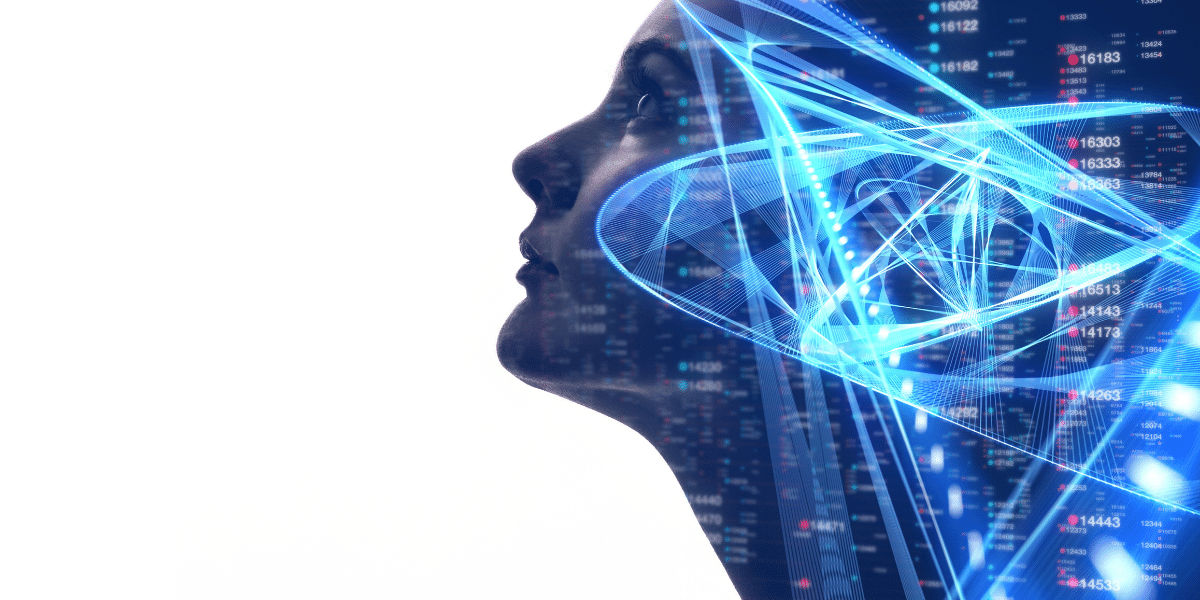
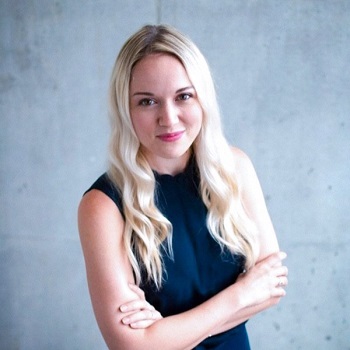
🔬 Research summary by Victoria Heath (@victoria_heath7), our Associate Director of Governance & Strategy.
[Original paper by Alessandro Saffiotti, Peter Fogel, Peter Knudsen, Luise de Mirando, Oscar Thörn]
Overview: How can AI systems enhance the interactions between a human and an artificial performer to create a seamless joint performance? In this paper, researchers create a new model for human-AI collaboration using an AI system to mediate between the agents and test the model in three different performance scenarios.
Introduction
Artificial intelligence (AI) is here to stay—the question is, how will these systems be integrated into our daily lives? Further, what will be the impact? These questions have led many to the conclusion that we must develop effective collaborative models which encourage collaboration between humans and AI-based artificial agents rather than augmentation or replacement models. This aligns with what is outlined in the Ethics Guidelines recently adopted by the European Commission which “insists that humans should maintain agency and oversight with respect to AI systems.”
To further research on collaborative models, researchers Alessandro Saffiotti, Peter Fogel, Peter Knudsen, Luise de Mirando, and Oscar Thörn turn towards the creative arts and create a new collaboration model that uses an AI system as a mediator between a human and artificial performer. They test this model in three different case studies: 1) between a pianist and a virtual drummer, 2) between dancers and a virtual drummer, and 3) between a pianist and a robot dancer. Following these tests, the authors affirm their hypothesis that the AI system is capable of effectively creating a “harmonious joint performance” between the human and artificial performer.
A model for human-AI collaborative artist performance
The collaboration model created by Saffiotti et al is rather simple: an AI system is “used as a mediator to coordinate the performance of two autonomous agents,” the human performer and the artificial performer. Artificial performer, in this case, can include any agent that “generates physical outcomes.” In the following case studies, the artificial performers are a virtual drummer and a humanoid robot. The model has three features: 1) supervisory (the AI system does not generate a creative output directly), 2) reactive (the AI system analyzes the human’s artistic expression and adapts the parameters of the artificial performer to match it), and 3) proactive (the AI system can set the performance parameters proactively based on the human performance). These features, explain Saffiotti et al, implement “the two directions in human-AI co-creativity,” with the ultimate goal of aligning the “artistic expressions of the two performers, each one realized through its specific expressive means.” The AI system used in this collaborative model contains two distinct modules:
- Features extraction: this module uses inputs from the human performer (past and present) to generate estimates of the value of a set of variables related to the “musical expression” of the performer. In the case studies outlined below, this can take the form of keypress velocity, rhythmic density, etc.
- Parameter generation: this module takes the variables from the features extraction to “decide the values of the execution parameters of the artificial agent.” By doing so, it is providing the artificial performer with information to adjust its performance based on the musical expression of the human performer. For example, these parameters can include the intensity and complexity of drumming.
It’s important to note that the AI system itself does not generate performance outputs (e.g. music) but performance parameters instead. The architecture of their AI system, explain Saffiotti et al, relies on a knowledge-based approach in which knowledge from the human performers is encoded into the system as “fuzzy logic” using multiple-input multiple-output Fuzzy Inference Systems. For example, the knowledge provided by the human performers was expressed in terms like, “If rhythmic complexity on the lower register is high, then rhythmic complexity of drums should increase strongly.” All of the case studies below utilize this system with some minor tweaks.
The model in action: three case studies
The first test conducted using this collaborative model was between a human pianist and a virtual drummer at two public concerts in Sweden. Before these improvised jazz performances, the tempo and style were agreed upon and used as a setting for the virtual drummer, while the other parameters were set in real-time using the model. Feedback from the performer following the concerts was positive, describing the AI-controlled drummer as “human-like” and “proactive” and “surprising” in its performance. The researchers note that this feeling of “proactiveness” or anticipatory behaviour may be the result of the use of expectations in parameter generation where the system is using patterns to anticipate the artist’s behaviour and adjusting the parameters accordingly.Â
The second test was between two human dancers and a virtual drummer at Music Tech Fest in 2019. In collaboration with the dancers, they decided which “features” to analyze and how the drummer should “react.” For example, the dancers’ distance from each other would change the virtual drummer’s drumming pattern. The third test was between a human pianist, a robot dancer (Softbank Robotics’ commercial robot Pepper), and a virtual drummer during a live performance. The robot and the virtual drummer received parameters set by the AI system (similar to the first case study).
How should collaborative performances be evaluated?
While the authors received unstructured feedback following each test (primarily positive), there remained questions as to how to measure these performances in order to understand the model’s effectiveness. Is the “subjective experiences from humans” enough to evaluate the model?
In order to gather more structured feedback on the effectiveness of their model, Saffiotti et al created an online user study with over 90 subjects. They created two versions of a case study with the human pianist, virtual drummer and robot: a test version which used the AI model to decide the parameters for the robot’s performance and a control version which selected those parameters randomly. By doing so, they aimed to test the collaboration aspect of the performance. The results from this study indicate that their model successfully aligns the artificial agent’s performance with the human’s, leads to a sense of “collaborative performance,” and may lead to a higher perception of “artistic value.”
Between the lines
While the case studies outlined above affirmed the researcher’s hypothesis—an AI system would effectively create a “harmonious joint performance” between the human and artificial performer—they acknowledge that more research needs to be done. In particular, Saffiotti et al aim to replace their knowledge-based approach with a more data-driven approach in order to “complete the hard-written rules, or to adapt them to the artistic taste of a given musician.” By making the feature extraction and parameter generation modules more precise and robust, they can further understand the role an AI system can play in mediating between a human and a machine.