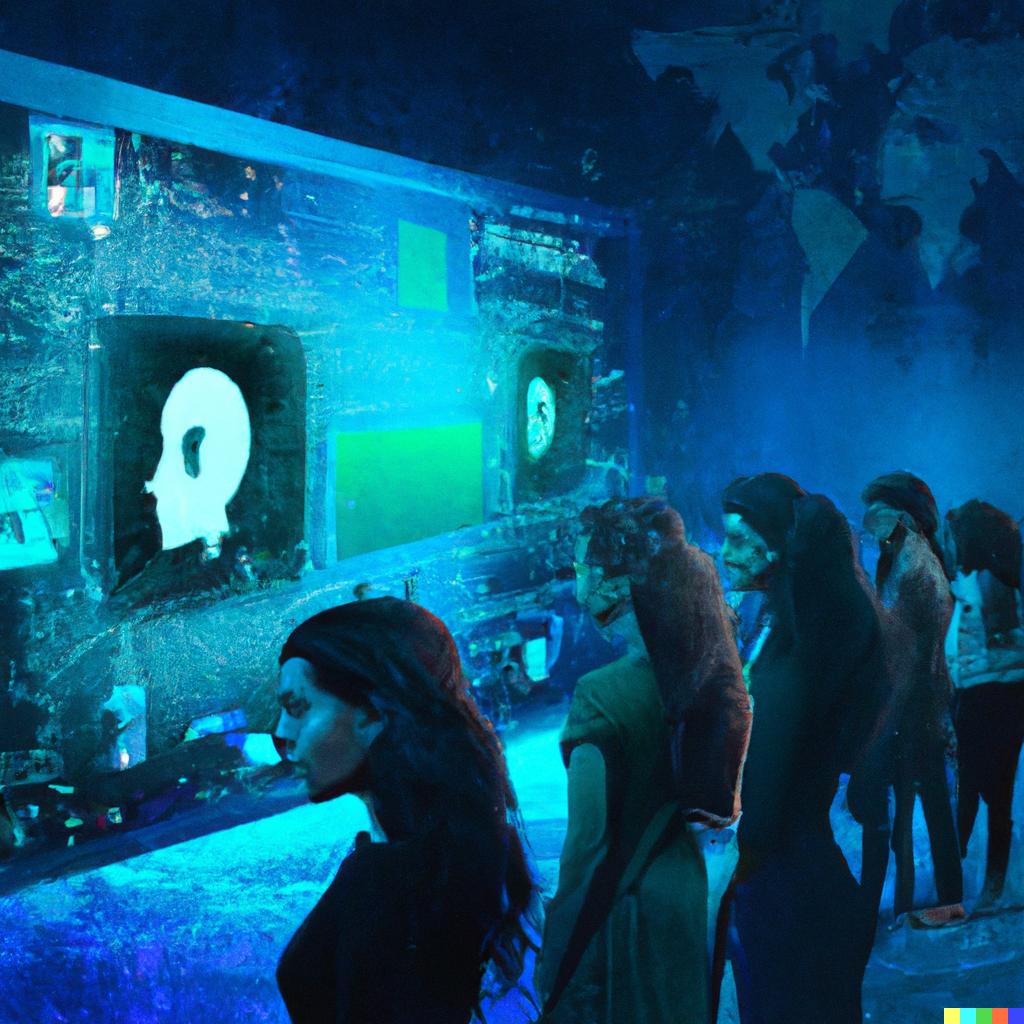
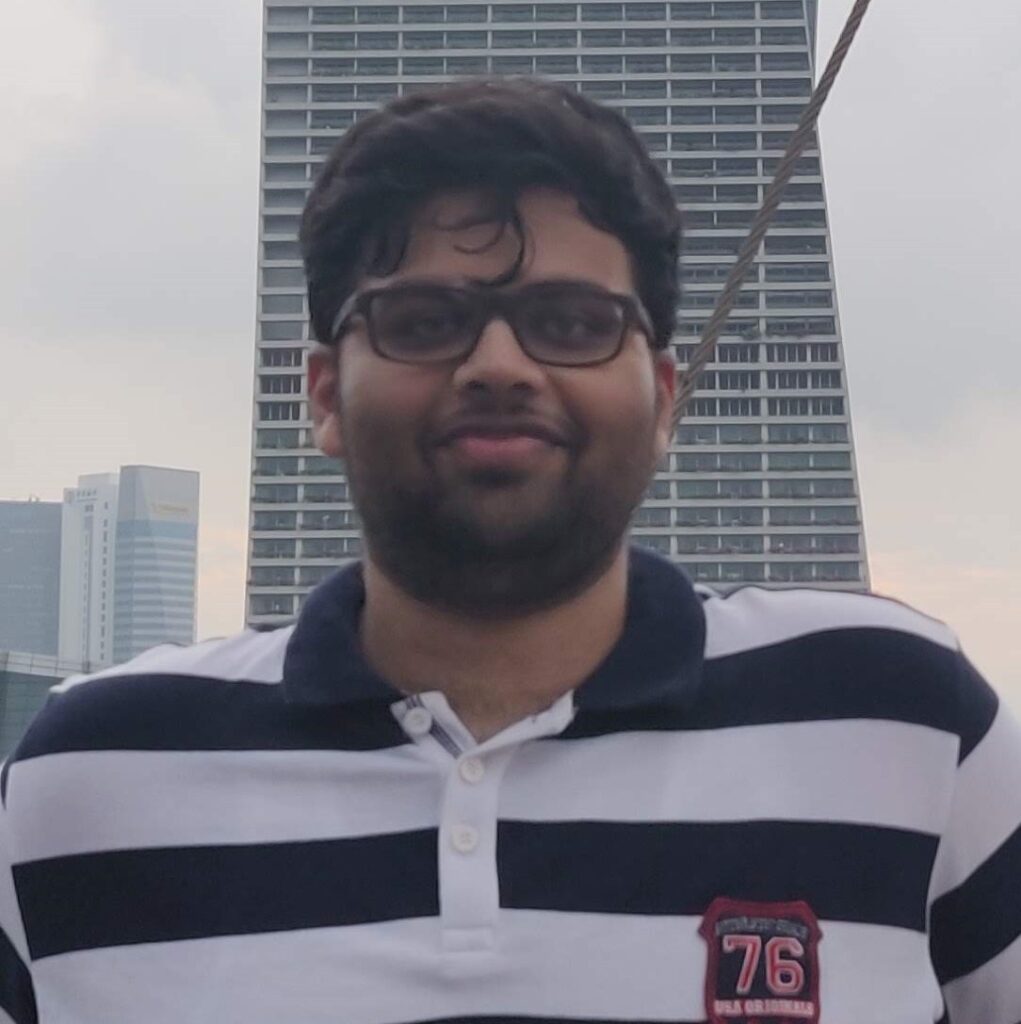
🔬 Research Summary by Prakhar Ganesh, incoming Ph.D. student at the University of Montreal and Mila; interested in studying the learning dynamics of neural networks at the intersection of fairness, robustness, privacy, and security.
[Original paper by Prakhar Ganesh, Hongyan Chang, Martin Strobel, and Reza Shokri]
Overview: Statistical measures for group fairness in machine learning reveal significant variance among different training instances, i.e., simply retraining the model under a different random seed can lead to dramatic changes in the experienced bias. In our research, we delve into the impact of randomness in model training on this variability and emphasize the critical role of data order during training and its influence on group fairness. Our work was recognized with the prestigious Best Paper Award at FAccT 2023.
Introduction
Fairness research in ML has grown a lot, but how reliable are the trends uncovered by these papers? Did you think that bigger models always mean more bias? Not for toxic text classification (Baldini et al., 2022), as the variance across random seeds is too large to determine any reasonable trends. Do existing bias mitigation methods work well with LLM fine-tuning? Actually, a lot of it depends on the randomness of your pre-trained model (Sellam et al., 2022). But what if we start with the same pre-trained model? Still not reliable! Recent work in bias mitigation for clinical texts shows the improvement isn’t statistically significant across random seeds (Amir et al., 2021).
What’s happening here? The problem is model multiplicity, a phenomenon in which – “there [can] often exist multiple models for a given prediction task with equal accuracy that differs in their individual-level predictions or aggregate properties” (Black et al., 2022). Neural networks are heavily over-parameterized models, which can give rise to this multiplicity. Several researchers have highlighted the high variance in model fairness in the last few years, despite similar accuracy scores. And one of the most arbitrary sources of this multiplicity is the randomness in model training!
In this work, we perform an empirical investigation into the high fairness variance due to randomness in training and answer the following questions,
- Is there a single dominant source of randomness? Yes, data reshuffling during training.
- Why are fairness measures highly sensitive to randomness? Higher prediction uncertainty of under-represented groups.
- How exactly does data order impact fairness? The most recent gradient updates are the most powerful, irrespective of the preceding training.
- What are the practical implications of our work? We will show how to study multiplicity in fairness without actually training multiple models. We will also show how to create custom data orders to improve model fairness within a single epoch of fine-tuning.
Key Insights
Our work is highly empirical, and thus for the main body of this research summary, I will focus on various insights that we got from our experiments.
The Dominant Source of Randomness: Data Reshuffling
We found that the high fairness variance across multiple runs in neural networks is dominated by randomness due to data reshuffling during training. Reshuffling causes large changes in fairness scores even between consecutive epochs within a single training run, highlighting its immediate impact on group fairness. At the same time, other forms of randomness, like weight initialization, have minimal influence.
Fairness Measures and High Sensitivity to Randomness
We show a higher vulnerability of minorities to changing model behavior, i.e., a higher prediction uncertainty for under-represented groups. In simpler terms, groups with smaller representations in the dataset are more significantly influenced by any change in the model parameters, including changes due to the randomness in data reshuffling, resulting in high fairness variance. This disparate prediction uncertainty between groups is not limited to a single metric and will be reflected in any statistical fairness measure defined on model predictions.
The Impact of Data Order on Fairness
We highlight the immediate impact of the data order on fairness. That is, we found that a model’s fairness score is heavily influenced by the data distribution in the most recent gradient updates seen by the model, irrespective of the preceding training. We will use this insight later to create custom data orders that can efficiently control group-level performances (and thus model fairness) with a minor impact on the overall accuracy.
Applications
Given the immediate impact of data order on model fairness, the fairness score at the end of a training run is mainly influenced by the data order in the last training epoch. Moreover, given the nature of data reshuffling during training, the data order at the end of every epoch across multiple training runs represents the same distribution as the data order across multiple epochs in a single training run. Thus, we propose (and show with a diverse set of experiments) that using fairness variance across epochs in a single training run is a good proxy to study fairness variance across multiple runs, thus reducing the computational requirements by a significant factor.
Based on our insights, we also create custom data orders that can improve model fairness within a single training epoch and perform as well as existing bias mitigation algorithms. We do this by simply moving individuals from under-represented groups to the end of the data order, thus ensuring the model’s data distribution in its most recent gradients resembles a balanced distribution, even though the overall dataset is imbalanced. Interestingly, we show that similar custom data orders can also be created by adversaries (by moving minorities to the start of the data order) to freely control fairness gaps however they like in only a single epoch of training, even under explicit bias mitigation.
Between the lines
Our work explores the nuances of machine learning randomness by decoupling various sources of randomness and studying their impact separately, which has been missing from existing literature on group fairness despite several studies highlighting the impact of randomness on fairness scores. More importantly, our work highlights the power of data order during training, which can compete with far more complicated bias mitigation constraints and optimizations, and we wonder if data order can also be the answer to introducing many other desirable properties that we want in a learning model.
Our work is, however, limited to smaller neural networks. One of the central observations in our work is the immediate impact of data order, which is driven by the volatile nature of each gradient update in our setting. It would be interesting to see whether such behavior persists in a less volatile setting, like with a lower learning rate or bigger batch size, as is usually used to train extremely large models. Moreover, our work is limited to the impact of data order on fairness, which is dependent only on the final discrete predictions made by the model. This is a special case of the model’s internal learning dynamics. We believe future work investigating the impact of data order on the model’s internal behavior can have widespread applications in many other domains.