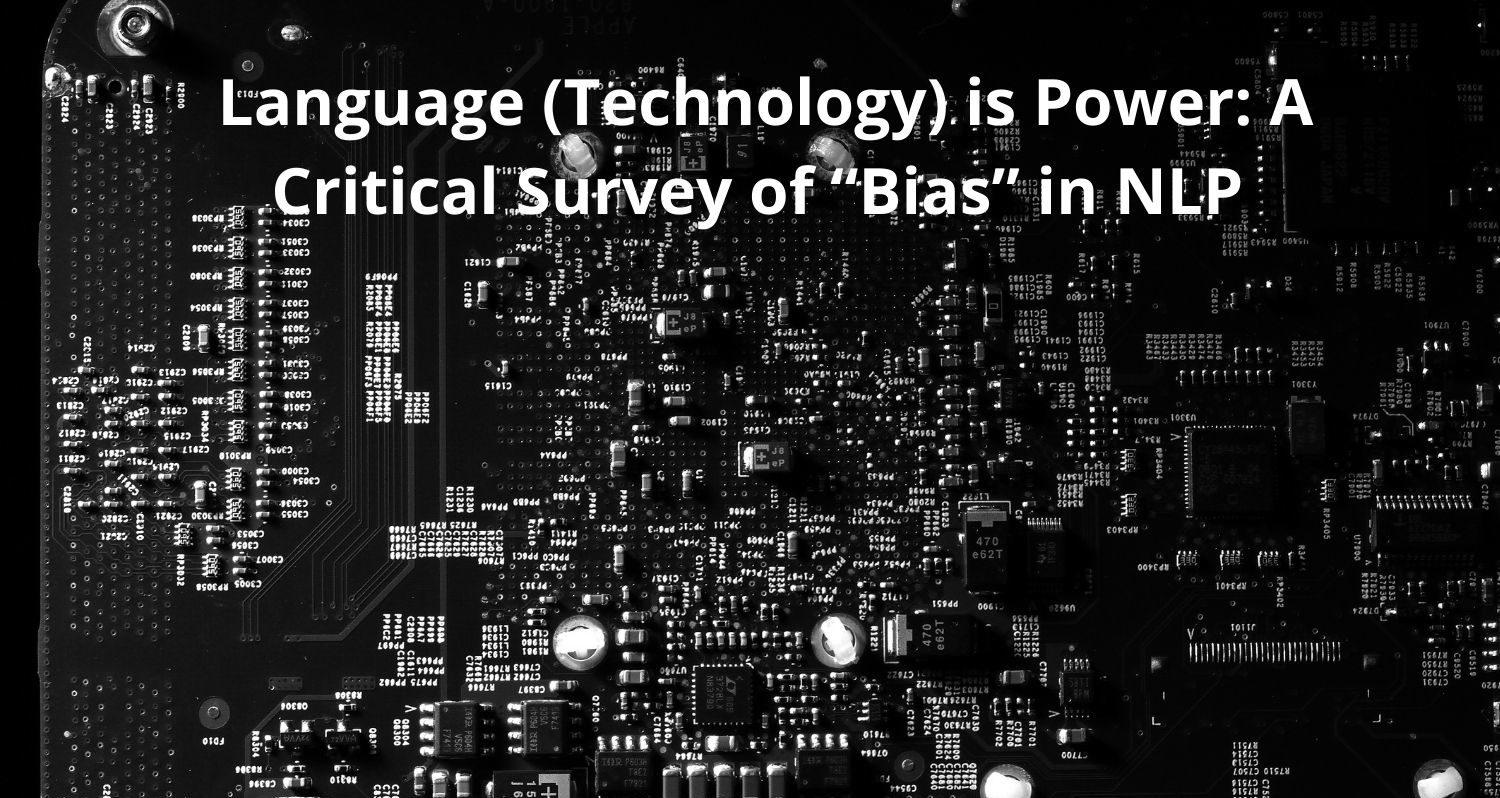
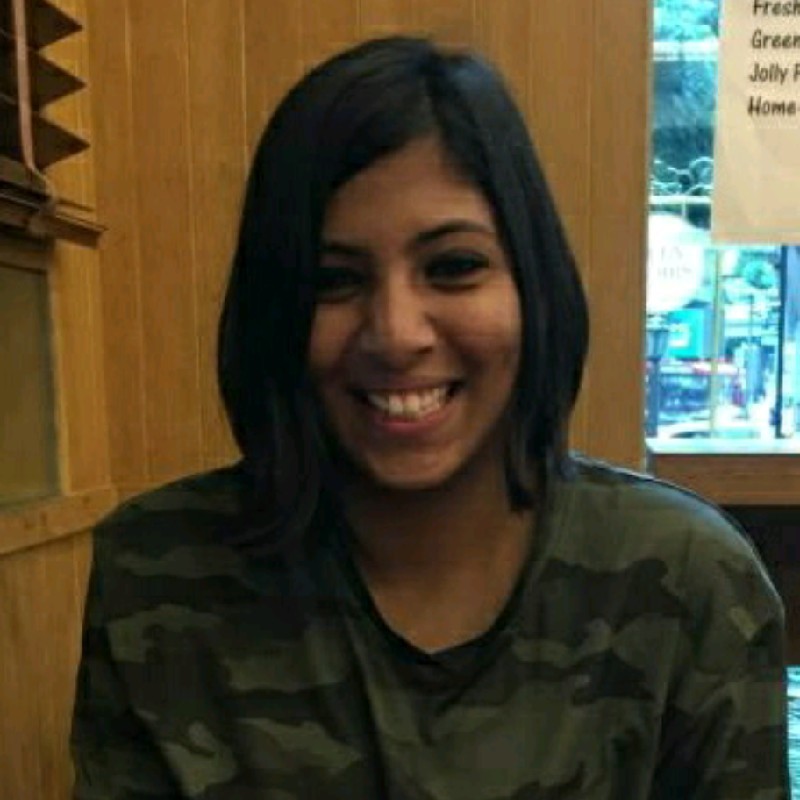
Summary contributed by Falaah Arif Khan, our Artist in Residence. She creates art exploring tech, including comics related to AI.
Link to original paper + authors at the bottom.
Mini-summary: With the recent boom in scholarship on Fairness and Bias in Machine Learning, several competing notions of bias and different approaches to mitigate their impact have emerged. This incisive meta-review from Blodgett et al dissects 146 papers on Bias in Natural Language Processing (NLP) and identifies critical discrepancies in motivation, normative reasoning and suggested approaches. Key findings from this study include mismatched motivations and interventions, a lack of engagement with relevant literature outside of NLP and overlooking the underlying power dynamics that inform language.
Full summary:
The authors ground their analysis in the recognition that social hierarchies and power dynamics deeply influence language. With this in mind, they make the following recommendations for future scholarship on Bias in NLP- They implore researchers to engage with relevant literature outside of the technical NLP community, in order to better motivate a deeper, richer formalization of “bias”- it’s sources, why it is harmful, in what ways and to whom. They also underline the importance of engaging with communities who are most affected by NLP systems and to take into account their lived experiences.
Their critical survey on recent scholarship demonstrates that perspectives that reconcile language and social dynamics are currently lacking. They find that most papers contain poorly motivated studies that leave unstated what algorithmic discrimination even entails or how it contributes to social injustice. This is further exacerbated by papers that omit normative reasoning and instead focus entirely on system performance. When motivations are enumerated in papers, they often remain brief and overlook an exposition on what type of model behaviors are deemed as harmful or ‘biased’, in what ways do these behaviors cause harm and to whom do they inflict harm. In the absence of a strong, well-articulated motivation for studying bias in NLP, papers on the same task end up operating with different notions of “bias” and hence take different approaches to mitigating this “bias”.
With opposing notions of “bias”, scholars tend to treat “bias” that is inherently representational (the model represents certain social groups less favorably than others) as allocational (discriminatory allocation of resources to different groups) and so authors tend to incorrectly treat representational norms as problematic only due to the fact that they can affect downstream applications that result in allocations.
In terms of shortcomings of techniques used to study “bias” in NLP, the paper identifies a lack of engagement with relevant literature outside of NLP, a mismatch between motivation and technique, and a narrow focus on the sources of bias.
With these limitations of existing scholarship in mind, the authors propose a fundamental reorientation of scholarship on analysing ‘bias’ in NLP towards the question: How are social hierarchies, language ideologies and NLP systems co-produced? Language is a tool for wielding power and language technologies play a critical role in maintaining power dynamics and enforcing social hierarchies. These dynamics influence every stage of the technological lifecycle and hence scholarship focused only on algorithmic interventions will prove to be inadequate.
The authors also validate their recommendations using a case study on African-American English (AAE). They explain how models such as toxicity detectors that perform extremely poorly on AAE perpetuate social stigmatization of AAE speakers. The case study drives home the authors’ point that analysis of ‘bias’ in such a context cannot be limited to merely algorithmic analyses, without taking into account the underlying systemic and structural inequalities.
The authors conclude with an open call to the scientific community, reiterating the need to unite scholarship on language with scholarship on social and power hierarchies.
Original paper by Su Lin Blodgett, Solon Barocas, Hal Daumé III, Hanna Wallach: https://www.aclweb.org/anthology/2020.acl-main.485.pdf