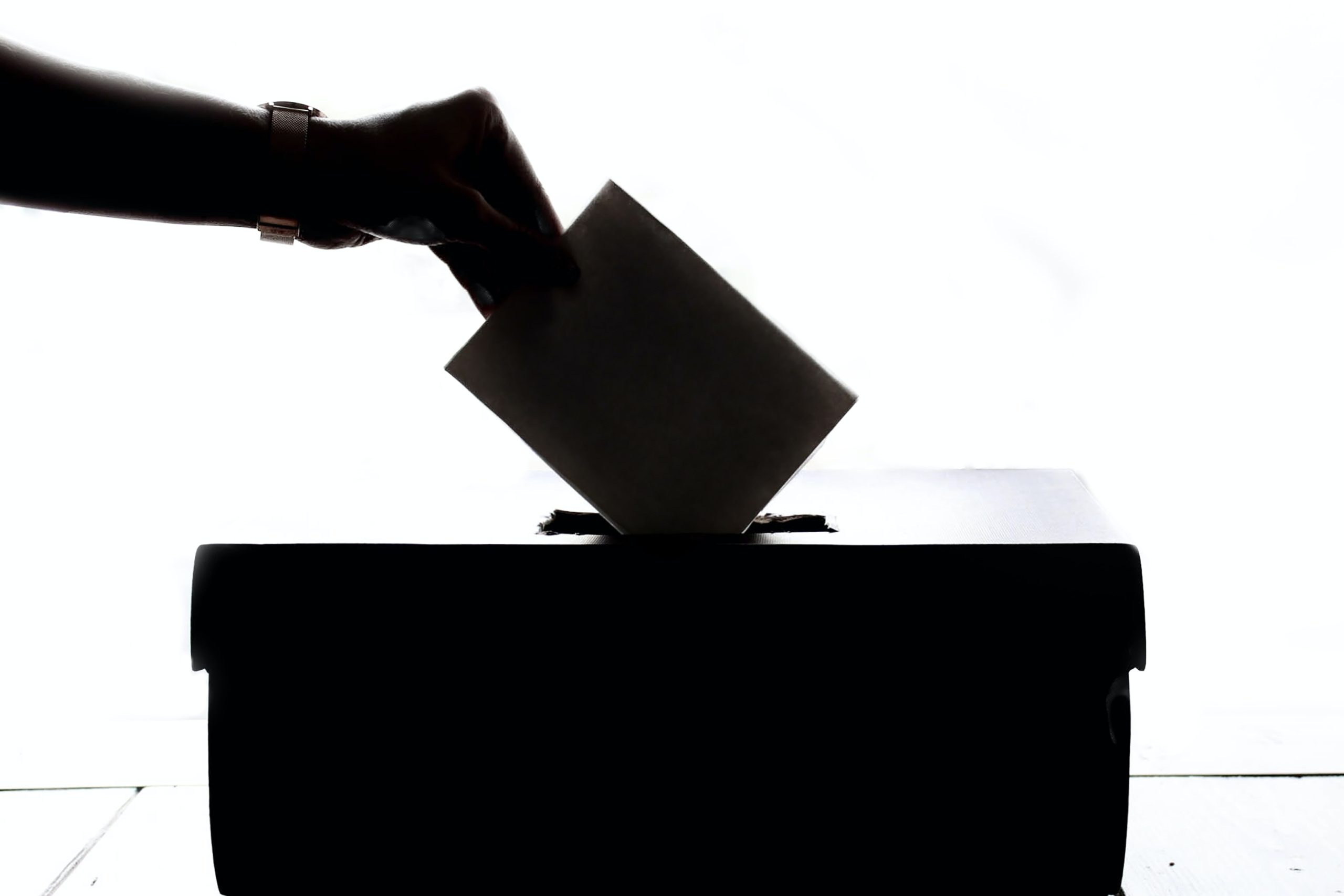
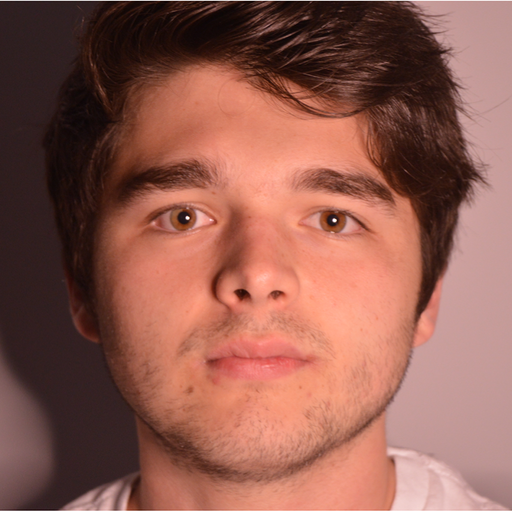
🔬 Research summary by Connor Wright, our Partnerships Manager.
[Original paper by Raphael Koster, Balaguer Jan, Andrea Tacchetti, Ari Weinstein, Tina Zhu, Oliver Hauser, Duncan Williams, Lucy Campbell-Gillingham, Phoebe Thacker, Matthew Botvinick and Christopher Summerfield]
Overview: An under-explored area in AI is how it can help humans design thriving societies. Hence, could an AI design an appropriate economic mechanism to help achieve a democratic goal? This research says yes.
Introduction
An under-explored area in AI is how it can help humans design thriving societies. To test whether an AI system could achieve this democratic goal, the authors designed the Human Centred Redistribution Mechanism (HCRM) to develop an economic mechanism preferred by incentivised humans. Testing this involved four different experiments. Hence, I’ll cover each experiment before diving into the takeaways from the research. I’ll conclude that while this is a victory for AI, the human will remain in-the-loop.
Key Insights
A significant source of inspiration for the research was trying to provide an answer to the value-alignment problem. Given the plurality of human views in society, aligning AI with given human values is tough and almost bound to be discriminatory on some level. Hence, the designers didn’t input any values they believe the AI should aim for to avoid bias. Instead, they set the goal of democracy, arriving at a mechanism that most participants prefer.
The designers wanted to test the consensus on how rewards should be distributed when people collaborate. Here, they designed four experiments based on a wealth distribution game where participants were faced with constant decisions to redistribute or not their monetary endowment.
Consisting of 10 rounds, each player contributes a portion of their endowment to the public pot. This pot is paid back to players depending on the distribution mechanisms employed. Alongside traditional methods, the AI-designed HCRM utilised reinforcement learning to distribute the endowment according to wealth equality and inequality. These 10 rounds were undertaken in the following four variation experiments.
Experiment one
Three distribution mechanisms were trialled: strict egalitarian, liberal, liberal egalitarian. The participants were split into groups of four, with a head player receiving 10 coins each round, while tail players either received 2, 4 or 10 coins. Hence, tail players were either unequal compared to the head players, or equal when they received 10 coins. The participants played 10 rounds, each with the same coin distribution but a different distribution mechanism determining the payout from the public pot.
The strict egalitarian method divided the fund equally among all the players. This allowed certain players to free-ride on the generous contributions to the pot that others made, as everyone got the same. Elsewhere, the libertarian mechanism distributed the pot based on players’ contributions, encouraging them to contribute more.
The liberal egalitarian method operated differently. Instead of simply how many coins were given, it appealed to the fraction of the endowment paid into the pot. This quickly incentivised tail players to contribute. However, this disincentivised head players to contribute, leading to a low public pot.
With these three methods presenting problematic outcomes (unfair distribution and disencentives), the researchers asked whether an AI system could do better. Here, they introduced the HCRM in experiment two.
Experiment two
The HCRM was pitted against the three traditional distribution methods over 10 rounds, containing two rival mechanisms in each one. Participants voted for which distribution method they preferred and played with that strategy in the final round. HCRM was found to be more popular than any other baseline distribution mechanism. However, while preferred overall, in conditions of highest inequality liberal egalitarianism was voted to offer a plausible alternative to HCRM.
Experiment three
A new radical mechanism was introduced to compete with HCRM. Yet, HCRM was still preferred by participants.
Experiment four
Some former players were invited back and trained to design a distribution method to compete with HCRM. However, having designed the mechanism and put it to the test, HCRM was still the preferred choice.
Takeaways from the experiment
An AI system can be designed to complete a democratic objective. However, such a mechanism may inherit the problem of ‘tyranny of the majority’ found within other democratic systems, emphasising already existing biases.
As a result, privacy and explainability become fundamental principles to uphold to mitigate any issues. The HCRM was not equipped with active memory, meaning it could be explained easily (it does not include a complicated choice history of a particular player), as well as more private (meaning players couldn’t be identified through their choices).
Between the lines
At the end of the paper, the authors clarify they are not advocating for an all-AI and automated government. In my view, it’s essential to keep ourselves grounded and acknowledge what the journey ahead is rather than immediately get carried away with any AI victory. For example, this article shows how autonomous vehicles are still years away, despite the strides made. Hence, while AI systems can achieve democratic goals, it must be acknowledged that the human will stay firmly in-the-loop for now.