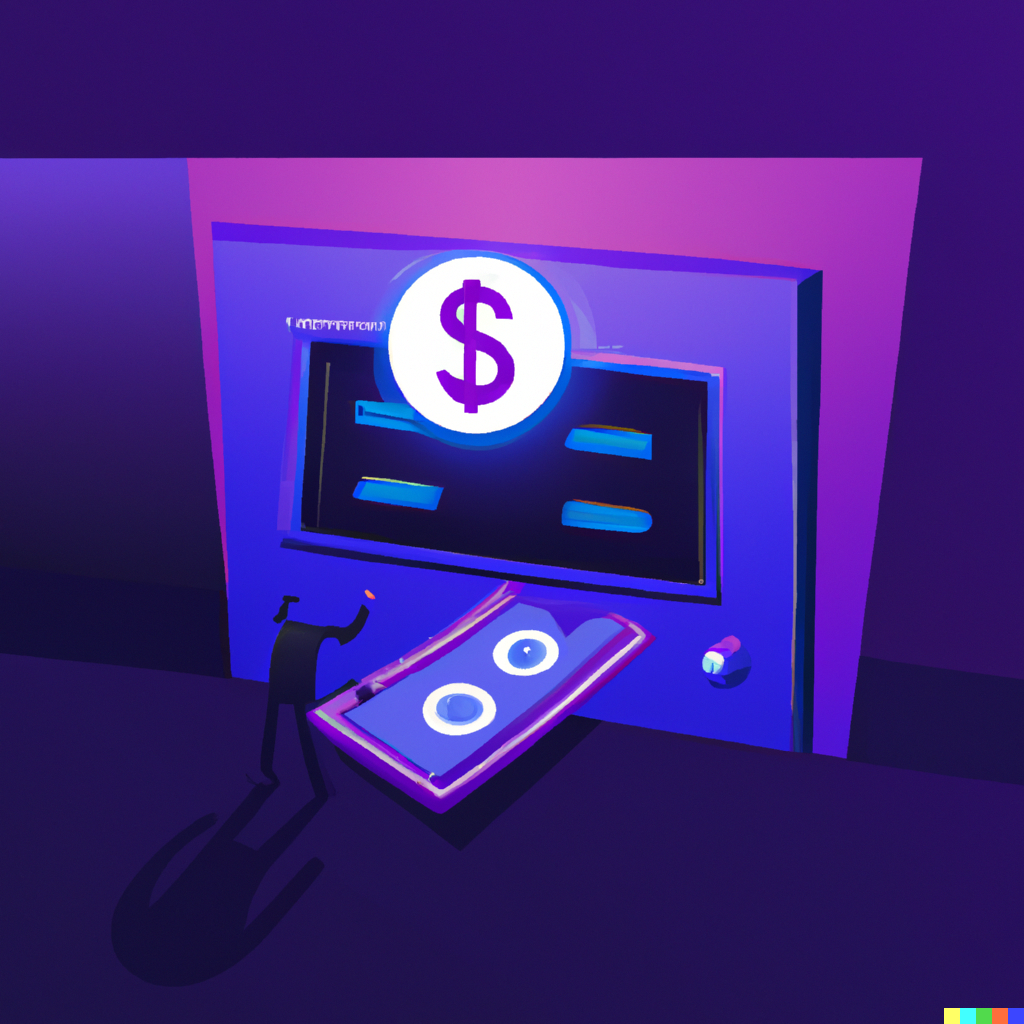
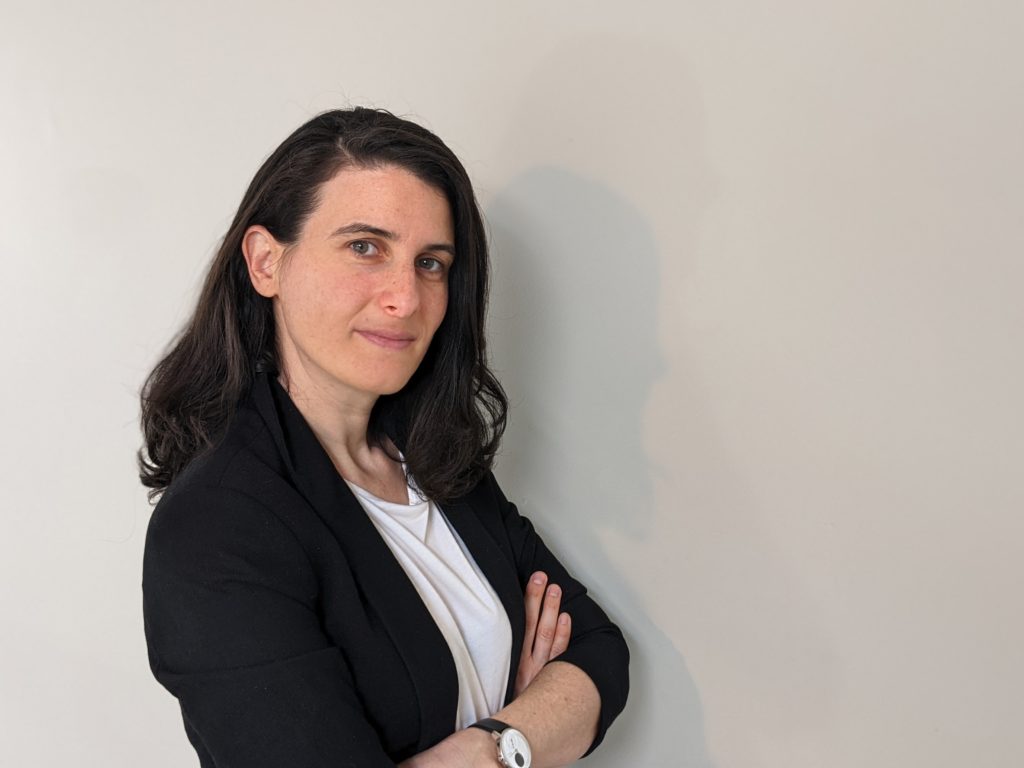
✍️ Original article by Ravit Dotan, a researcher in AI ethics, impact investing, philosophy of science, feminist philosophy, and their intersections.
People who invest in data and AI companies should care about AI ethics because it improves their financial performance and social impact. In this article, I recommend that investors use the workflow below as part of their due diligence. In what follows, I describe each step of this workflow, including presenting a sample scorecard and discussing potential next steps for investors.
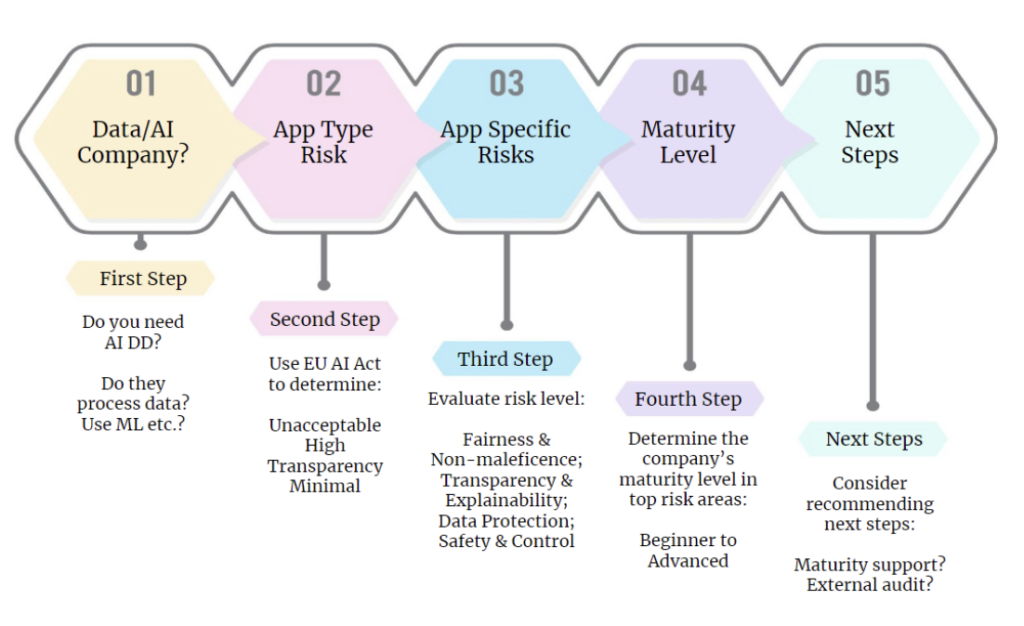
1. Decide whether due diligence is needed
Deciding whether a company is an AI company can be difficult because the term “AI” is over-used and ambiguous. First, we currently lack a universally accepted definition of AI. Second, companies sometimes boast about using AI when they don’t because they think it makes them more attractive. Third, companies that don’t perceive themselves as AI companies may be using AI nonetheless, e.g., in back-office operations. Fourth, some companies that don’t use AI, such as data-heavy companies, still face AI-related risks.
Given these challenges, I recommend an inclusive approach. Investors should consider not only the usage of AI but also the usage of data. An AI due diligence is appropriate when the company processes (or is expected to process) large amounts of data, especially if this data is sensitive or collected from end-users. In addition, AI due diligence is appropriate when the company develops or uses technology that satisfies one of the influential definitions of AI (or is expected to do so).
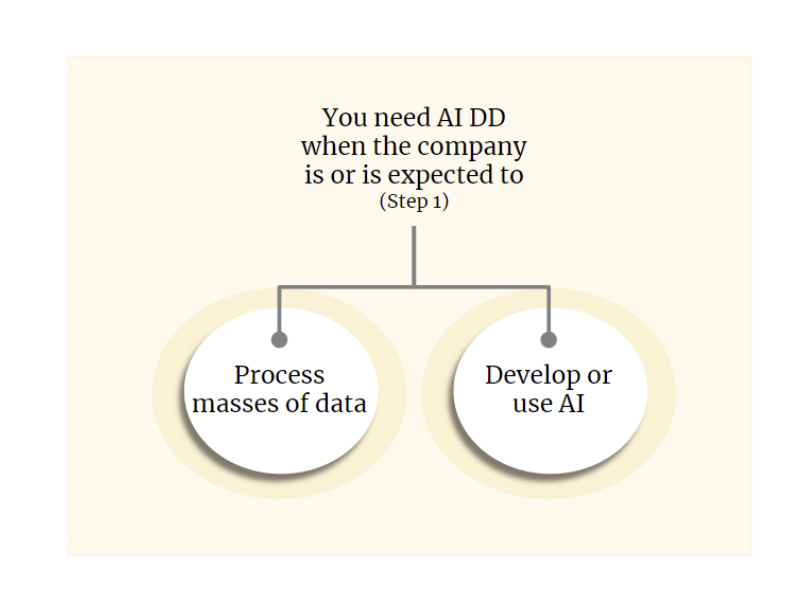
One of the influential AI definitions is given by the draft of the EU AI Act, which is expected to regulate AI in the EU. According to this definition, which is still under deliberation, an AI is software that:
- Can, for a given set of human-defined objectives, generate outputs such as content, predictions, recommendations, or decisions, influencing the environments they interact with
- AND is developed with one or more of the following techniques:
(a) Machine learning approaches, including supervised, unsupervised, and reinforcement learning, using a wide variety of methods, including deep learning
(b) Logic- and knowledge-based approaches, including knowledge representation, inductive (logic) programming, knowledge bases, inference, deductive engines, (symbolic) reasoning, and expert systems
(c) Statistical approaches, Bayesian estimation, search- and optimization methods
2. Application-type Risk
The first step in conducting AI due diligence is understanding the company’s data and AI risks. The draft of EU AI Act can help with that. The proposed bill classifies AI systems into four risk groups: unacceptable, high, transparency obligations, and minimal. Each group will be regulated differently, from prohibition to no regulation. Investors can use this classification for a course-grain evaluation of the company’s AI risk level.
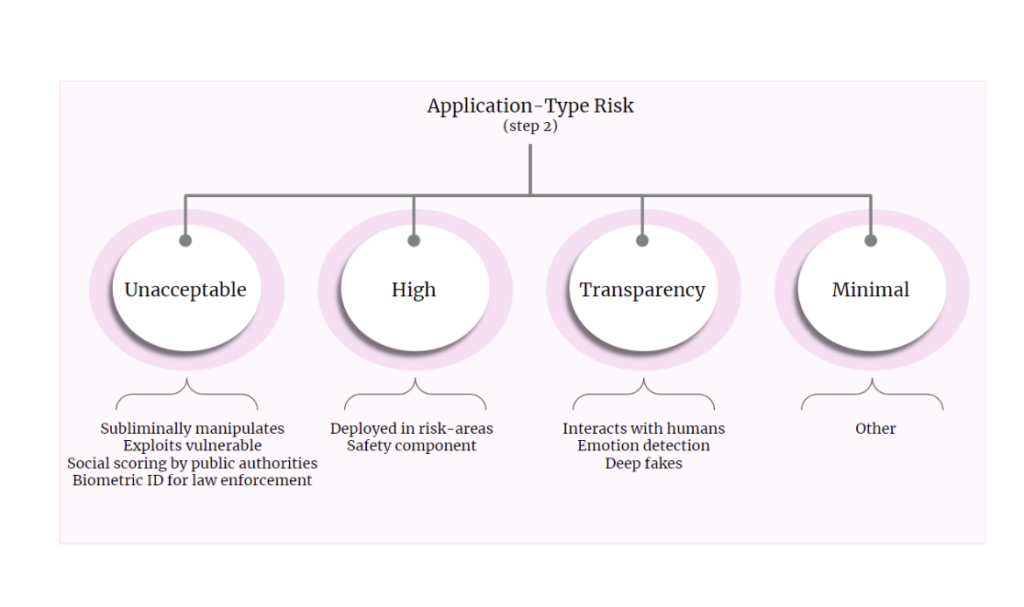
In more detail, the classification is as follows:
- Unacceptable Risk (applications in this class will be prohibited)
- Has a significant potential to manipulate persons subliminally
- Exploits vulnerable groups
- Social scoring for general purposes done by public authorities
- Real-time remote biometric identification systems in publicly accessible spaces for law enforcement
- High Risk (applications in this class will be heavily regulated)
- Used as a safety component in regulated products, such as toys, cars, and medical devices.
- Deployed in high-risk areas, such as law enforcement, biometric identification, and education.
- Applications that require transparency (applications in this class will have transparency obligations)
- Interacts with humans
- Used to detect emotions or determine association with (social) categories based on biometric data
- Generates or manipulates content (‘deepfakes’)
- Minimal – all other AI applications.
- These will not be regulated.
3. Application-specific risks
The EU AI Act’s classification helps evaluate AI risks, but the applications in each class vary greatly. For a more fine-grained evaluation, we can use influential principles from AI ethics, such as fairness and non-harm, transparency and explainability, data protection, and autonomy and human control (this list and the discussion of it below are based on a study led by Jessica Fjeld). Companies that abide by these principles mitigate the risk of negative impacts on people and society. Ignoring or violating these principles creates risks of negative impacts.
The potential consequences of ignoring or violating a given principle depend on the company’s actions. Therefore, to understand the risks, we need to consider the company’s activities in light of each principle.
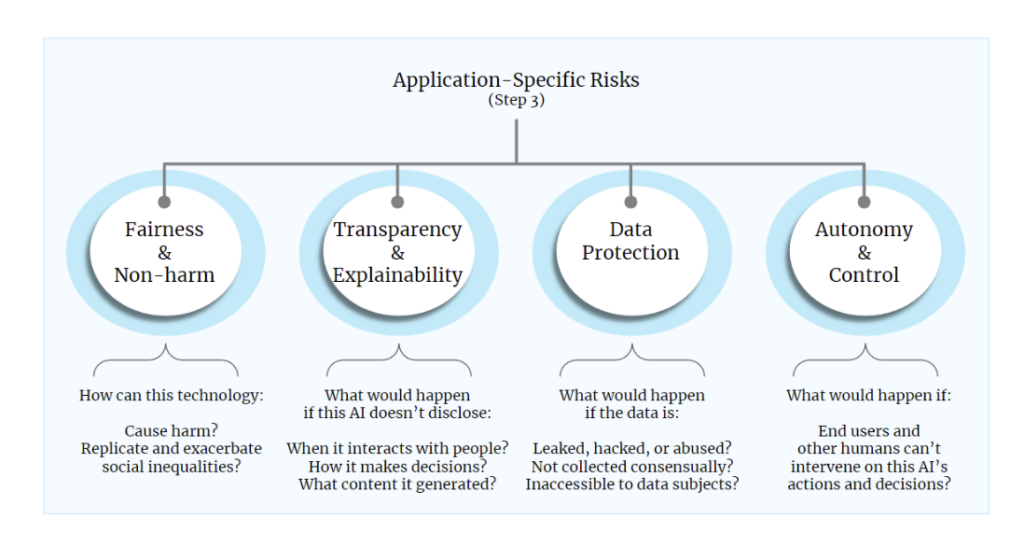
Fairness and non-harm (usually called “non-maleficence”) are about avoiding harm and avoiding replicating and exacerbating social inequalities. AI can conflict with fairness ideals when it wrongfully discriminates, which may happen when datasets and design choices are biased and when the AI’s outputs impact minority groups differently. To evaluate fairness and non-harm risks, you can ask questions such as: How can this technology cause harm? How can it replicate and exacerbate social inequalities? The more substantive the potential negative impact, the higher the risk.
Transparency means that the system makes oversight easy. Transparent AI systems notify users when interacting with them, when they make decisions about them, and when an AI system generates an artifact (such as an image or a video). Explainability means that technical concepts and findings are translated into understandable content. For example, explainability ideals require that users understand why the AI made the decisions about them that it did.
To evaluate the risk transparency and explainability risks, you can ask questions such as: What would happen if the AI didn’t notify users when it interacts with them, how it makes decisions about them, or when it generates content? The more substantive the potential negative impacts, the higher the risk.
Data protection includes preventing data leaks and corruption, protecting privacy, asking for consent before collecting and using data, and allowing users to access, revise, and erase their data. To evaluate data protection risks, you can ask questions such as: What would happen if the data is abused? What would happen if data is not collected consensually or if the data is inaccessible to the data subjects? The more substantive the potential negative impacts, the higher the risk.
Autonomy and human control mean that humans should control what the AI does. First, people should be able to intervene in the actions of AI systems. Second, end users should be able to appeal automated decisions about them and refuse to be subject to them. To evaluate autonomy and human control risks, you can ask questions such as: What would happen if end-users and humans cannot intervene in this AI’s decisions and actions? The more substantive the potential negative impacts, the higher the risk.
You can customize this list of principles if you’d like. I explain why it is good to do so and give guidance on how to do it responsibly here.
4. Maturity in Risk Areas
In addition to evaluating the technology’s risks, investors can evaluate the company’s efforts to mitigate those risks. To assess the degree to which the company mitigates data and AI risks, I recommend that investors focus on three pillars of the company’s activities:
- Knowledge – what does the company do to understand AI ethics and its relevance to their business?
- Workflow – what does the company do to integrate AI ethics into its workflows and incentives?
- Oversight – how does the company keep itself accountable for implementing AI ethics?
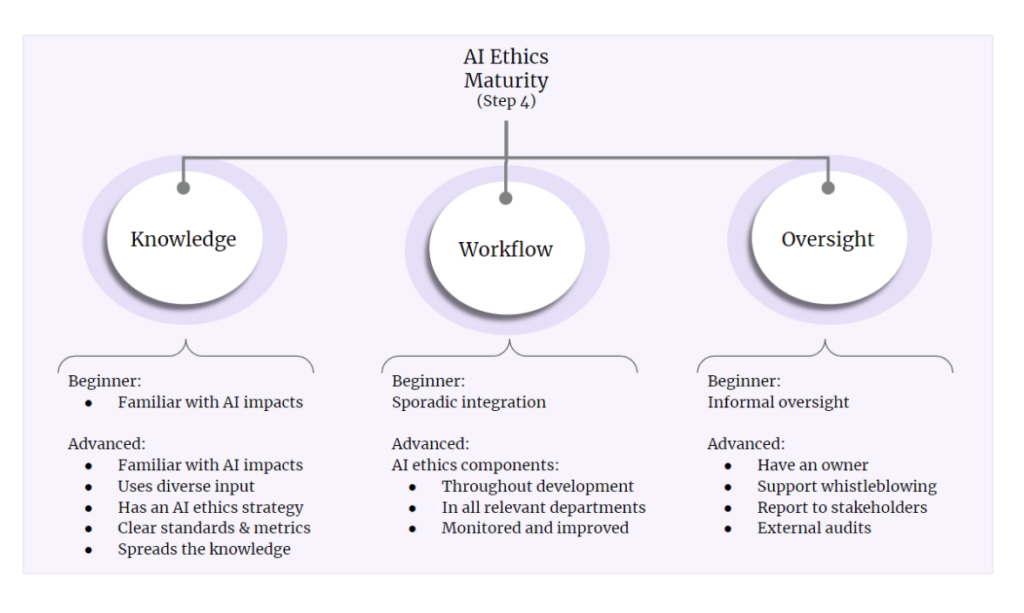
Investors can rank the company’s AI ethics maturity from beginner to advanced in each of the pillars and for each of the risk areas. To do so, investors can use the framework I presented and demonstrated in the two previous articles in the series. In brief, companies that are advanced in AI ethics engage in the activities detailed in the diagram above in ways that are commensurate with their stage of development, from pre-seed to enterprise. Deciding what a satisfactory level of maturity depends on the investor’s risk preferences and the company’s risk level.
Investors may evaluate the companies by talking to their representatives or asking them to complete questionnaires. Either way, thinking carefully about who you want to interact with is essential. For example, you might want to get information from various position holders, including the heads of the relevant departments, to get a sense of how things work on the ground.
5. Next steps
Through the due diligence process described in this article, you can collect information about companies’ data and AI risks and their AI ethics maturity level. You can aggregate this information in a scorecard, e.g.:
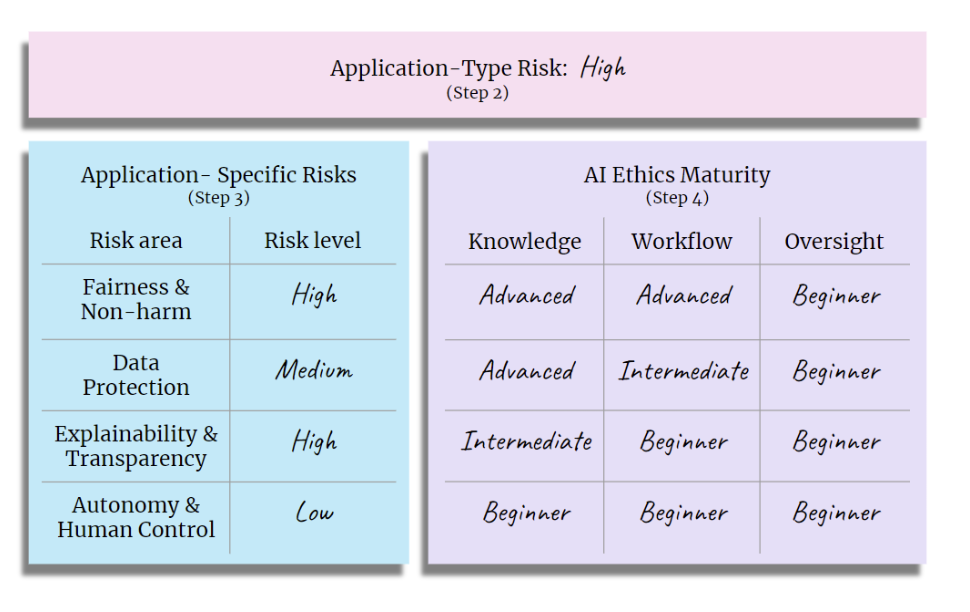
You can use the information obtained in this due diligence to recommend the following steps, including refusing to engage with the company, providing it with AI ethics support, and requiring an external audit:
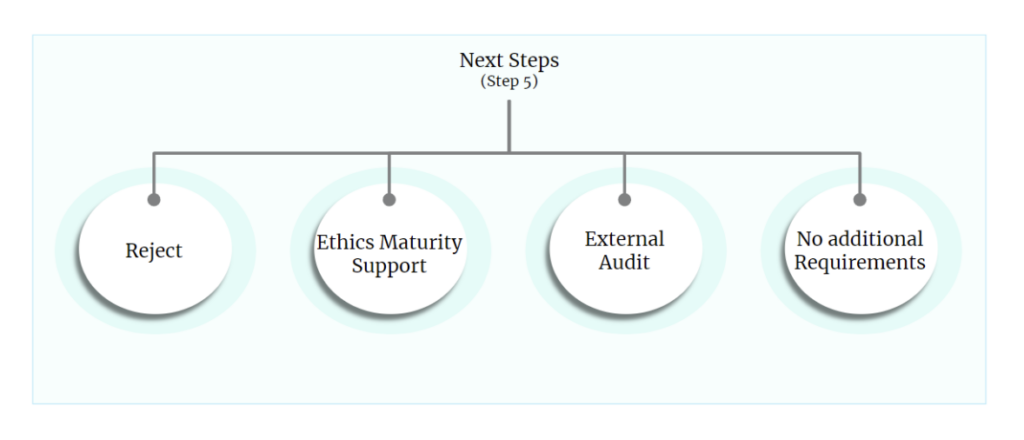
It is helpful to decide on your next steps and policies in advance. For example:
- Reject – do you have non-negotiable red lines? For example, will you engage with a company even if its application-type risk is unacceptable? What is the minimum acceptable AI ethics maturity level for high-risk companies?
- Ethics Maturity Support – in which cases will you recommend that the company works to increase its data and AI ethics maturity? Will you provide that support, e.g., collecting AI ethics progress reports and giving access to relevant services?
- External Audits – in which cases will you require the company to undergo AI ethics external audits?
- No additional requirements – will there ever be circumstances in which you will require or provide nothing else of the company concerning AI ethics? Since AI can be highly unpredictable and companies change with time, I do not recommend this option. It is always best to at least provide AI ethics maturity support.
6. Summary
In the first two articles in this series, I described my approach to implementing AI ethics and a case study. In this article, I explained how investors and buyers of AI companies could use AI ethics to make more informed investment decisions. Feedback is very welcome; feel free to use this form.
Acknowledgments:
The research for this article was supported by the Notre-Dame-IBM Tech Ethics Lab and VentureESG.