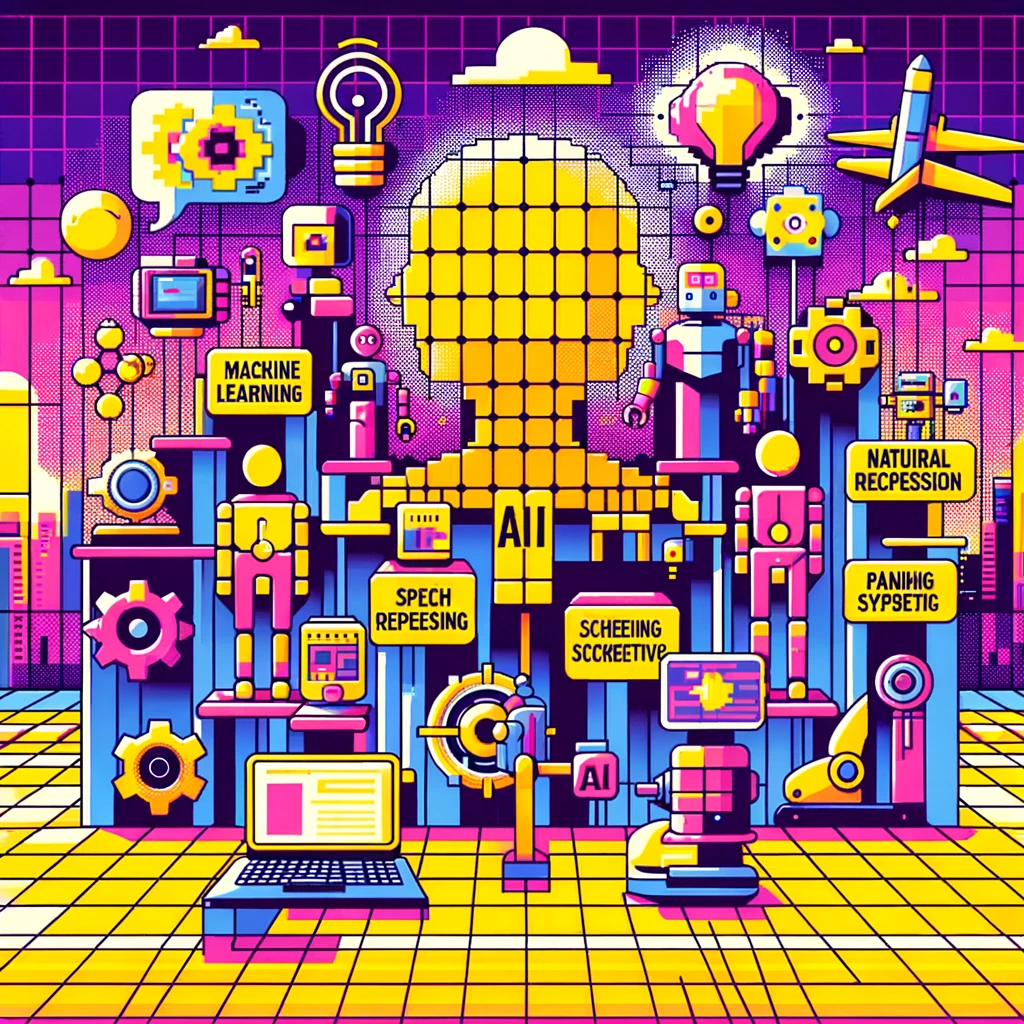
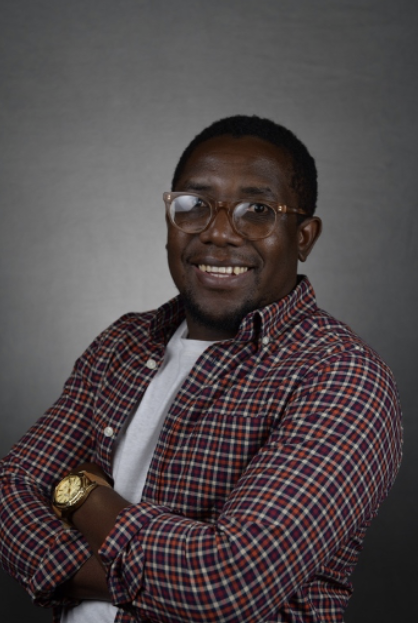
🔬 Research Summary by Gregory Gondwe, an Assistant Professor of Journalism at California State University – San Bernardino and a Harvard faculty Associate with the Berkman Klein Centre.
[Original paper by Gregory Gondwe]
Overview: This study aims to create typologies for analyzing Artificial Intelligence (AI) in journalism and mass communication research by identifying seven distinct subfields of AI, including machine learning, natural language processing, speech recognition, expert systems, planning, scheduling, optimization, robotics, and computer vision. The study’s primary goal is to provide a structured framework to assist AI researchers in journalism in understanding these subfields’ operational principles and practical applications, thereby enabling a more focused approach to analyzing research topics in the field.
Introduction
Artificial Intelligence (AI) is reshaping the landscape of journalism and mass communication research. With AI applications proliferating, understanding their subfields is crucial for comprehending their impact. Machine learning has been pivotal, with AI-generated content demonstrated to create convincing misinformation at scale. Natural language processing (NLP) has enabled sentiment analysis in news articles, offering insights into public reactions. Robotics, exemplified by AI-powered chatbots, is changing news reporting.
This study dives into AI in journalism, focusing on typologies to aid comprehensive investigations. It presents a framework that helps researchers explore AI’s multifaceted presence within journalism, highlighting seven fundamental subfields, such as machine learning and NLP. The goal is to offer a systematic approach for media scholars to navigate AI’s diverse facets, allowing researchers to focus on specific AI applications relevant to their study. By understanding AI’s subfields, scholars can delve into issues like AI-generated content’s impact on media consumption and automation in news reporting. This study highlights the practical implications of AI typologies in journalism research, enabling a deeper understanding of automated news production, personalized content delivery, and the challenges of AI-generated misinformation. Ultimately, it contributes to the expanding knowledge of AI in journalism and empowers researchers to conduct informed investigations in this evolving field, anticipating AI’s future in the media industry.
Key Insights
Arguments Surrounding Artificial Intelligence in Journalism Studies
Three main themes have emerged in the evolving world of AI and journalism. First, AI is seen as a tool to make journalism more efficient by automating tasks like content curation, personalized recommendations, and transcription. This automation is believed to free up journalists to focus on more in-depth reporting. Second, there are concerns about the quality and accuracy of AI-generated content. While AI can help with fact-checking, it can also produce misinformation or biased content. It’s essential to have strict editorial oversight to ensure accurate information dissemination. Third, ethical considerations come to the forefront. AI is often based on Western values and capitalism, which can lead to biases, the exclusion of marginalized communities, and the commodification of personal data. There are also concerns about AI-driven content personalization creating information bubbles.
Understanding the different types of AI is crucial to navigate these complex issues. A recent study developed a taxonomy of generative AI, categorizing AI into nine types, including text-to-image, text-to-audio, and text-to-science models, each serving different functions. This taxonomy helps researchers and journalists make sense of the AI landscape and its implications in journalism and mass communication.
Generative AI in Journalism and Communication Studies: A Multidimensional Exploration
Generative AI is a dynamic and evolving field that holds vast potential, and its significance is particularly pronounced within the academic domains of journalism and communication studies. The application of generative AI techniques in these fields has the capacity to revolutionize traditional practices in news reporting, content creation, and audience engagement. This transformation can encompass a spectrum of possibilities, ranging from automated content generation to personalized content recommendations, sentiment analysis, automated transcription, and translation services.
However, it’s crucial to acknowledge that the integration of generative AI in journalism and communication studies is not uniform across all its dimensions. While the promise of AI is clear, only a select few AI subfields have found practical utility in these areas, with the majority of AI innovations still in the developmental phase. The reasons behind this asymmetry are multifaceted. As a field, AI is constantly evolving, with new subfields and technologies emerging rapidly. This dynamism often challenges academic and industry communities in terms of understanding, adopting, and adapting to these innovations.
In the context of journalism and communication studies, the gradual integration of AI technologies is a complex process that requires both technical and ethical considerations. Adopting generative AI tools demands rigorous editorial oversight to ensure that content produced by AI adheres to high standards of accuracy, fairness, and ethical principles. Moreover, the increasing reliance on AI-driven content personalization in the media landscape has prompted concerns about creating information bubbles, where users are exposed to content that aligns with their existing beliefs, potentially hindering diverse perspectives and discourse. This taxonomy categorizes generative AI into various subfields, each with distinct functionalities, from text-to-image to text-to-science models. A nuanced grasp of these subfields is essential for scholars and practitioners to harness the full potential of generative AI while addressing its associated challenges and ethical implications.
Between the lines
The study centers on the multifaceted implications of incorporating generative AI in journalism and communication studies. It highlights the selective integration of AI subfields in these domains due to the evolving nature of AI technologies, emphasizing the need for continuous research and adaptation. Editorial oversight emerges as a critical aspect in ensuring the accuracy and reliability of AI-generated content. Moreover, ethical concerns, including bias, exclusion, data centralization, and the potential formation of information echo chambers, underscore the importance of addressing societal and ethical implications in the integration of AI.