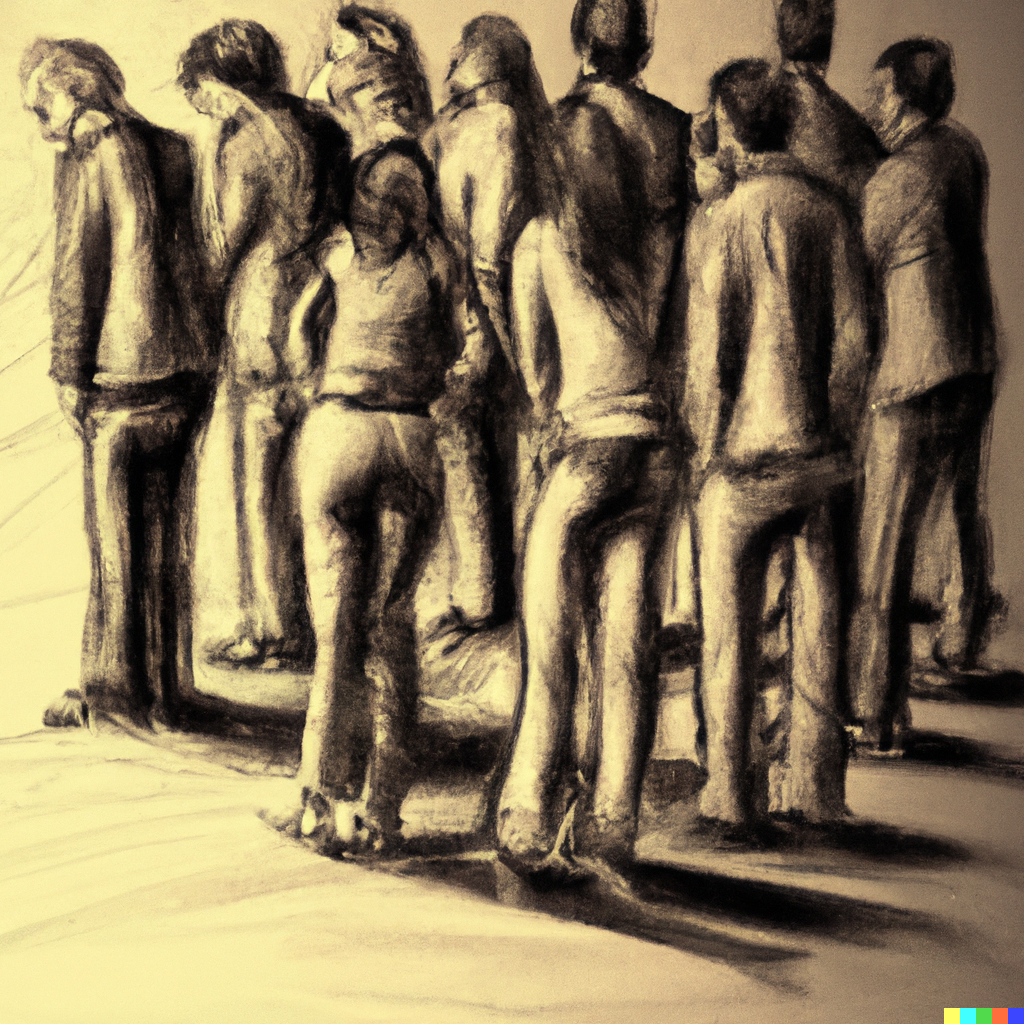
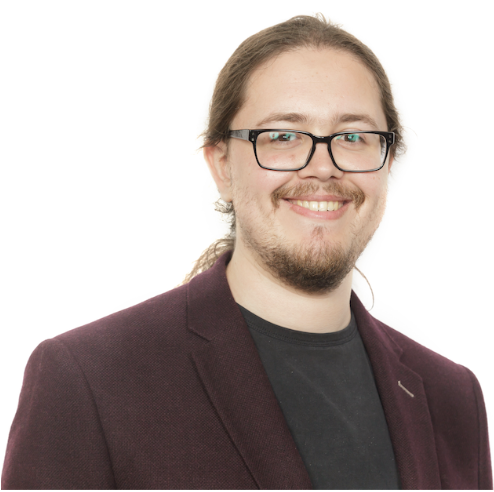
🔬 Research Summary by Ismael Kherroubi Garcia, trained in business management, and philosophy of the social sciences. He is the founder and CEO of Kairoi, the AI Ethics and Research Governance Consultancy.
[Original paper by Mylene Petermann (Ada Lovelace Institute), Niccolo Tempini (Senior Lecturer in Data Studies at the University of Exeter’s Institute for Data Science and Artificial Intelligence), Ismael Kherroubi Garcia (Kairoi), Kirstie Whitaker (Alan Turing Institute), Andrew Strait (Ada Lovelace Institute)]
Overview: Products and services built through AI and data science research can substantially affect people’s lives, so research must be conducted responsibly. In many corporate and academic research institutions, a primary mechanism for assessing and mitigating risks is using Research Ethics Committees (RECs), also known in some regions as Institutional Review Boards (IRBs). This report explores academic and corporate RECs’ role in evaluating AI and data science research, providing recommendations to businesses, academia, policymakers, and funders working in this context.
Introduction
AI tools are widely applicable, and their different uses raise different controversies. Consider the case of ChatGPT being deployed in an experiment by a nonprofit healthcare provider. In short, the experiment consisted of mental health supporters using ChatGPT to “write more supportive responses quickly.” Meanwhile, the nonprofit’s founder claimed the study to be “exempt” from requiring informed consent from users. This stance has fuelled countless debates over social media. These are also the types of questions RECs are best equipped to manage.
Since the 1960s, RECs have been empowered to review research before it is undertaken and to reject proposals unless the proposed research design meets certain ethical standards. However, RECs have generally been established to handle biomedical research. Most academic and corporate RECs’ current role, scope, and function need to be revised for the novel challenges that AI and data science research pose.
Through an extensive literature review, workshops, and interviews with experts from academia and industry, we identify six major challenges RECs face when working in the context of AI and data science research. We make eight recommendations to research institutes, industry, and the broader AI and data science ecosystem.
Key Insights
Challenges faced by RECs
- Many RECs lack the resources, expertise, and training to address the risks that AI and data science pose appropriately
- Traditional research ethics principles are not well suited for AI research, as they assume a closer researcher-subject relationship, as found in biomedical research
- Specific principles for AI and data science research are still emerging and are not consistently adopted by RECs
- Multi-site and public-private partnerships can exacerbate existing challenges of governance and consistency in decision-making processes
- RECs struggle to review potential harms and impacts that arise throughout AI and data science research
- Corporate RECs lack appropriate transparency concerning their processes
Recommendations
For academic and corporate RECs
#1: Incorporate broader societal impact statements from researchers.
AI and data science research communities have called for researchers to incorporate moral considerations at various stages of their work, from peer review to conference submissions. RECs can support these efforts by incentivizing researchers to engage in reflexive exercises to consider and document the broader societal impacts of their research.
#2: RECs should adopt multi-stage ethics review processes of high-risk AI and data science research.
Many challenges that AI and data science raise will arise at different research stages. RECs should experiment with requiring multiple evaluation stages for high-risk research. For example, a REC can evaluate projects at both the point of data collection and the point of publication.
#3: Include interdisciplinary and experiential expertise in REC membership.
Many of AI and data science research’s risks can only be understood by engaging with diverse experiences and expertise. RECs must be interdisciplinary to address the myriad issues that AI and data science can pose in different domains. RECs must incorporate the perspectives of those impacted by the research and its outputs.
For academic and corporate research institutions
#4: Create internal training hubs for researchers and REC members, and enable cross-institutional knowledge sharing.
Cross-institutional knowledge-sharing can ensure institutions do not develop standards of practice in silos. Training hubs should collect and share information on the ethical issues and challenges AI and data science research might raise, including case studies supporting reflexive exercises. In addition to our report, we have developed a resource of six case studies highlighting the ethical challenges RECs might face.
#5: Corporate labs must be more transparent about their decision-making and engage more with external partners.
Corporate labs face specific challenges regarding AI and data science reviews. While many are better resourced and have experimented with broader societal impact thinking (compared to academic RECs), some of these labs have faced criticism for being opaque about their decision-making processes. Many of these labs make consequential decisions about their research without engaging with local, technical, or experiential expertise that resides outside their organizations.
For funders, conference organizers, and the broader research ecosystem
#6: Develop standardized principles and guidance for AI and data science research.
National research governance bodies like UKRI should work to create a new set of ‘Belmont 2.0’ principles that offer standardized approaches, guidance, and methods for evaluating AI and data science research. Developing these principles should draw on diverse perspectives from different disciplines and communities impacted by AI and data science research, including multinational perspectives – particularly from regions historically underrepresented in the development of past research ethics principles.
#7: Actors across the research ecosystem should incentivize a responsible research culture.
AI and data science researchers lack the incentives to reflect on and document the societal impacts of their research. Different actors in the research ecosystem can encourage ethical behavior. Funders, for example, can create requirements that researchers develop societal impact statements to receive a grant. Meanwhile, conference organizers and journal editors can encourage researchers to include such statements when submitting research. By creating incentives throughout the research ecosystem, ethical reflection can become more desirable and be rewarded.
#8: Policymakers should increase funding and resources for ethical AI and data science research reviews.
There is an urgent need for institutions and funders to support RECs, including paying for the time of staff and funding external experts to engage in questions of research ethics. The traditional approach to RECs has treated their labor as voluntary and unpaid. RECs must be properly resourced to meet AI and data science challenges.
Between the lines
There is no need to reinvent the wheel for AI and data science research. RECs and broader research governance departments have been around for decades. Our report highlights the opportunity to tap into this rich resource. And we have come a long way since RECs first emerged.
We now know we need a shift in academia from a culture of “publish-or-perish” to one of research integrity. We also have evidence that diverse teams fuel innovation. These are aspects our report points to and which RECs in general – not just in AI and data science – can work to improve.
More specifically, we know data science education needs more focus on ethics. We know AI systems risk exacerbating racial and societal inequalities. We know there are many sets of moral principles we can write into AI ethics frameworks. However, we must move on from debating values to operationalizing responsible AI practices. While RECs will need adapting and adequate resourcing, they can help drive the movement toward responsible AI and data science research.