that is easier to predict or monetize. For the reader familiar with the typical risk minimization objective in supervised learning, this implies that to achieve low risk, the firm can not merely extract existing patterns but change the distribution over data. This constitutes a widely unexplored dimension of prediction.
From the perspective of user welfare, high performative power constitutes a reason for concern as it implies a lot of control for the platform over its participants. But ultimately, whether this power is used for good or bad requires further investigations, and it largely depends on the firm’s optimization strategies, its incentives, and how well they are aligned with user interests.
Between the lines
Performative power is proposed in the context of digital economies. It is widely acknowledged that digital economies are complex and differ significantly from classical markets. As a consequence, classical tools from economic theory struggle to provide insight into market power in digital economies necessary for effective antitrust enforcement. In part, because many of these markets are multi-sided, the meaning of products, market participants, and prices is completely altered, and participant behavior is highly susceptible to behavioral weaknesses. To tackle these challenges, there is a need for new investigative tools and economic concepts.
In response to this call, performative power focuses on where the rubber meets the road: the predictive model of the platform and its causal powers. Thereby it side-steps the challenge of specifying the complex mechanisms governing the market but instead focuses on a directly observational statistic. We envision that performative power can provide a valuable new perspective and serve as a complementary tool to existing approaches for measuring power in digital economies. It could help flag the potential for user harm in digital marketplaces and identify situations that merit further investigation.