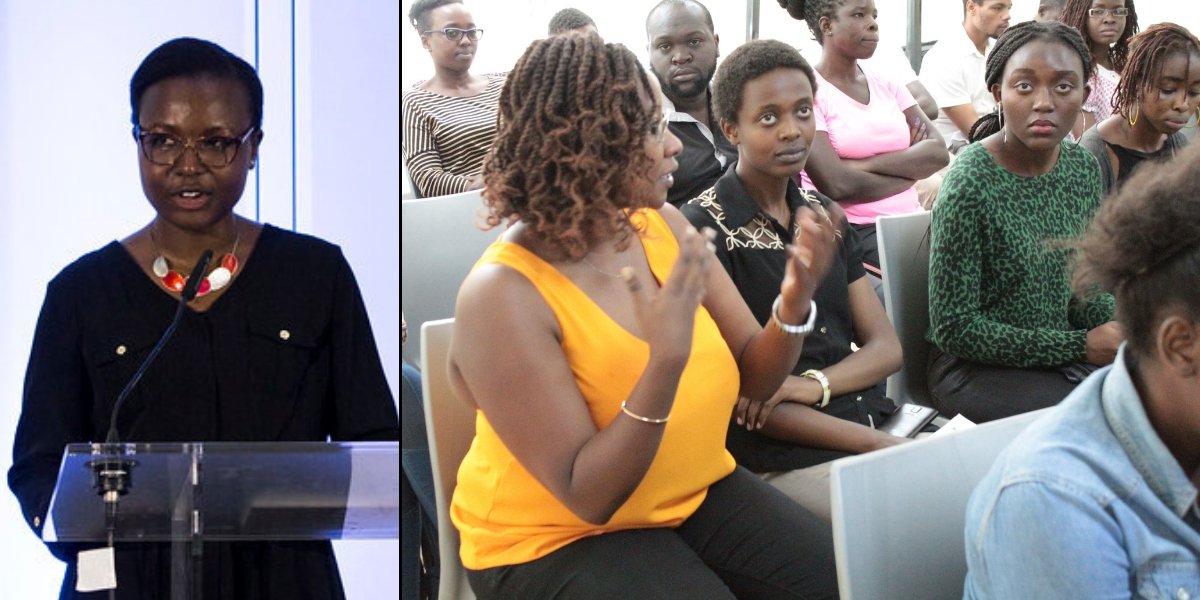
Kathleen Siminyu is a data scientist & machine learning engineer who is Regional Coordinator for the Artificial Intelligence for Development – Africa Network. She is Co-Founder of the Nairobi Women in Machine Learning & Data Science community, and part of the Deep Learning Indaba Steering Committee. Her other interests include natural language processing for African languages and low-cost hardware robotics.
We share this story as a demonstration of how AI can indirectly bring people together and empower communities instead of downgrade, divide, or discriminate against them. We believe that community leaders have an important role to play in defining humanity’s place in a world of algorithms.
Why she thrived in STEM despite the barriers for women
I’ve always been strong in the sciences and it’s something that was encouraged in the household because both my parents are doctors. Also, I’m the only girl in the house and grew up with brothers. Because of that, my mom never thought, “You’re a girl so you’re different” and I always got to do what the boys did. She likes to tell the story of how as a child, she bought me dolls a few times but I’d always say “No I want a car like my big brother!”
Eventually, she stopped trying to impose this idea of “You’re a girl so you should play with dolls” on me, and allowed me to do whatever I wanted even if it was the same thing my brother was doing.
Being raised in this environment where I was allowed to do what the boys did really helped because then my attitude for everything in life became “What do you mean I can’t do that? If the boys can do it, why can’t I? I’ve always been able to do what they do.”
Disempowering situations, and how she overcame them
But my environments didn’t always reinforce that, like when I was studying math and computer science in university. In our class of 55 people, we were 4 girls. Naturally, we gravitated toward each other. When there was a group programming project for our unit on internet applications, we had to do it in groups of 4. We thought we’d do it together – all 4 girls. And the lecturer actually said “No you can’t be in one group – you’re all girls. Normally, girls in this situation write the documentation. So you need to split up and only have one girl per group so that you can have the boys do the work for you.”
There are environments where women are told “No you can’t do that, you need to pair up with someone else — a boy, who can help you.” But I like to code so I just kept coding and here I am. Women are sometimes not as assertive and need an extra push so that they believe they can do it – I see that in the women in our community. We noticed in the beginning that our attendance was largely male even though it’s a community meetup for women. After talking to people, we realized that sometimes the content felt too difficult for them – and that stopped them. For guys, it was difficult too but their rationale was, “This community adds value even though it’s hard, so I’ll keep coming back.”
For women, we asked them why they didn’t come back and the response was, “I could barely follow along last time so I thought let me take a course and then come back a little later when I am better able to follow along.” Telling them that this is exactly what the space is for helped a lot. You don’t need to wait until you’re already an expert – this space is really for you to explore.
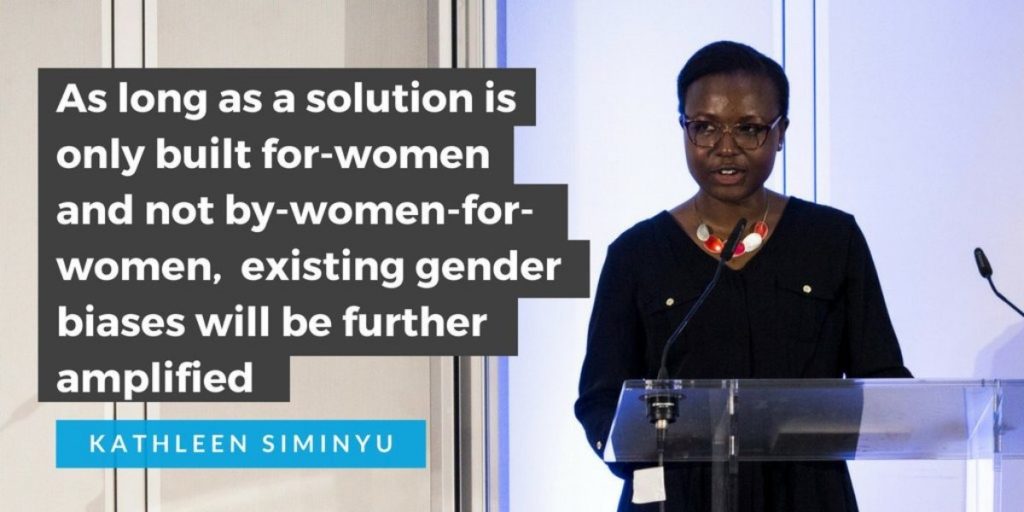
Breaking the computer scientist origin story stereotype
The first time I got my own computer was in my second year of university. Before that, I used my mother’s. My first exposure was in primary school though – we had computers at school. I can’t say that at the beginning I was enamored by computers and knew I wanted to be a computer scientist. That was not my journey at all. I thought I’d become a doctor, so ending up in computer science was very surprising.
I realized medicine wasn’t for me, and I already had the requirements of math and physics so I explored other options, which led me to computer science. I actually only started programming in my third year of university, so it was fairly “late”. It’s never too late to pick it up. Some people start in primary or high school, but I started late and I still turned out okay.
How she got started with building communities and why she continues to do it
I went to a public university in Kenya and the quality of education wasn’t the best – the social aspect is really what kept me going. But in my final years, I realized that after graduation I was expected to earn a living with the skills I’ve earned. Then I naturally thought, “What skills have I learned?” To develop my skills, I got involved with developer communities, which are very vibrant back at home at the universities. I got involved with the Intel Student Partners program, which would get teams of 2 – 3 ambassadors on various university campuses and empower us to carry out events every semester. That’s how I started to write code: by running this community.
The Intel team would organise skills trainings for us as ambassadors and teach us how to use some of their software tools. We were then charged with passing on these skills to other students. And that’s how I first got involved with community building. It’s also what got me my first internship because in my interview one of the things which helped me stand out is that I built a community. A lot of people can write code, but computer scientists who can communicate well are rare.
At my first job as a data scientist, I was very inexperienced. I was not a data scientist, I was a Math and CS graduate — so it was a learning process. I looked to the ways that I had been able to gain skills in the past and that was through community. At the time in Nairobi, there was no such community specifically for individuals in data science and machine learning. Me and a colleague who also happened to be looking for community, we got together and in touch with the wider Women in Machine Learning & Data Science organization, and they said “If you want to do it we can support you and you can start a local chapter there.”
Starting that community was just me looking for ways to improve my skills and find people who could support my learning journey. And since then I’ve been building communities and writing code.
4 lessons learned on building a community
Listen to the community to find out exactly what it needs, as opposed to you deciding what it needs. A lot of times we start up a program and in our heads we think it will work but then we launch it and no one’s interested. If you sit down and listen to people to figure out what they want to get out of this space, you can start to really address the community’s needs. For example, we used to regularly run monthly meetups, and a few people would come and tell us whatever fancy thing they’re doing with AI at work. But then it was hard to ensure retention — guys were not attending consistently. It’s because they wanted to build technical skills and that is hard to do if every time you attend a session, the content is something completely different. That’s when we decided to diversify the range of activities we facilitate. Give them what they want.
Building personal relationships and following up with people has really helped. Because we were only 2 people that ran the community this was hard to do it for everyone, but when you do it for one person that person will check in with another person. That’s a very organic solution for getting women to come back. In Nairobi, a lot of the data science and machine learning events have a really high representation of women and I think that can be directly attributed to the fact that we’ve created this space for women. People often start and think, “I don’t know what this is but I want to get involved.”
Find a way to appreciate volunteers. It’s not an easy job. It’s difficult, can feel thankless, and no one’s getting paid to do it. You could try making it easy for them to come by taking care of their food, transport or giving them some sort of token. You don’t want them to have the experience of “It was so much work and I got nothing out of it.” Making them feel appreciated goes a long way.
Diversify the activities. We just did meetups for a while until we realized that guys wanted more engagement, then we experimented with different things.
- We run quarterly cohorts of courses – we don’t provide the material, we use free online resources and they keep each other accountable by meeting up, and calling each other. It’s a study group basically, and it could be for a book or online course.
- Hackathons work too: working with companies who give us data, and this type of event is for people who have the skills and are looking for a way to use it. The company frames the problem and we get data scientists to work on it. It’s a win-win.
- Now we’re testing open-source projects, and finding a way that people can use the skills that they’ve already gained to build something bigger together – doing away with the siloed efforts and making people feel like a part of something larger.
How she found her calling, and the stories that keep her going in the face of adversity
Everyday I realize that I’m in this position. There’s this quote I love: “As a man’s real power grows and his knowledge widens, ever the way he can follow grows narrower: until at last he chooses nothing, but does only and wholly what he must do…”
If you asked me three years ago if I saw myself building communities outside of school, I wouldn’t have imagined it. I’m not paid to do it so it’s not my job, but I found I’m good at doing it, and it needs to be done. I continue doing it because everyday you hear great stories: I’ve seen women come to this community, do a program for four months, and then at the end of it have the courage to quit their job and look for a data science job…and actually get it!
One time, we had a woman come with her 3 or 4 year old toddler, a little girl, because her help had unexpectedly cancelled for the day. She apologised for the little disruptions the little girl caused but I thought, we really must be doing something right if she thought she would just come with her little girl as opposed to staying home with her. This really is a space for women and stories like these keep me going.
The hard things about running a volunteer organization
This past week, my fellow co-founding organiser quit. I understand where she’s coming from. We’re not being paid to do this. Running a volunteer organization is a lot of work: it takes dedication and it’s hard to ensure succession. Because we are very passionate about this, we have high standards when it comes to execution, but sometimes if you leave it to someone else they may not feel as strongly about it so they may not do it the way you would do it. Which means we often do a lot of stuff by ourselves, and that can lead to burnout. If you’re not focused on what you want out of it, you can lose yourself and it can feel like a thankless job.
I’m sad to see my co-organizer go but I respect her decision. It is okay to want other things out of life, professionally in this case. I myself have been thinking about this increasingly, that perhaps I want to hang up my cape in this sense and explore growing myself through other roles and experiences. I would still like to try crack this succession thing and have been working with some people to grow the team and even things out in terms of responsibilities. But yeah it’s very difficult to be doing something like this on the side – it’s energy draining at the end of the day.
What’s next: Masters at the Georgia Institute of Technology and coordinating a network of African AI Researchers
My venturing into Data Science was with little experience so I’ve done a lot of learning on the job, but feel I haven’t really followed a systematic learning path. My learning has been very driven by business needs, for example when our payments product needed a risk model, I dug into risk and anomaly detection and built that skill. If our messaging product needed a language model, for say, language detection, I’d delve into some NLP. I have not always been able to dig deeper into my interests. Going back to school, I’m really just looking for depth — a systematic way to explore different things in data science and machine learning, and then start to say “I like this, I want to spend the next 2-5 years working in this field.”
Right now, I am starting to think that field may be NLP and see how I can make significant contributions being specifically focused on tools and resources for low-resource languages, particularly African languages. I am interested in Robotics too and hope this course will give me an opportunity to start delving into this.
I am also taking a part-time role coordinating a network of excellence in AI for sub-Saharan Africa under the Artificial Intelligence for Development Initiative. I’m looking forward to fostering relationships and collaboration with researchers, policy makers and practitioners across the continent and playing my part towards making Africa better prepared for the age of Artificial Intelligence.