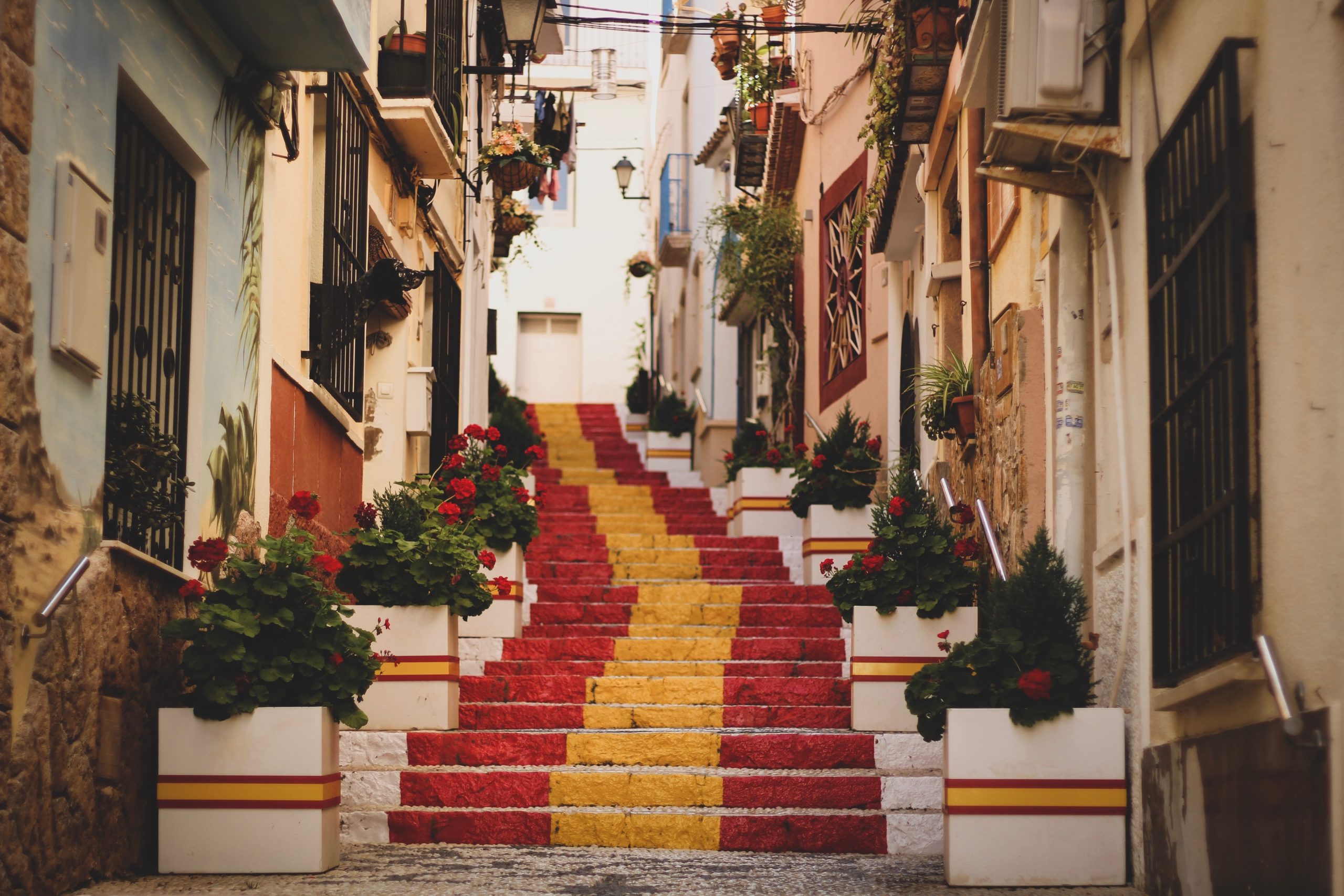
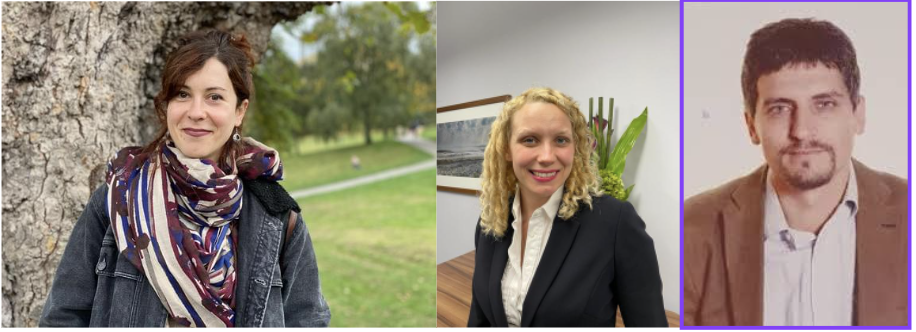
🔬 Research Summary by Ana Valdivia, Cari Hyde-Vaamonde, Julián García-Marcos.
Ana Valdivia is a Research Associate in Artificial Intelligence at King’s College London (Department of War Studies – ERC Security Flows). Her research has explored a critical perspective towards algorithmic systems and the design of ethical, transparent and fair machine learning classifiers.
Cari Hyde-Vaamonde is an experienced lawyer and court advocate, having practised in diverse fields including technology. Turning her focus to research in the field recently culminated in a UKRI 4-year award to research the impacts of AI in justice settings at King’s College London, where she is also a Visiting Lecturer.
Julián García-Marcos graduated in Law, entered the judicial career in 2008. Investigating Judge in Irun and Donostia-San Sebastian until 2021. Nowadays, Magistrate of the 1st section (Criminal) of the Provincial Court of Guipúzcoa (Spain).
[Original paper by Ana Valdivia, Cari Hyde-Vaamonde, Julián García-Marcos]
Overview: Algorithms designed for use by the police have been introduced in courtrooms to assist the decision-making process of judges in the context of gender-based violence. This paper examines a risk assessment tool implemented in the Basque country (Spain) from a technical and legal perspective and identifies its risks, harms and limitations.
Introduction
In 2018, M reported her ex-husband to police authorities in the Basque Country (Spain). She had suffered gender-based violence. During the report, the police authority asked her several questions: ‘Is the male aggressor or victim an immigrant?’, ‘Very intense jealousy or
controlling behaviours?’, ‘What is your perception of danger of death in the past month?’. After this interview, the police used a risk assessment tool that evaluates the severity of the case. In the case of M, the algorithmic output assessed that her husband had a low-risk of further gender-based violence. The report of M’s case together with the algorithmic evaluation was sent to the courtroom. However, the judge assessed that M was at high-risk, contradicting the result of the algorithm. In this paper, we propose an interdisciplinary analysis to examine the impact of this risk assessment tool in this context. Through an exhaustive analysis of publicly available documents and a conversation with a judge, who is in turn a user of this tool, we unveil risks, benefits and limitations of using these algorithmic tools for assessing cases of gender-based violence in courtrooms.
Key Insights
Risk assessment tools to predict violence
The use of algorithms such as risk assessment tools to predict violence has a long-standing history. Statistical predictions which involve predicting an individual’s violent behaviour on the basis of how others have acted in similar situations began in the eighties through the analysis of risk factors. To predict violence, scholars have proposed several statistical strategies to overtake human judgment, and do it better, perhaps citing greater efficiency. Yet statistical outputs do not always outperform human judgements. Recently, some limitations regarding the use of these tools have been the focus of the field of fairness in machine learning and critical data studies. Part of this he scholarship relates to the demystification of the neutrality and objectivity of algorithmic and statistical tools, the unintended discrimination and disparate impact of these tools due to statistical bias and the influence that algorithmic tools have on human decisions.
The intimate partner femicide and severe violence assessment tool: the EPV
In the Basque Country (Spain), police officers are using an algorithm to automatically assess the risk of gender-based violence. This risk assessment tool, the EPV, is based on 20 items evaluating several aspects of aggressors and victims to classify the risks of gender-based
violence recidivism. It was developed by a research team made of clinical psychologists who used their expertise to ‘propose a brief, easy-to use scale [tool] that is practical for use by the police, social workers, forensic psychologists, and judges in their decision-making process’.
The efficacy of this risk assessment tool was assessed through an analysis of the trade-off between the true positives (TP) and true negatives (TN). However, in this context, TP don’t have the same importance than TN: while an error in a negative case (FP) implies that the case is overestimated (more protection to the women), an error in a positive case (FN) implies that the case is underestimated putting in risk a women that suffers gender-based violence. It is then preferable to obtain higher rates of FP than FN, which implies that in the worst case scenario, cases with a non-severe risk of violence are categorised as high risk, implying perhaps greater attention.
Is judicial reasoning aided by EPV-R?
This paper considers real examples of judicial decision-making behaviour. A judge will hear representations of lawyers, documentary evidence, and sometimes oral evidence from witnesses, the accuser and the defendant. At the end of the hearing, on deciding what measures to take, the individual in the judicial role is also presented with evidence of the EPV-R score, suggesting the risk level of the defendant. This score is presented without a narrative. It is left to the judge to decide how to weigh this score, while the score itself is impossible to interrogate at court, either factually, or technically. It may contrast strongly with their own judgment. How this conflict is resolved will vary according to the individual judge, but will have serious consequences for the parties to the case. The impact of errors, and concerns regarding the balance of false positives to false negatives is rarely aired, if ever, and yet crucial for reasoning.
Technical and legal risk and harms of the EPV-R
From a technical perspective, current debates on risks, harms and limitations of socio-technical systems have been focusing on bias and the disparate impact that algorithms might have on different demographic groups, inspired by several publications and journalistic investigations. However, we seek to move the analysis beyond the critique on bias by examining three factors: (1) opaque implementation, (2) efficiency’s paradox and (3) feedback loop.
From a legal perspective, there is a lack of appropriate legal guidelines for use in a court scenario. In the case of EPV-R, we are told by a judge, a first-hand user of this information, that no warning regarding the reliability of the data is given. The legal framework requires real deliberation by the judge, but the status of the algorithm as a quasi-expert closes down enquiry. Principles of the rule of law and due process require that individuals are aware of the case against them, and for the individual in the case, the right of free movement may be restricted. Equally, a victim’s word may be doubted on the basis of a score of “low-risk”.
Between the lines
This risk assessment tool goes to the very core of the judge’s function. If we do not accept that EPV-R is the best overall measure of risk under the legal framework, a judge must then weigh its assessment against their own judgment, based on the facts of the case. To do so they must consider how reliable the EPV-R assessment is (as compared to the other evidence), and what ‘risk’ means in this tool. Yet, at present, the judge does not have the proper means to do this. This paper has been prepared by authors of diverse disciplines (law and computer science) to highlight a practice that has widely gone unreported, was not fully anticipated by the designers of the initial software, and its use is so far unsupported by empirical research. In fact, we identify several elements that make the use of this algorithm in the decision-making of judges not recommended. In considering further steps, we also recommend reviewing the work of Costanza-Chock on Design Justice; D’Ignazio and Klein and Peña and Varon on Data Feminism. Bringing together many perspectives on risk assessment tools in the context of gender-based violence will lead us to build better algorithms, promoting technologies and practices that have real impact in the algorithmic social justice context.