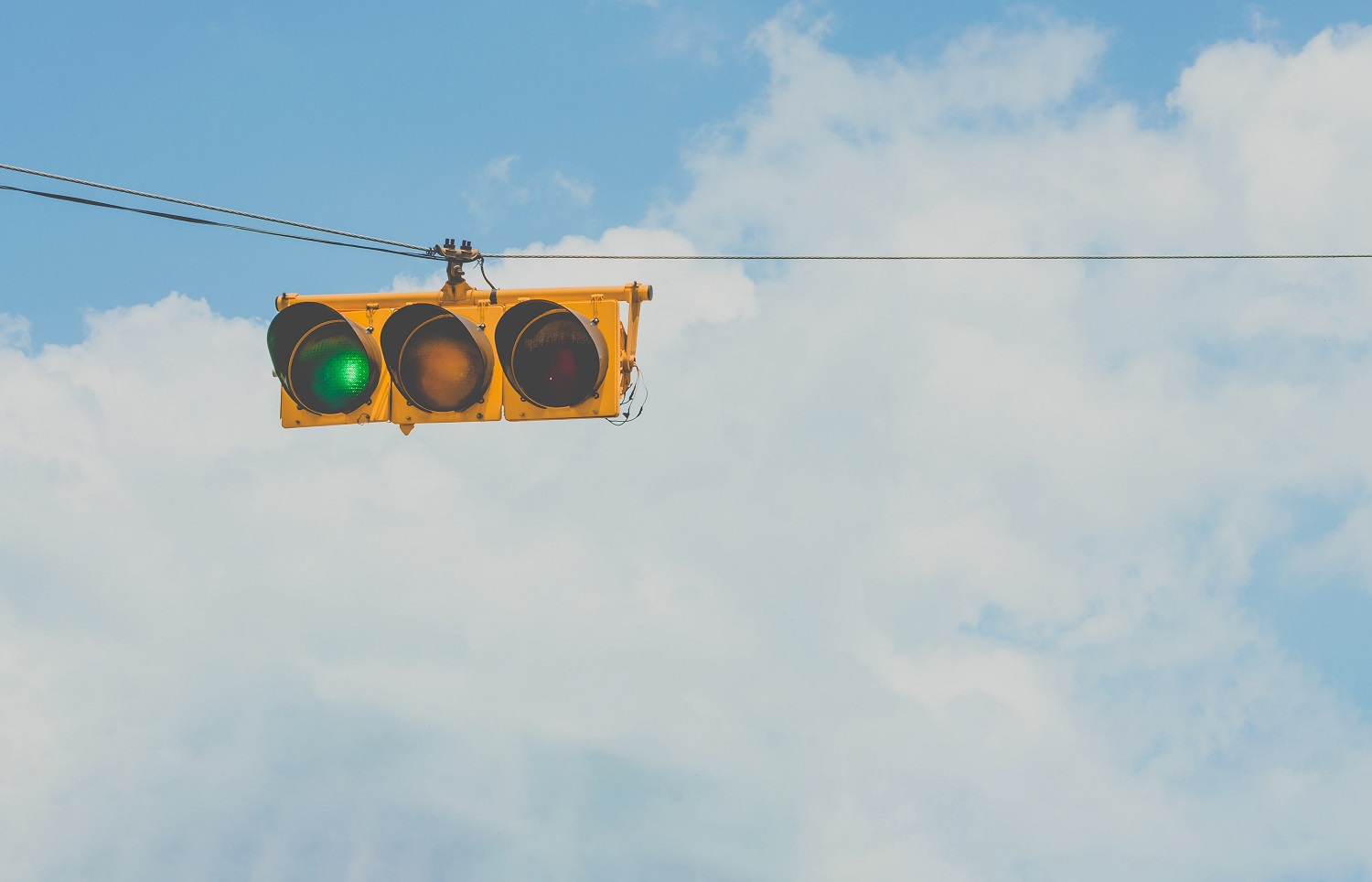
Authors: Abhishek Gupta, Erick Galinkin
Abstract
Security and ethics are both core to ensuring that a machine learning system can be trusted. In production machine learning, there is generally a hand-off from those who build a model to those who deploy a model. In this hand-off, the engineers responsible for model deployment are often not privy to the details of the model and thus, the potential vulnerabilities associated with its usage, exposure, or compromise.
Techniques such as model theft, model inversion, or model misuse may not be considered in model deployment, and so it is incumbent upon data scientists and machine learning engineers to understand these potential risks so they can communicate them to the engineers deploying and hosting their models. This is an open problem in the machine learning community and in order to help alleviate this issue, automated systems for validating privacy and security of models need to be developed, which will help to lower the burden of implementing these hand-offs and increasing the ubiquity of their adoption.