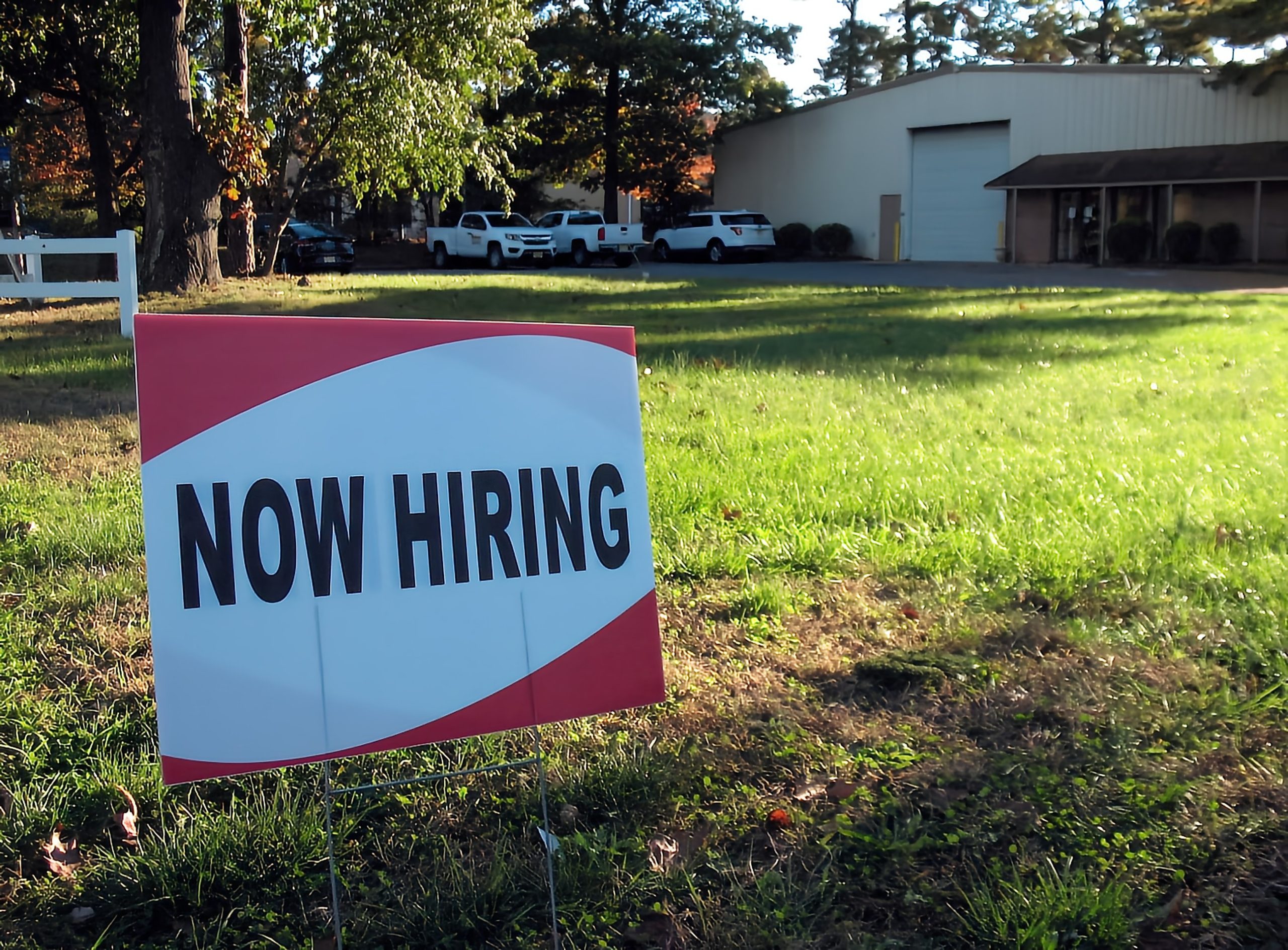
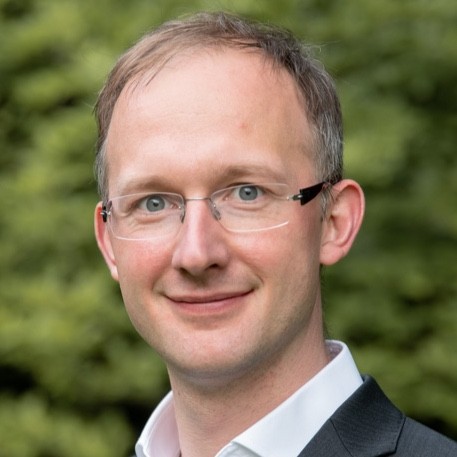
🔬 Original Article by Marco Meyer, Research Group Lead at University of Hamburg, Director at Principia Advisory. LinkedIn.Â
Remember the movie Minority Report? Starring Tom Cruise, the movie showed us with a world in which police apprehended people before they committed crimes based on the foreknowledge of three psychics.
What felt like a science fiction dystopia when the movie was released in 2002 had become a reality in US courtrooms soon after. The COMPAS algorithm predicts a defendant’s risk of committing another misdemeanor or felony within 2 years of assessment based on 137 features about an individual and their criminal record. These predictions have been used in courtrooms around the US to inform decisions about bond amounts to the length of sentences, as reporting by ProPublica revealed in 2016.
The report produced a public outcry because the algorithm’s output was biased against blacks, falsely flagging blacks a lot more frequently as future reoffenders than whites. This finding catalyzed a lot of important work on biases in algorithms. It has sensitized us to the problem of bias in algorithmic decision making and given rise to dozens of AI fairness tools that check algorithms for differential impact.
The problem of pseudo-science in algorithmic decision making
I wish another finding of the report would have received equal attention: The finding that the overall accuracy of predictions was just 65%. In fact, a study by Julia Dressel and Hany Farid showed that untrained people recruited from a popular crowdsourcing marketplace are as good as the COMPAS algorithm at predicting recidivism if presented with just seven features about defendants. While COMPAS draws on 137 features to make its predictions, the same accuracy can be achieved by a simpler algorithm using just two features: age and total numbers of previous predictions.
In other words, the COMPAS algorithm is based on pseudo-science. The mark of science is that it gives us better predictions than our gut reactions. But COMPAS cannot beat the common-and-garden predictions by untrained people. What’s worse, it inherits our biases.
Pseudo-science in hiring algorithms
The problem of basing algorithmic decision making on pseudo-science is not limited to decisions about criminal sentencing. Mona Sloane, Emanuel Moss, and Rumman Chowdhury show in a new article that pseudo-science is still lurking in algorithms affecting fundamental decisions about our lives. The authors have analyzed algorithmic decision-making systems in hiring.
What they found is astounding. There have long been systems for scanning hiring platforms for good candidates and to filter resumes for credential criteria. Yet a new generation of systems are even more deeply integrated into the hiring process:
“These screening technologies variously evaluate applicants by assessing their aptitude for a role through online game playing, analyzing their speech and/or mannerisms to predict on-the-job performance, or by analyzing Meyers-Briggs-styled “personality assessment” questionnaires.”
(Sloane et al.)
What, if anything, is wrong with this? Not the tech as such. The data science techniques underlying these systems are cutting edge, using supervised machine learning, computer vision, and natural language processing to analyze inputs from written text to micro-expressions.
The issue is that mannerisms and Meyers-Briggs personality assessments provide no reliable insight into future job performance. This bias is not due to the data or model choice. Rather, these hiring algorithms make claims to knowledge that are wrong. They claim that what you can infer about a person’s inner states by external attributes such as facial expression or pupil dilation is useful in predicting job performance. Yet there is no scientific evidence for this claim.
Why pseudo-science takes hold in organizations
If HR algorithms based on junk science are ineffective, won’t they soon disappear? They will not, for two reasons. First, their ineffectiveness is hard to discover. The reason is that hiring occurs in a wicked learning environment, as Robin Hogarth, Tomas Lejarraga, and Emre Soyer have pointed out. In kind learning environments, you get fast and accurate feedback on your predictions. For instance, match scores will quickly reveal whether a new basketball technique is effective. By contrast, in wicked learning environments, the feedback you get on your predictions is delayed or inaccurate. In the case of hiring, job performance can only be discerned after months or years. What is worse, comparing the performance of successful to unsuccessful applicants is impossible, because applicants that are screened out don’t have an opportunity to prove themselves.
The second reason is that managers are glad for any tool that reduces the number of resumes they need to sift through. Mats Alvesson and André Spicer have argued that research on organizations has overplayed the degree to which organizations seek to be smart. No doubt, organizations need to deal intelligently with information in many domains, and increasingly so. But functional stupidity is an equally important part of organizational life. Functional stupidity is a lack of reflection, reasoning, and justification that is supported by the organization. The key insight is that organizational stupidity can maintaining organizational order and conserve resources. From the organizational perspective, the selling point of HR algorithms is that they provide an accepted and cheap way to reduce the number of applicants that need to be considered. Scrutinizing the effectiveness of these algorithms is therefore counter-productive from an organizational perspective. Suppose the organization scrutinizes the scientific soundness of hiring algorithms. If the inquiry finds that the algorithms are effective, it results in no change. If the inquiry finds that the algorithms are ineffective, the organization has undermined the algorithms’ acceptability. As a result, they deprived themselves of a solution to the problem of how to sift through many applicants. We should not expect most organizations to go out on a limb to shoot themselves in the foot.
How to audit for pseudo-science
These reasons for the persistence of pseudo-science in hiring algorithms have two implications. Since the ineffectiveness of hiring algorithms is difficult to discover, we need to ensure that algorithmic audits include checks for the soundness of its scientific assumptions. Currently, the emphasis of algorithmic audits is to assess the accuracy of algorithms. But in wicked learning environments, accuracy is all but meaningless as a metric. Another focus of current algorithm audits is to screen for bias, e.g., by comparing predictions for different groups. Yet hiring mechanisms can make arbitrary recommendations without being biased. What might these checks for scientific soundness look like? There are at least two questions audits need to answer:
- What is the assumed relationship between the input data and the purposes for what the output data is used? E.g., what is the assumed relationship between the data about applicants and their job performance?
- Are these assumptions backed up by research? E.g., does research show a link between micro-expressions and job performance?
Since ignorance about the pseudo-science in hiring algorithms can be functional for the organizations using them, we cannot rely on organizations to advocate for science. Worse still: the fact that organizations have an interest to stay ignorant about the scientific underpinnings of the hiring mechanisms means that the companies building these algorithms have little incentive to compete on scientific rigor. We should not expect market mechanisms to improve the scientific foundations of hiring algorithms over time or companies that overstep scientific boundaries to disappear from the market.
Instead, a combination of regulation and professional ethics is required to move past pseudo-science in hiring algorithms. Regulation can set standards for algorithmic audits that include checks for algorithmic soundness. But regulation alone can be circumvented if HR professionals are not socialized to the importance of scientific soundness of hiring mechanisms. For instance, over the last decades, hiring has become more inclusive. Anti-discrimination laws have played an important role. But to achieve a truly inclusive hiring process, a wide range of practices needs to change, from making career websites accessible to all to expanding where companies advertise. Implementing these practices cannot be achieved by regulation alone but need to be driven by inclusion-savvy HR professionals. Making scientific soundness part of professional ethics requires to educate HR professionals and to create expectations. Part of the algorithmic literacy of HR professionals needs to be a sensitivity to the importance of a sound scientific basis to any algorithm they may use. The reputation of HR professionals increasingly depends on pushing for inclusivity in hiring. Ensuring the scientific soundness of their tools deserves the same status.