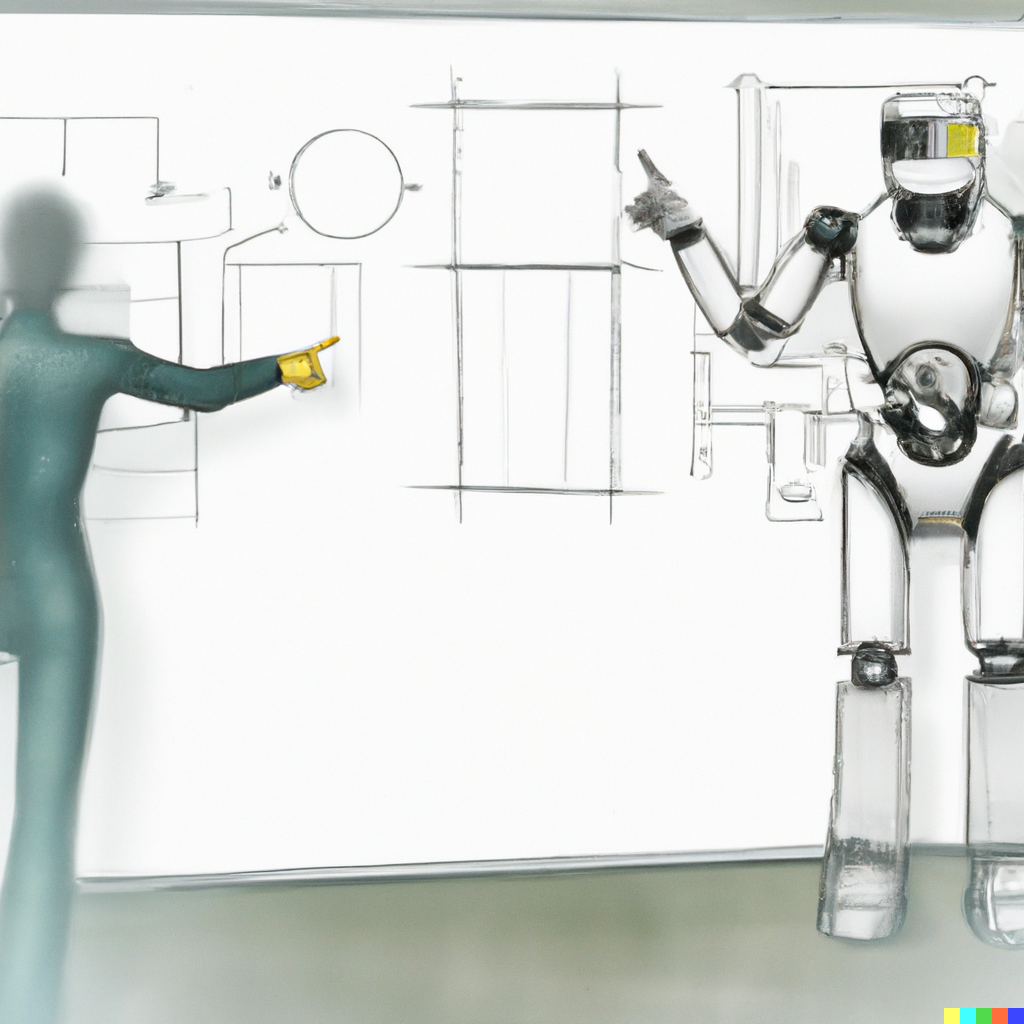
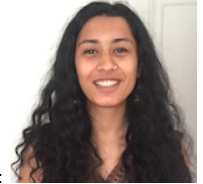
🔬 Research Summary by Charvi Rastogi, a Ph.D. student in Machine Learning at Carnegie Mellon University. She is deeply passionate about addressing gaps in socio-technical systems to help make them useful in practice, when possible.
[Original paper by Charvi Rastogi, Marco Tulio Ribeiro, Nicholas King, Harsha Nori, and Saleema Amershi]
Overview: While large language models (LLMs) are being increasingly deployed in sociotechnical systems, in practice, LLMs propagate social biases and behave irresponsibly, imploring the need for rigorous evaluations. Existing tools for finding failures of LLMs leverage either or both humans and LLMs, however, they fail to bring the human into the loop effectively, missing out on their expertise and skills complementary to those of LLMs. In this work, we build upon an auditing tool to support humans in steering the failure-finding process while leveraging the generative skill and efficiency of LLMs.
Introduction
In the era of ChatGPT, where people increasingly take assistance from a large language model in day-to-day tasks such as information search, making these models safe to use by the general public through rigorous audits is of utmost importance. However, LLMs have an incredibly wide-ranging applicability, making testing their behavior on each possible input practically infeasible. To address this, we design an auditing tool, AdaTest++, that effectively leverages generative AI and human auditors to create a powerful partnership. It is focused on emphasizing complementary skills of generative AI, such as prolific and efficient generation, creativity and randomness, and limited sociocultural knowledge, and those of humans, such as social reasoning, contextual awareness of societal frameworks, and intelligent sensemaking.
We conducted a user study where participants audited two commercial language models: OpenAI’s GPT-3 [1] for question-answering capabilities and Azure’s text analysis model for sentiment classification, using our tool. We observed that users successfully leveraged their relative strengths in an opportunistic combination with the generative strengths of LLMs. Collectively, they identified a diverse set of failures efficiently, covering several unique topics, and discovered many types of harms such as representational harms, allocational harms, questionable correlations, and misinformation generation by LLMs, thus opening promising directions for the use of human-LLM collaborative auditing systems.
Key Insights
What is auditing?
An algorithm audit is a method of repeatedly querying an algorithm and observing its output to draw conclusions about the algorithm’s opaque inner workings and possible external impact.
Why support human-LLM collaboration in auditing?
Red-teaming will only get you so far. A red team is a group of professionals generating test cases on which they deem the AI model likely to fail, a common approach used by big technology companies to find failures in AI. However, these efforts are sometimes ad-hoc, depend heavily on human creativity, and often lack coverage, as evidenced by recent high-profile deployments such as Microsoft’s AI-powered search engine, Bing, and Google’s chatbot service, Bard. While red-teaming serves as a valuable starting point, the vast generality of LLMs necessitates a similarly vast and comprehensive assessment, making LLMs an important part of the auditing system.
Human discernment is needed at the helm. LLMs, while widely knowledgeable, have a severely limited perspective of the society they inhabit (hence the need for auditing them!). Humans have a wealth of understanding to offer through grounded perspectives and personal experiences of harms perpetrated by algorithms and their severity. Since humans are better informed about the social context of the deployment of algorithms, they can bridge the gap between the generation of test cases by LLMs and the test cases in the real world.
Despite these complementary benefits of humans and LLMs in auditing, past work on collaborative auditing relies heavily on human ingenuity to bootstrap the process (i.e., to know what to look for) and then quickly become system-driven, which takes control away from the human auditor. In this work, we design collaborative auditing systems where humans act as active sounding boards for ideas generated by the LLM.
How to support human-LLM collaboration in auditing?
We investigated the specific challenges in an existing auditing tool, AdaTest. Based on our auditing and human-AI collaboration research, we identified two key design goals for our new tool, “AdaTest++,” supporting human sensemaking and human-LLM communication.
To support failure finding and human-LLM communication, we add a free-form input box where auditors can request particular test suggestions in natural language by directly prompting the LLM, e.g., Write sentences about friendship. This allows auditors to communicate their search intentions efficiently and effectively and compensate for the LLM’s biases. Further, since effective prompt crafting for generative LLMs is an expert skill, we craft a series of prompt templates encapsulating expert strategies in auditing to support auditors in communicating with the LLM inside our tool. Some instantiations of our prompt templates are given below for reference:
Prompt template: Write an output type or style test that refers to input features.
Usage: Write a movie review that is sarcastic and negative and refers to the cinematography.
Prompt template: Write a test using the template “template using {insert},” such as “example.”
Usage: Write a movie review using the template “the movie was as {positive adjective} as {something unpleasant or boring}” such as “the movie was as thrilling as watching paint dry.”
Does supporting human-AI collaboration in auditing actually help?
We conducted think-aloud user studies with our tool AdaTest++, wherein people with varying expertise in AI (0-10 years) audited language models for harm. We applied mixed-methods analysis to the studies and their outcomes to evaluate the effectiveness of AdaTest++ in auditing LLMs.
With AdaTest++, people discovered a variety of model failures, with a new failure discovered roughly every minute and a new topic every 5-10 minutes. Within half an hour, users collectively identified many failure modes, including failures previously under-reported in the literature. Users successfully identified several types of harms, such as allocational harms, representation harms, and others provided in a harm taxonomy. They also identified gaps in the specification of the auditing task handed to them, such as test cases where the “correct output” is not well-defined, supporting the re-design of the task specification for the LLM.
We observed that users executed each stage of sensemaking: surprise, schematization, and hypotheses formation often, which helped them develop and refine their intuition about the algorithm being audited. The studies showed that AdaTest++ supported auditors in both top-down and bottom-up thinking and helped them search widely across diverse topics and dig deep within one topic.
Importantly, we observed that AdaTest++ empowered users to use their strengths more consistently throughout the auditing process while benefiting significantly from the LLM. For example, some users followed a strategy where they queried the LLM via prompt templates (which they filled in) and then conducted two sensemaking tasks simultaneously: (1) analyzed how the generated tests fit their current hypotheses and (2) formulated new hypotheses about model behavior based on tests with surprising outcomes. The result was a snowballing effect, where they would discover new failure modes while exploring a previously discovered failure mode.
Between the lines
As LLMs become powerful and ubiquitous, it is important to identify their failure modes to establish guardrails for safe usage. Towards this end, equipping human auditors with equally powerful tools is important. Through this work, we highlight the usefulness of LLMs in supporting auditing efforts towards identifying their own shortcomings, necessarily with human auditors at the helm, steering the LLMs. LLMs’ rapid and creative generation of test cases is only as meaningful towards finding failure cases as judged by the human auditor through intelligent sensemaking, social reasoning, and contextual knowledge of societal frameworks. We invite researchers and industry practitioners to use and further build upon our tool to work towards rigorous audits of LLMs.
Notes
[1] At the time of this research, GPT-3 was the latest model available in the GPT series.