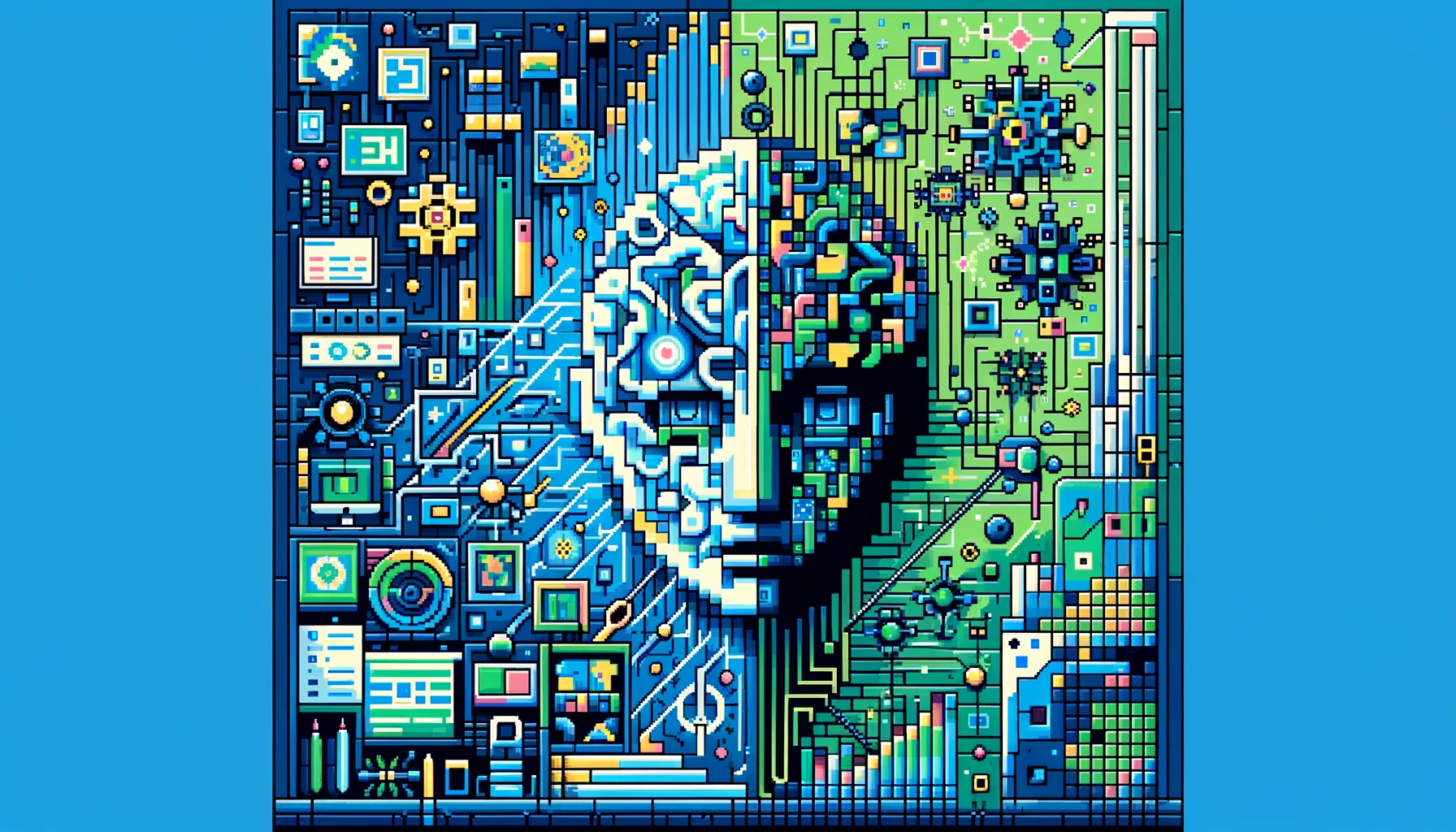
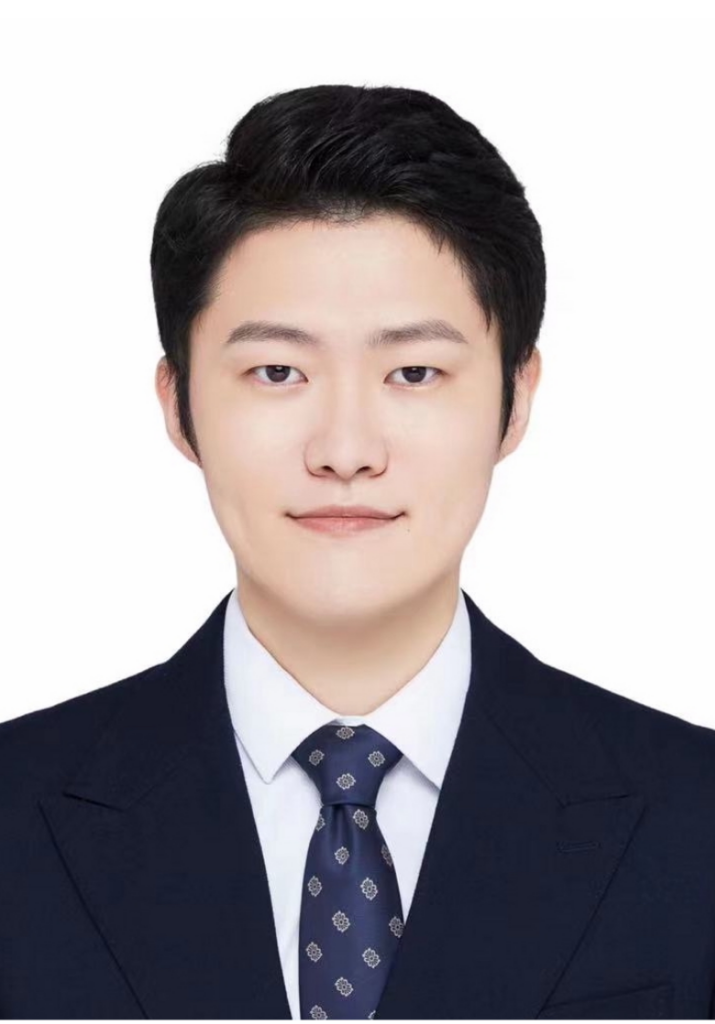
🔬 Research Summary by Jian Gao, a Ph.D. candidate in operations management at UCLA Anderson School of Management, focusing on the interactions between humans and autonomous technologies.
[Original paper by Francisco Castro, Jian Gao, and Sébastien Martin]
Overview: People can use generative artificial intelligence (AI) to become more productive, but the AI-generated content may not match their preferences exactly. They can obtain more aligned results by editing the content themselves or providing more information to the AI. However, this takes time, and users face a trade-off between output fidelity and communication cost. This paper studies the societal consequences of such human-AI interactions, focusing on the risks of homogenization (everyone’s work becoming more similar) and the potential societal influence of biased AI.
Introduction
Generative artificial intelligence (AI) systems have improved at a rapid pace. For example, ChatGPT recently showcased its advanced capacity to perform complex tasks and human-like behaviors. However, have you noticed that content generated with the help of AI may not be the same as content generated without AI? In particular, the boost in productivity may come at the expense of users’ idiosyncrasies, such as personal style and tastes, preferences we would naturally express without AI. To better align our intentions with AI’s outputs (i.e., output fidelity), we have to spend more time and effort (i.e., communication cost) to edit our prompts or revise the AI-generated output ourselves. But what is the impact of this tradeoff at the individual and aggregate levels?
To study this effect, we propose a Bayesian framework in which rational users decide how much information to share with the AI, facing a trade-off between output fidelity and communication cost. We show that the interplay between these individual-level decisions and AI training may lead to societal challenges. Outputs may become more homogenized, especially when the AI is trained on AI-generated content. And any AI bias may become societal bias. A solution to the homogenization and bias issues is facilitating human-AI interactions, enabling personalized outputs without sacrificing productivity.
Key Insights
An Example
Imagine you are a journalist ready to cover a piece of breaking news. Without the help of generative AI, you will be slow, but the content will be entirely yours, including your specific style, political views, tone, and layout. Now, let’s say you want to use ChatGPT to speed things up. However, ChatGPT might not get your style right unless you give it specific instructions, which can take time. So you have a choice: either quickly get a draft that may not match your taste (i.e., low communication cost but low output fidelity) or take a bit longer to get something closer to your style (i.e., high output fidelity but also high communication cost).
Human-AI Interactions and Homogenization
When solving for each user’s optimal decision, we find that their use of AI depends on how “unique” they are. For instance, if a journalist follows a straightforward, news-reporting style — akin to what you may find in daily newspapers with just-the-facts reporting — they might be satisfied with the first draft AI produces. This way, they save lots of time and effort, even if the output isn’t an exact match to their style. On the other hand, if a journalist has a unique style, such as weaving intricate narratives or employing a satirical tone similar to outlets like ‘The New Yorker’ or ‘The Onion,’ they’d likely spend more time guiding the AI to ensure the article aligns with their distinct voice. In essence, those adhering to common news-reporting styles can quickly adapt the AI’s initial suggestions, while those with more specialized styles would spend extra effort to get the piece just right.
To establish the homogenization effect, we prove that when people use AI to help with tasks, the results tend to be more alike or ‘homogenized’ than if they did it alone. On a broader scale, when everyone uses AI, the results are less diverse than if they work individually. Furthermore, this phenomenon can be exacerbated when this AI-generated content is used to train the next generation of AI: we show that the users’ rational decisions and the AI’s training process can mutually reinforce each other, leading to a homogenization “death spiral.” This is concerning, especially since much of the data used to train tools like ChatGPT directly comes from the Internet. If the web becomes flooded with AI-generated content, we could end up in a world where everything looks and sounds alike.
Human-AI Interactions and AI Bias
We also study the effects of AI bias, identifying who benefits or loses when an AI doesn’t accurately reflect what the general population wants. First, we show that censoring bias—when an AI is censored to exclude unique and extreme styles or views—will hurt the overall population in the long run. This may seem counterintuitive since we might think that mainstream users would benefit from this censorship. Yet, our finding reveals that the benefits for this majority are marginal, while the harm to the minority with unique preferences is substantial, reducing the overall benefit for society.
Then there’s another kind of bias, which we call directional biases. For instance, if an AI is trained on more politically left-leaning (or right-leaning) articles, it might not hugely damage its overall usefulness. Still, it will tilt the balance of articles produced, leading to a societal bias. This means a few people designing the AI have the power to influence what we see and hear. More alarmingly, even if we know an AI has a particular bias, many may still use it to save time and effort. On the positive side, when users actively interact and guide the AI, it can help offset some of these biases. This underscores why it’s important to remember human behavior when discussing the impact of generative AI.
Mitigation of the Issues
We show that tasks that are either hard to do without AI (e.g., image generation) or for which speed is particularly important (e.g., grammar correction) are especially sensitive to the risks of homogenization and bias. However, there’s hope. Our research also demonstrates that facilitating human-AI interactions by providing users with ways to better express their preferences to the AI can help preserve population diversity and limit the risk of AI bias. For example, Open AI has experimented with custom instructions and voice recognition in ChatGPT, which can make the tool more user-friendly and adaptable.
Between the lines
Understanding the general effects of user behaviors while interfacing with an AI remains an open question that is difficult to study empirically. We hope our analytical approach highlights the importance of adopting a human-centric perspective rather than solely focusing on AI technology. Indeed, while AIs could surpass human abilities in various aspects, their impact largely depends on how we employ them. The interaction with AIs could offer a novel way to produce and create. Still, it also introduces an extra risk: AIs may filter and even replace our original preferences, styles, and tastes, thereby leading to a world influenced by the content used to train the AI— potentially leading to homogenized and biased content. Improving human-AI interactions and encouraging users to authentically voice their unique views is crucial to avoid these societal pitfalls.