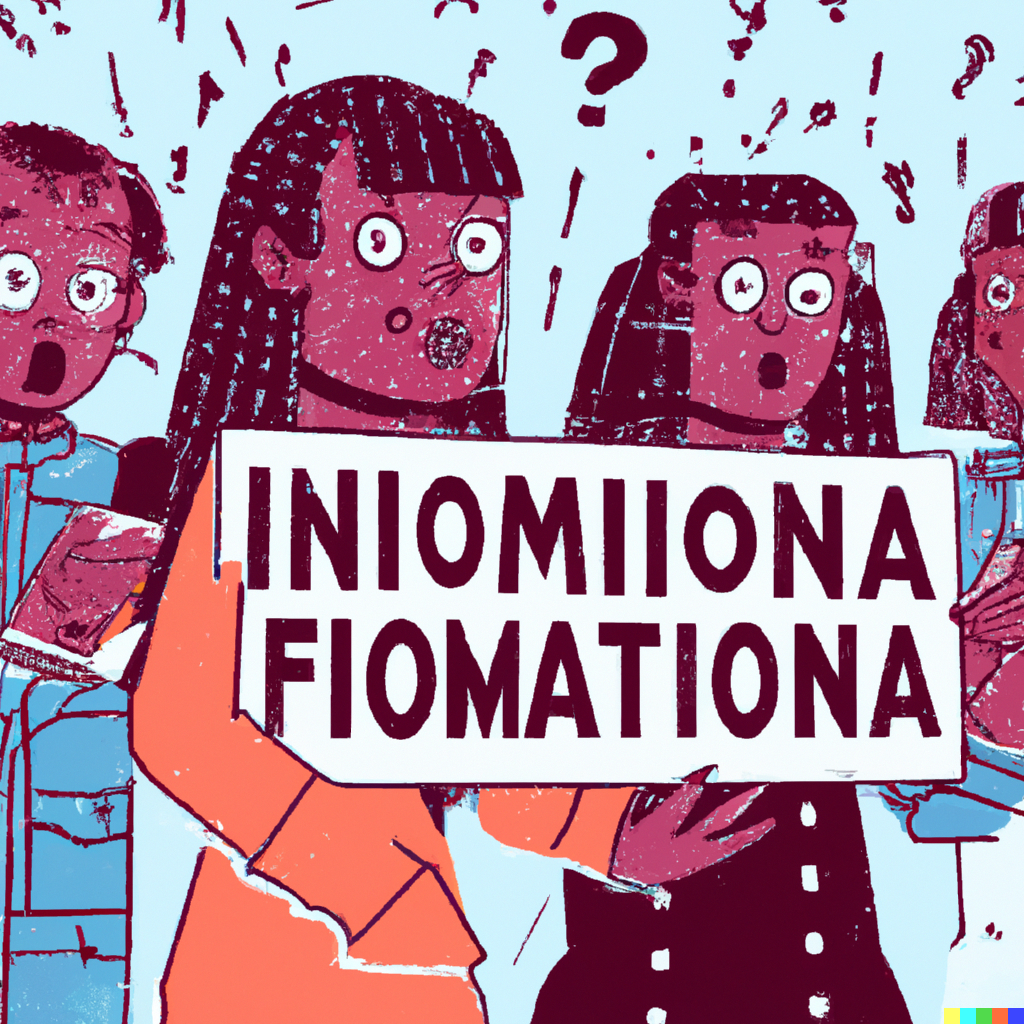
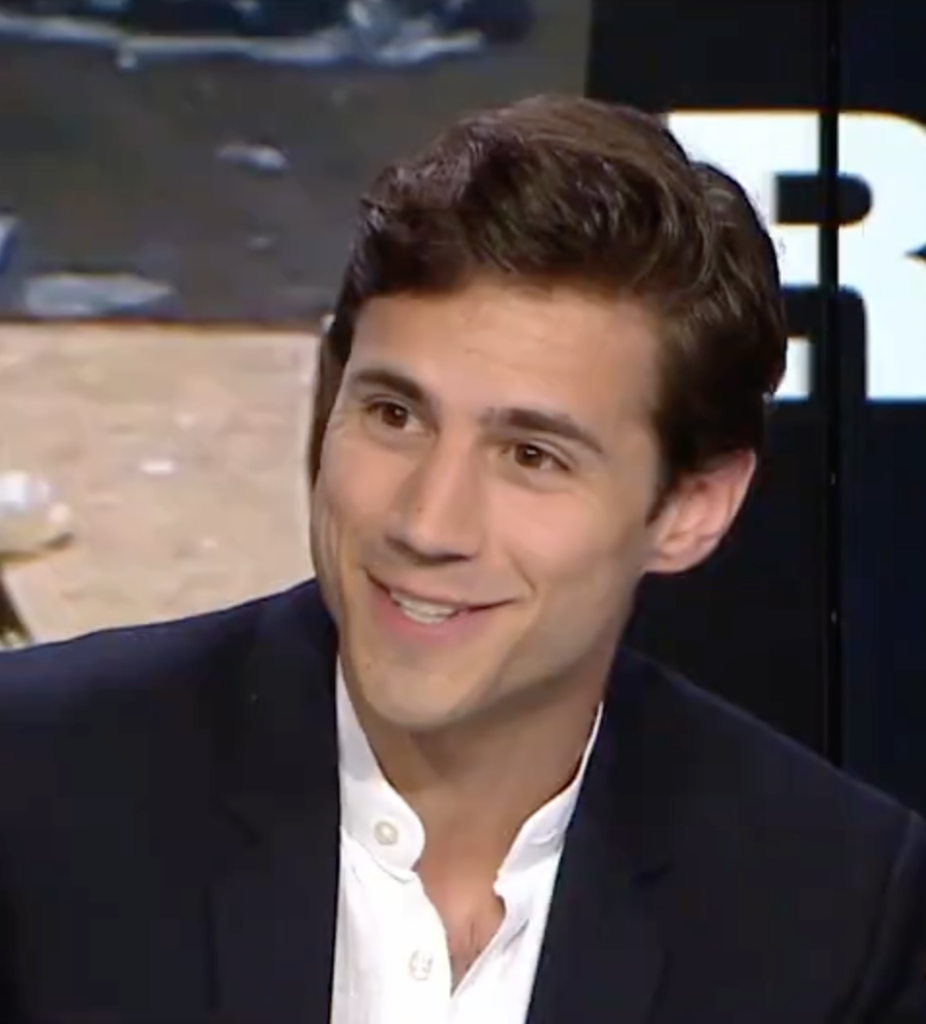
🔬 Research Summary by Hubert Etienne, a researcher in AI ethics, the former Global Generative AI Ethics Lead at Meta and the inventor of Computational philosophy.
[Original paper by Hubert Etienne and Onur Çelebi]
Overview: This paper sheds a whole new light on online misinformation, analyzing content reported by Facebook and Instagram users in France, the UK, and the US. It combines mixed methods to better understand what users report as ‘false news’ and why. It presents several major findings with great implications for regulators to compare the state of online misinformation in different countries and for social media’s integrity teams to improve misinformation algorithmic detection tools.
Introduction
While online misinformation is a crucial issue for societies to address the growing distrust citizens express against their institutions, current research falls under three limitations: First, it is largely based on US data, providing a biased view of the phenomenon, as if misinformation were the same in every country. Second, it rarely analyses misinformation content itself (social media posts) but databases annotated by fact-checkers. Third, it approaches misinformation only from the perspective of fact-checkers with little attention to how social media users perceive it.
This paper suggests an original approach to fill these gaps: leveraging mixed methods to examine misinformation from the reporters’ perspective, both at the content level and comparatively across regions and platforms.
The authors present the first research typology to classify user reports, i.e. social media posts reported by users as ‘false news’, and show that misinformation varies in volume, type, and manipulative technique across countries and social media. They identify six manipulative techniques used to convey misinformation and four profiles of ‘reporters’, as social media users reporting online content to platform moderators for distinct purposes. This allows the author to explain 55% of inaccuracy in misinformation reporting, suggest ways to reduce it and present an algorithmic model capable of classifying user reports to improve misinformation detection tools.
Key Insights
- A reporter-oriented approach to studying misinformation at the content level
The paper presents the first research methodology to classify user reports, resulting from the human review of a dataset of 8,975 content items reported on Facebook and Instagram in France, the UK, and the US in June 2020.
- Country and platform specificities of content reported as misinformation
The analysis of the dataset reveals three main findings:
- Six manipulation techniques to frame and disseminate misinformation online are observed, including a novel one which seems to have emerged on Instagram in the US: ‘the excuse of casualness’. This new manipulation technique presents significantly more challenges for algorithmic detection and human moderation, therefore confirming the importance of better filtering of user reports to improve the accuracy of misinformation detection models.
- Meaningful distinctions are observed, suggesting that the volume of online misinformation content greatly varies between countries (France, the UK, the US) and platforms (Facebook, Instagram), together with the manipulative technique employed to disseminate it. For example, several signals suggest that the circulation of misinformation was significantly smaller in both volume and severity in France than in the US, with the UK occupying an intermediate position.
- A possible convergence in misinformation content between platforms is revealed by a body of corroborating evidence. The great uniformity between content reported by Facebook and Instagram users in the US contrasts with the dissimilarity of content reported by Facebook and Instagram users in France. This suggests a convergence between content on the two platforms and confirms that misinformation is not only a Facebook problem anymore, but also a serious issue for Instagram.
- A plurality of reporters’ profiles allows various leverages for noise reduction
The authors identify four reporter profiles and associate these with distinct reasons for social media users to report content to moderators:
- Reporting false news to trigger moderators’ actions
- Reporting to annoy the content creator
- Reporting inappropriate content that is not misinformation
- Reporting to draw the moderators’ attention to a problematic situation
This allows them to break down the inaccuracy in misinformation reporting into four categories of ‘noise’ and suggest specific means of action to reduce these. While only 7% of content reported by users in the dataset was labeled as ‘false news’ by fact-checkers, suggesting 93% of inaccuracy in user reporting, the authors show that 55% of such inaccuracy should probably not be attributed to the reporters’ lack of seriousness, as it was believed until now, but rather to confusion surrounding the reporting features and moderating rules.
- Leveraging multi-signal reporting to improve misinformation detection
Finally, the authors demonstrate the performance of a gradient-boosting classification model trained on a combination of user reports when identifying the different types of noise. The aim of this model is not to detect the type of content that fact-checkers would rate false news, but rather to identify the most relevant reports to be sent to fact-checkers and to redirect misreported items to more suitable moderators.
In contrast with highly sophisticated detection models trained on massive datasets and leveraging many input signals, the value of this model is its capacity to achieve a promising performance despite its extremely simple architecture, its training on a very small dataset, and its processing of only 10 basic input human signals associated with the categories users employ to report a content piece. While state-of-the-art misinformation classifiers leverage multimodal architectures to grasp the meaning of a post in relation to its semantic and pictorial components, this model is blind to the content itself and ignores personal information about both the content creator and the reporter, as well as contextual information about the content’s sharing and reporting.
Between the lines
By studying misinformation from the reporters’ perspective and at the content level, the authors’ goal was to refute the idea that user reports are a low-accuracy signal poorly suitable for online misinformation detection. They demonstrate that user reporting is a complex signal composed of different types of feedback that should be understood and assessed separately. When approached as such, reporting offers a valuable signal not only for enhancing the performance of false news detection algorithms but also for identifying emerging trends in misinformation practices.
This approach paves the way for more participative moderation frameworks that reward the best reporters by prioritizing their feedback over those of adversarial actors. The meaningful variations in the volume, type, topic, and manipulation technique of misinformation observed between countries and platforms also support the claim that misinformation is anything but a globally uniform phenomenon. Instead of generalizing the findings of US-centered studies, researchers, industry players, and policymakers should examine misinformation with respect to the specificities of each country and platform.