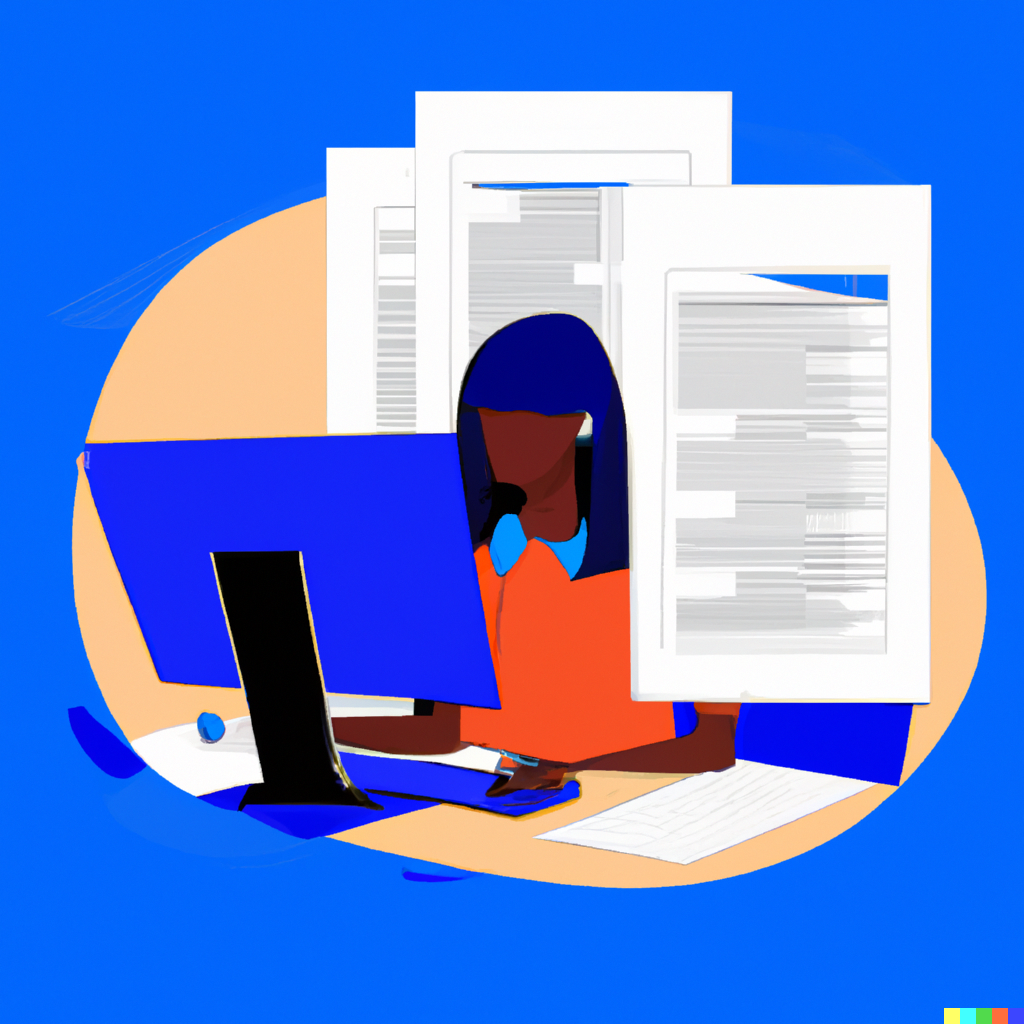
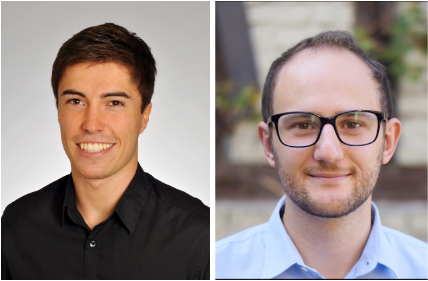
🔬 Research Summary by Philipp Spitzer and Joshua Holstein
Philipp is a second-year PhD student at the Karlsruhe Institute of Technology, where he is working on topics related to human-AI interaction and the use of machine learning to capture and distribute expert knowledge.
Joshua is a second-year PhD student at the Karlsruhe Institute of Technology, focusing on the intricacies of data from complex systems, emphasizing the collaboration between experts and AI-driven solutions.
[Original paper by Philipp Spitzer, Joshua Holstein, Michael Vössing, and Niklas Kühl]
Overview: In the realm of human-AI interfaces, quantifying instance difficulty for specific tasks presents methodological complexities. This research investigates prevailing metrics, revealing inconsistencies in evaluating perceived difficulty across human and AI agents. This paper seeks to elucidate these disparities through an empirical approach, advancing the development of more effective and reliable human-AI interaction systems, considering the diverse skills and capabilities of both humans and AI agents.
Introduction
Integrating artificial intelligence (AI) into daily life has magnified the need to accurately determine the difficulty encountered by humans and AI agents in various scenarios. Assessing difficulty is essential to improve human-AI interaction, emphasizing a systematic comparison between human and AI agents. Based on an extensive review, it identifies inconsistencies in prevailing methodologies used to measure perceived difficulty and underscores the need for uniform metrics.
The paper presents an experimental design combining between-subject and within-subject paradigms to address this gap. It uses standard confidence metrics in conjunction with the innovative Pointwise 𝒱-Information (PVI) score to accurately evaluate the apparent difficulty for each entity on specific instances. This approach guarantees equal accessibility to information for both agents, creating the basis for an in-depth primary investigation.
The potential implications of this research are manifold. By discerning the differing perceptions of difficulty between humans and AI agents, this study anticipates the development of enhanced and consistent frameworks for human-AI interaction. These advancements ensure efficient collaboration in an increasingly AI-augmented scientific landscape.
Key Insights
The Pervasiveness of AI
Artificial Intelligence (AI) has become an integral part of our modern world, revolutionizing how we interact with technology. From language learning applications to self-driving cars, AI’s presence is undeniable. Yet, one of the core challenges in this evolving landscape is evaluating the difficult perception of instances for humans and AI agents, a challenge beyond the scope of previous research.
The Need for Accurate Difficulty Assessment
In the realm of human-AI interactions, accurately gauging the difficulty of individual instances is paramount, e.g., classifying a specific image. The implications of misjudging instance difficulty can range from minor inconveniences, like struggling with a language-learning app, to potentially catastrophic consequences, such as a self-driving car navigating through treacherous conditions. To enable effective collaboration and enhance user experiences, it’s essential to precisely evaluate each agent’s perceived difficulty in achieving desired outcomes.
Understanding the Landscape: Key Terms and Metrics
In the world of assessing instance difficulty, several terms play a central role. Performance, for example, quantifies the overall accuracy of completing a task over multiple instances or agents. However, it’s the terms uncertainty and confidence that often add complexity to the equation. While used interchangeably in some contexts, they carry distinct meanings. Uncertainty relates to the distribution of probabilities across potential outcomes, whereas confidence represents the probability of a specific decision being correct.
A crucial distinction lies in the differentiation between objective and perceived difficulty. The former can be quantified by examining the inherent complexity of a given instance, for example, by measuring the number of features it entails. In contrast, perceived difficulty revolves around subjective perception. Human and AI agents perceive this type of difficulty differently. The one for AI is oftentimes based on softmax outputs, while the one for humans is sometimes assessed through average performance.
Unifying Metrics and Methods
To bridge the gap between how humans and AI perceive instance difficulty, the paper presents an experimental design to study the difference in difficulty perception for single instances. This approach aims to create uniform assessments of perceived difficulty for humans and AI agents by employing a mixed-effects model that combines between-subject and within-subject designs. It employs two well-established metrics—confidence and the Pointwise 𝒱-Information (PVI) score, with the latter offering a unique perspective by considering label distribution. Confidence for AI agents is measured using Monte-Carlo Dropout, while humans express their confidence through Likert scales.
In addition, the study investigates the impact of granting access to label distribution information before task execution. AI agents typically have this information due to their training, whereas humans often lack it. Comparing two conditions—one where humans have access to label distribution information and one where they do not—sheds light on how this access influences humans’ assessments of perceived difficulty. This exploration is guided by hypotheses that suggest the potential impact of information access on assessments and highlight instances where human and AI agents might differ in their perceived difficulty assessments.
In Conclusion: Bridging Perceived Difficulty
In a world increasingly shaped by AI, assessing instance difficulty for humans and AI agents on a specific task remains a fundamental challenge. This paper navigates the complexities of this challenge, offering insights and an innovative experimental design. The implications of this research reach far and wide, from the practical application of enhancing AI-assisted systems to the research horizon, which beckons further exploration of perceived difficulty assessment. As AI continues integrating into our lives, this research charts a path toward more efficient, user-centric, and harmonious human-AI interactions. This journey extends beyond the confines of any single paper.
Between the lines
This research holds paramount importance in the field of human-AI interaction as it addresses a fundamental challenge: accurately assessing instance difficulty for both humans and AI agents. In an era where AI is increasingly integrated into various aspects of life, from autonomous driving to language learning, the consequences of misjudging instance difficulty can range from inconvenience to safety hazards. By delving into this challenge, the study provides a foundational understanding of bridging the perceived difficulty gap between humans and AI agents.
The implications of this research are highly valuable for both practice and research. Practically, it offers insights into designing more effective and reliable human-AI interaction systems. Accommodating individuals’ diverse cognitive styles and skills is critical for creating seamless and enjoyable AI-assisted experiences. In the research realm, this work opens up avenues for exploring nuanced approaches to assessing instance difficulty, considering individual differences, and refining metrics. Future research can delve deeper into understanding how human factors influence perceived difficulty and can explore innovative ways to enhance human-AI collaboration.