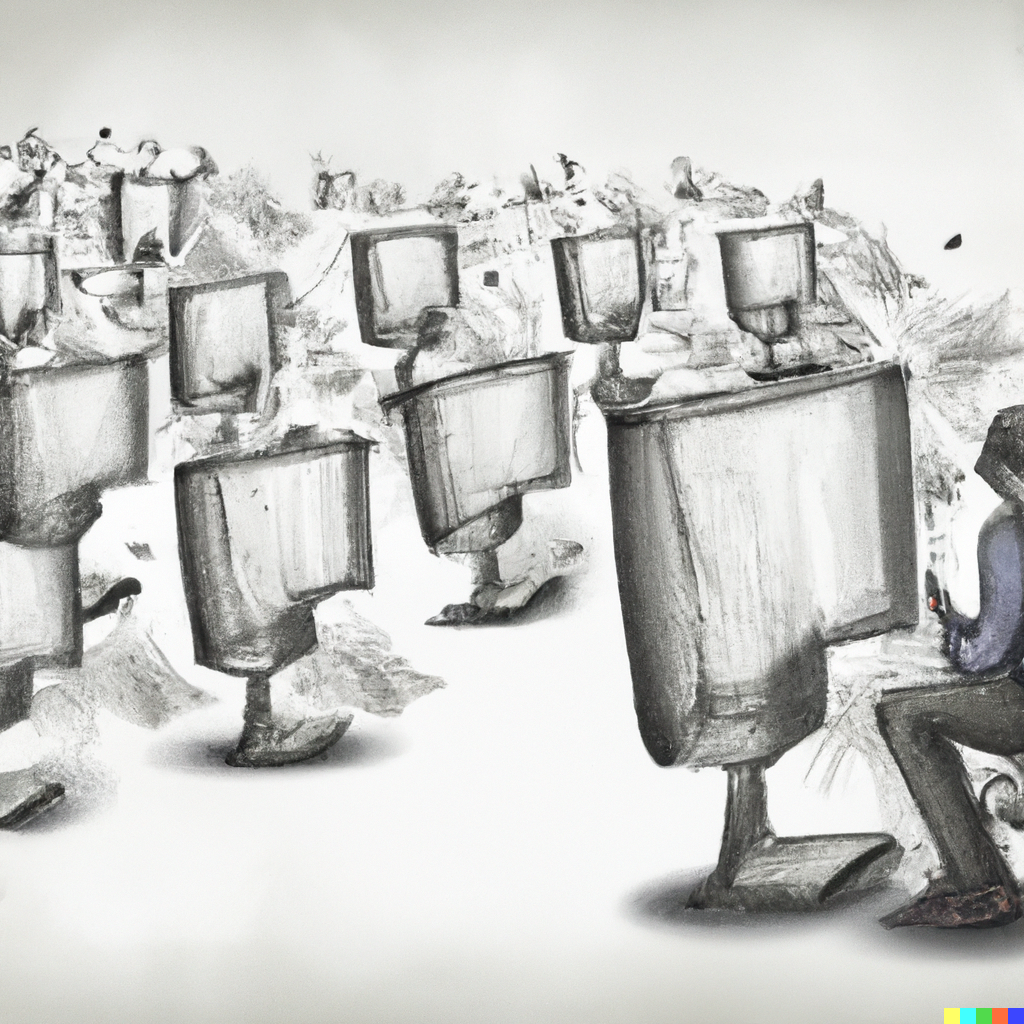
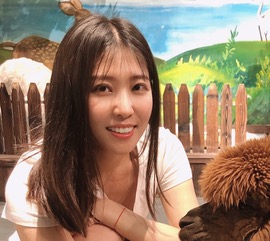
🔬 Research Summary by Yiting Qu, a Ph.D. student at CISPA Helmholtz Center for Information Security interested in AI Ethics and Safety.
[Original paper by Yiting Qu, Xinyue Shen, Xinlei He, Michael Backes, Savvas Zannettou, Yang Zhang]
Overview: Text-to-Image models are revolutionizing the way people generate images. However, they also pose significant risks in generating unsafe images. This paper systematically evaluates the potential of these models in generating unsafe images and, in particular, hateful memes.
Introduction
Text-to-Image models are generative models developed to generate images based on textual descriptions. Simply provide them with a text (a prompt), and they will produce images that perfectly illustrate the prompt in seconds. However, these models might be used in unexpected ways. Take Unstable Diffusion as an example, it is a profit-seeking community that automatically generates pornographic images using these models. This raises the question: what if a malicious user misuses these models and scales up the generation of unsafe images? Editor’s note: check out this MAIEI article highlighting some ethical issues and challenges with Unstable Diffusion.
To answer the question, we systematically evaluate the susceptibility of four popular Text-to-Image models in unsafe image generation. We collect three harmful prompt sets and one harmless prompt set, which are then fed into the Text-to-Image models for image generation. We assess the percentage of unsafe images in all generated images. The results show that 15.83% – 50.56% of the generated images are unsafe when models are intentionally misled with harmful prompts. Even when using harmless prompts, there is still a small possibility (i.e., 0.5%) of generating unsafe content.
Next, we zoom into a specific type of unsafe image: hateful memes. We envision a scenario where a malicious user aims to create variants of hateful memes using existing hateful symbols to attack specific individuals or communities. When combined with image editing techniques, we evaluate the potential of Stable Diffusion to generate these hateful meme variants. Compared to the variants manually drawn in the real world, we find that Stable Diffusion has a success rate of up to 24% in generating hateful meme variants that could be directly used to attack others. The quality and connotations of these AI-generated memes are comparable to real-world instances.
Key Insights
The Susceptibility of Text-to-Image Models in Unsafe Image Generation
The scope of unsafe images is broad and ambiguous. To capture the prominent unsafe content in AI-generated images, we adopt a data-driven approach and identify five categories of unsafe images: sexually explicit, violent, disturbing, hateful, and political. Within this defined scope, we assess the susceptibility of four open-source Text-to-Image models, Stable Diffusion, Latent Diffusion, DALL-E 2, and DALL-E-mini, using diverse sets of prompts.
Three harmful prompt sets are utilized to test the worst-case scenario when the models are exploited to generate unsafe content intentionally. These sets are derived from two main sources, 4chan and the Lexica website. We also include a clean prompt set using randomly sampled MS-COCO captions that describe normal objects. Using these prompts and the four Text-to-Image models, we generate 17K images. We then develop an image safety classifier to classify a generated image as safe or unsafe, specifying the category of unsafe content.
We have discovered that, on average, the four models have a 14.56% probability of generating unsafe images. Among them, Stable Diffusion is the most susceptible, with 18.92% of all generated images classified as unsafe; DALL-E 2 is the safest model, with only a 7.16% probability. However, this probability can increase significantly when intentionally provided with harmful prompts, ranging from 15.83% to 50.56% across the four models. What’s more concerning is that even when using a clean prompt set that describes normal objects, the models still have a 0.5% chance of generating unsafe images. Considering the large number of users and the frequent use of these models in daily life, these results indicate a significant risk of these models exacerbating the problem of unsafe image generation.
The Potential of Text-to-Image Models in Hateful Meme Generation
Hateful memes pose a large threat to online safety. In the real world, if a malicious user aims to attack specific individuals/communities via memes, one possible way is to find a notorious hateful meme, such as Pepe the Frog, and manually merge it with his target using Photoshop. The merged meme is referred to as the hateful meme variant. What would be the consequence if the malicious user aims to scale up the generation of hateful meme variants against a list of targets?
We develop an evaluation process focusing on Stable Diffusion and three image editing methods, including DreamBooth, Textual Inversion, and SDEdit. We use a dataset in the real world containing a list of hateful meme variants (manually drawn by humans) and the corresponding targets. The evaluation process starts with automatically generating prompts to describe how the targets are presented in hateful meme variants within this dataset. Next, we apply three image editing methods on top of Stable Diffusion and feed the designed prompts to generate variants. Finally, we compare the quality of generated and original variants using multiple assessment metrics.
Our research has found that 24% of hateful meme variants generated by Stable Diffusion are successful and could be used to attack the targets directly. Compared to real-world hateful meme variants, the generated ones, especially those with DreamBooth, have comparable image quality. This can be extremely concerning if malicious users launch a hate campaign online by producing many hateful meme variants.
Between the lines
The risk of Text-to-Image models in generating unsafe images should not be overlooked. Our research has found a significant likelihood of generating unsafe images, including sexually explicit, violent, disturbing, hateful, and political images. A particular concern is the automatic generation of hateful memes. It requires the user to input a piece of text into the model, reducing the cost compared to manually drawing. Considering the role of hateful memes in historical hate campaigns, we are concerned that current open-source Text-to-Image models might be exploited for the massive production of hateful memes and serve as a powerful weapon for orchestrated hate campaigns.
To mitigate the risk, model developers can make improvements from three aspects: 1) rigorously curating the training dataset of these models to minimize the proportion of unsafe images; 2) regulating user prompts and eliminating any unsafe keywords for models deployed online; and 3) training more accurate image safety detectors that report unsafe images, particularly hateful memes.