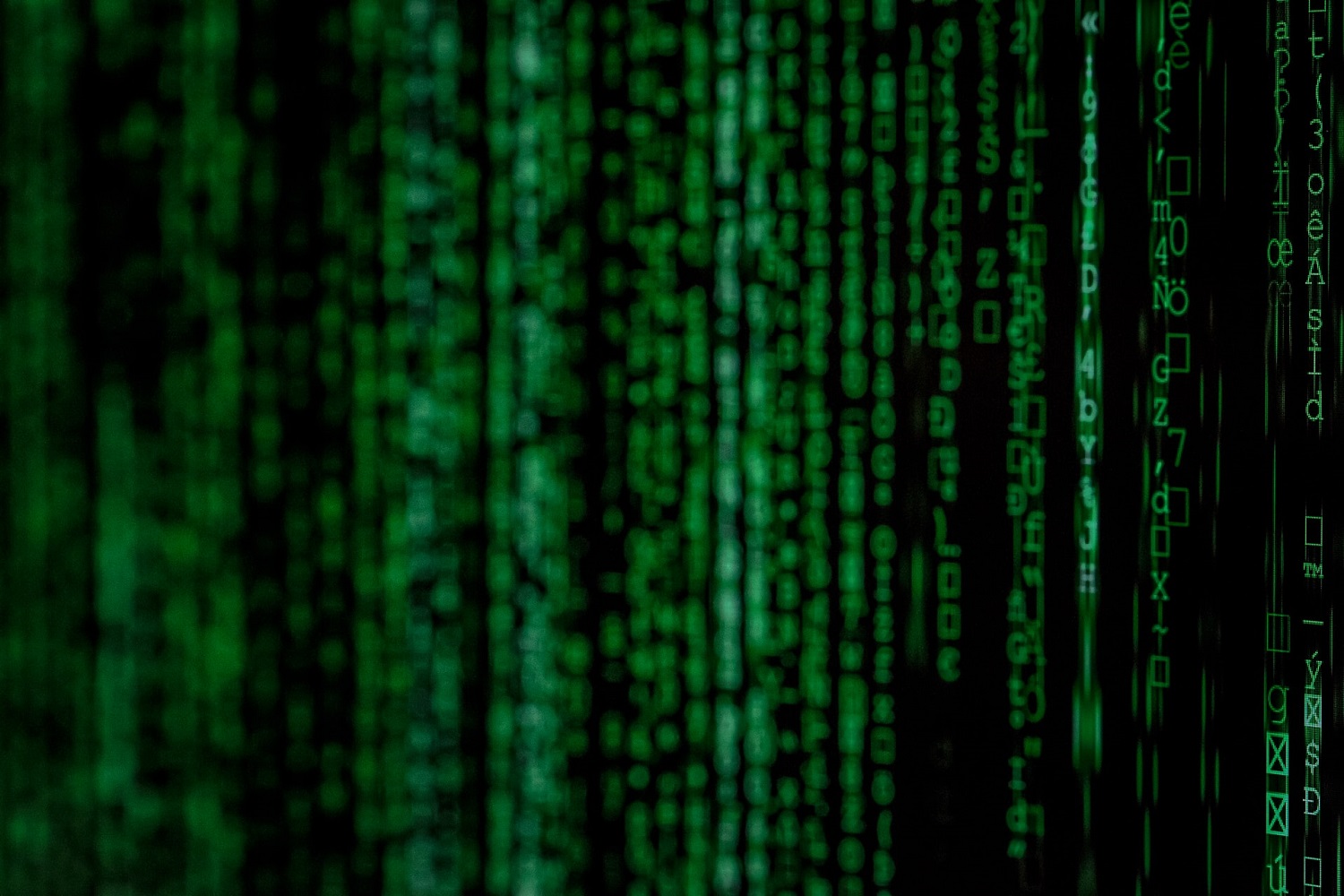
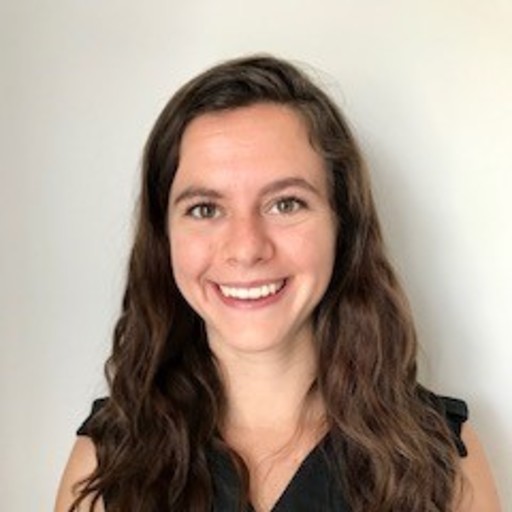
Summary contributed by our researcher Alexandrine Royer, who works at The Foundation for Genocide Education.
*Link to original paper + authors at the bottom.
Overview: This paper introduces the methodological framework behind the Green Algorithm, a free online tool that can provide a standard and reliable estimate of any computational task’s carbon emissions, providing researchers and industries with a sense of their environmental impact.
In the past year, we have witnessed costly wildfires in Australia, increasing droughts in the continental US, heavy rainfall in India, and more natural disasters. No corner of the globe is left untouched by the direct consequences of our rapidly warming world, and there is mounting public pressure for industries to make their activities ecologically viable. In the tech realm, privacy, security, discrimination, bias, and fairness are the common buzzwords that surround AI, yet the word “green” is rarely present.
With the world facing an acute climate crisis, high-performance computing’s carbon emissions continue to be overlooked and underappreciated. Lannelongue, Grealey, Inouye (the authors of the original paper) are hoping to change the conversation by introducing the free online tool Green Algorithms, which can estimate the carbon impact ā or CO2 equivalent- of any computational task. By integrating metrics such as running time, type of computing core, memory used, and the computing facility’s efficiency and location, the Green Algorithm tool will allow researchers and corporations alike to get a reliable estimate of their computation’s environmental impact.
Climate policy is becoming a pressing issue for governments who are making new climate commitments. The EU has committed to reducing all its greenhouse-gas emissions to zero by 2050. Chinese president Xi Jinping has jumped on the green bandwagon and promised to reduce the country’s carbon emissions to zero by 2060. While it is tempting to celebrate these governmental actions, the EU and China are significant investors in data centers and high-performance computing facilities, which release around 100 megatonnes of CO2 emissions annually. When it comes to assessing an algorithm’s environmental impact, we must consider both the energy required to run the system (i.e. the number of cores, running time, data centre efficiency) and the carbon impact of producing such energy (i.e. location and type of energy fuel).
The authors note that while there have been advances in green computing, these are concentrated on energy-efficient hardware and cloud-related technologies. Power-hungry machine learning models have grown exponentially in the past few years. Although some studies have attempted to calculate such systems’ carbon impacts, they rely on users’ self-monitoring and apply to only particular hardware or software. As stated by the authors, “to facilitate green computing and widespread user uptake, there is a clear and arguably urgent, need for both a general and easy-to-use methodology for estimating carbon impact that can be applied to any computational task.”
The Green Algorithm can calculate the energy needs of any algorithm by considering its “running time, the number, type and process time of computing cores, the amount of memory mobilized and the power draw of these resources.” The model also accounts for the data centre’s energy efficiency, such as lighting, heating or AC. To estimate the environmental impact behind the energy produced to run these systems, the authors employ a carbon dioxide equivalent to stand in for greenhouse gases’ global warming effects. The environmental impact is assessed by calculating the carbon intensity, being the carbon footprint of producing 1 kWh of energy. The data centre location is also an essential factor, as the source of energy, whether it is hydro or coal or gas, will affect the calculation. The model further provides a pragmatic scaling factor, which multiplies the carbon impact by the “number of times a computational is performed in practice.”
After entering the energy-related details of their algorithms, users will be provided with comparative figures such as the equivalent percentage of carbon emissions for international flights and the number of trees required to sequester the emissions of running their system. The Green Algorithm was tested by the authors for algorithms used in particle simulations and DNA irradiation, weather forecasting, and natural language processing, making it applicable to a wide variety of computational tasks. As summarized by the authors, “besides drawing attention to the growing issues of carbon emissions of data centres, one of the benefits of presenting a detailed open methodology and tool is to provide users with the information they need to reduce their environmental footprint.” The results produced by the Green Algorithm should be taken as a generalizable and relative figure, as the authors note there are limitations to the tool, such as an omission of hyperthreading, the exact breakdown of energy mixing in a given country, and the lack of a standard approach to calculating power usage effectiveness.
The Green Algorithm is a welcome development in monitoring the environmental impact of AI-related advances. The use of such tools can help stir proactive solutions to mitigating the environmental consequences of modern computation. While the field of machine learning appears to be fueled by relentless growth, policymakers, industry leaders, and the public will need to consider whether the environmental costs of introducing these new systems are far-outweighed by the potential societal benefits.
Original paper by Loice Lannelongue, Jason Grealey, Michael Inouye: https://arxiv.org/ftp/arxiv/papers/2007/2007.07610.pdf