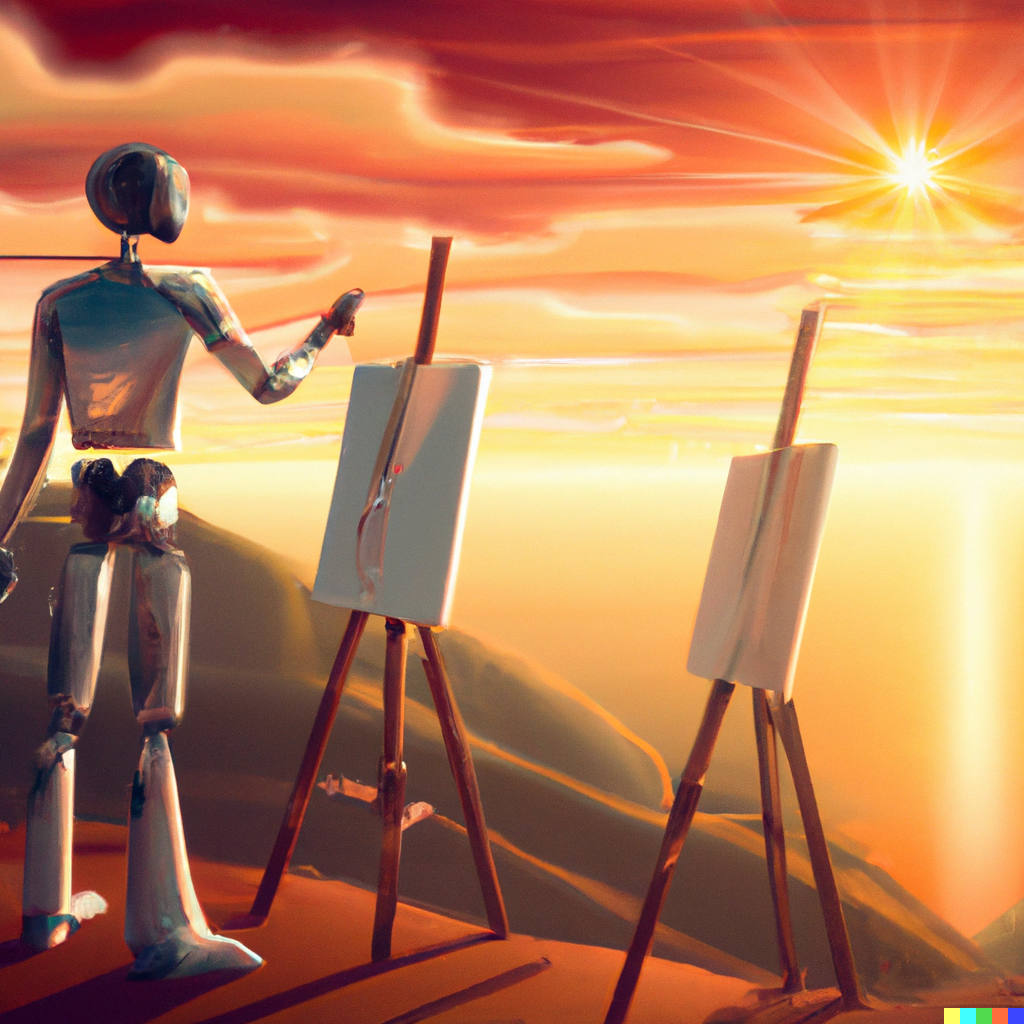
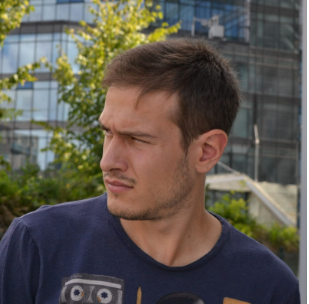
🔬 Research Summary by Giorgio Franceschelli, a second-year Ph.D. student at the University of Bologna working on Generative Artificial Intelligence, Reinforcement Learning, and creativity.
[Original paper by Giorgio Franceschelli and Mirco Musolesi]
Overview: Large Language Models (LLMs) like ChatGPT are revolutionizing several areas of AI, including those related to creative writing. This paper offers a critical discussion of LLMs in the light of human theories of creativity, including the impact these technologies might have on society.
Introduction
Large Language Models (LLMs) have recently captivated the imagination of millions of people. They have been used to generate journal articles, culinary recipes, and university-level essays and papers about themselves. In particular, they have been used to perform creative tasks like writing songs or concluding the plots of novels; the results are often remarkable. Notwithstanding, it is not obvious whether LLMs are truly creative, at least in the sense discussed by Ada Lovelace in 1842, when she argued that ‘’[a machine] has no pretensions to originate anything. It can do whatever we know how to order it to perform’’.
In our paper, we therefore critically analyze LLMs under the lens of the most prominent theories of creativity, from Boden’s three criteria to the 4P’s of creativity by Rhodes (product, process, press, and person).
Current models satisfy only some of the requirements due to their intrinsic limitations. Finally, we survey the potential social impact of creative AI, which can open several opportunities in terms of the “democratization” of AI technologies and potentially lead to negative implications for some sectors if not sufficiently regulated.
Key Insights
Can LLMs be considered creative?
LLMs and Boden’s Three Criteria
Margaret Boden defines creativity as “the ability to come up with ideas or artifacts that are new, surprising, and valuable.” While hundreds of definitions have been provided throughout history, Boden’s definition has achieved a certain consensus, especially in the field of computational creativity.
Defining AI creativity is challenging. LLMs are certainly able to produce valuable artifacts, both in terms of utility and in terms of attractiveness. On the other hand, novelty is more difficult to assess. If it is intended as “not being in existence before,” the variety of prompts and their stochastic nature commonly lead to novel outcomes. However, suppose it is interpreted as the dissimilarity between the produced artifact and other examples in its class. In that case, it will be limited by design: LLMs are trained to generate words/tokens that are most likely to follow previous ones according to the training set, i.e., the output will be somehow close to what others have written in the past. Similar considerations can be made for the concept of surprise: the nature of LLMs allows for creating unfamiliar combinations of familiar ideas (i.e., combinatorial creativity). Careful prompting could lead to “unexplored” outputs inside the current style of thinking (i.e., exploratory creativity), like writing poems on mathematical theorems. However, the purest form of creativity related to changing that style of thinking (i.e., transformational creativity) is out of discussion, at least with the technologies available today. In fact, current training techniques that rely on self-supervised training or fine-tuning to avoid generating inappropriate/unwanted content (for example, because it is not completely factual) make LLMs only capable of weaker forms of creativity.
Easy and Hard Problems in Machine Creativity
Producing creative outputs does not always translate into being creative: it is not only what is achieved but also how it is achieved that matters. In 1961, Rhodes suggested that the product is insufficient: we must also consider the process, press, and person perspectives.
The creative process consists of task motivation, plus generation, validation, and communication of the selected response. LLMs can only generate and communicate responses without validating them; they only deal with given problems without any inner motivation. The creative press refers to the relationship between the product and the influence its environment has upon it, commonly in terms of a never-ending cycle where new and valuable products are based on the current domain, which is, of course, changed by them. While LLMs correctly base their outputs on the provided domain, at the moment, they lack this intrinsic adaptation during the creative process. Finally, the creative person covers information about intellect, temperament, habits, attitude, and value systems. While it would be possible to implement some forms of validation or continual adaptation (which are easy problems), dealing with self-awareness and personality is a hard problem.
Practical Implications of Creative AI
Legal Side
Intellectual property in the context of Generative AI is currently a hotly debated topic since most laws do not explicitly contemplate non-human authors (see, for example, our previous work on generative learning and copyright). Copyright applies to works showing a minimum degree of originality and reflecting the author’s personality. Current LLMs might satisfy the first condition but not the latter requirement; works produced by them can be protected only if the original contribution is provided by a human, e.g., the user. The definition of originality in this context is a key open problem.
A more creative AI model might also help deal with copyright infringement. Current LLMs are trained on real data and may learn to reproduce portions of them, violating the copyright of works in the original training set. A creative-oriented training approach that fosters novelty might mitigate such risks.
Social Side
We believe that the overall impact of a more creative AI will be positive. LLMs provide new opportunities for humans, allowing them to spend more time validating news or thinking up ideas, to easily adapt an artifact for different audiences, to inspire researchers with new hypotheses and artists with new imagined possibilities.
However, it is important to point out their risks as well. As the quality of their outputs improves, certain roles in the professional writing industry might be threatened or disappear altogether. LLMs can also exploit certain ideas from human authors, capitalizing on their efforts in cheaper ways; more creative models should reduce this risk, but, at the same, they might hurt the market of human-generated works. Finally, LLMs might produce works indistinguishable from human ones: users of LLMs might deceptively claim themselves to be their authors without mentioning the assistance of these technologies.
Between the lines
Due to the astonishing outputs LLMs can produce, there is a clear temptation to anthropomorphize them. However, their intrinsic nature leads to a type of creativity that is different from the human one, at least with the currently available technologies. Measuring AI creativity is essentially an open problem (see our proposal here). The “surprise” of LLMs is somehow limited by the given training sets. In particular, they are not self-aware or motivated to make creative artifacts. Having said that, we believe their potential is really enormous.
There are several concerns that practitioners and researchers have to consider in this “brave new world”: in particular, how to use this technology to improve the democratization of these technologies and how it can be used in a way that will not affect the creative industries negatively.
We definitely live in interesting times.