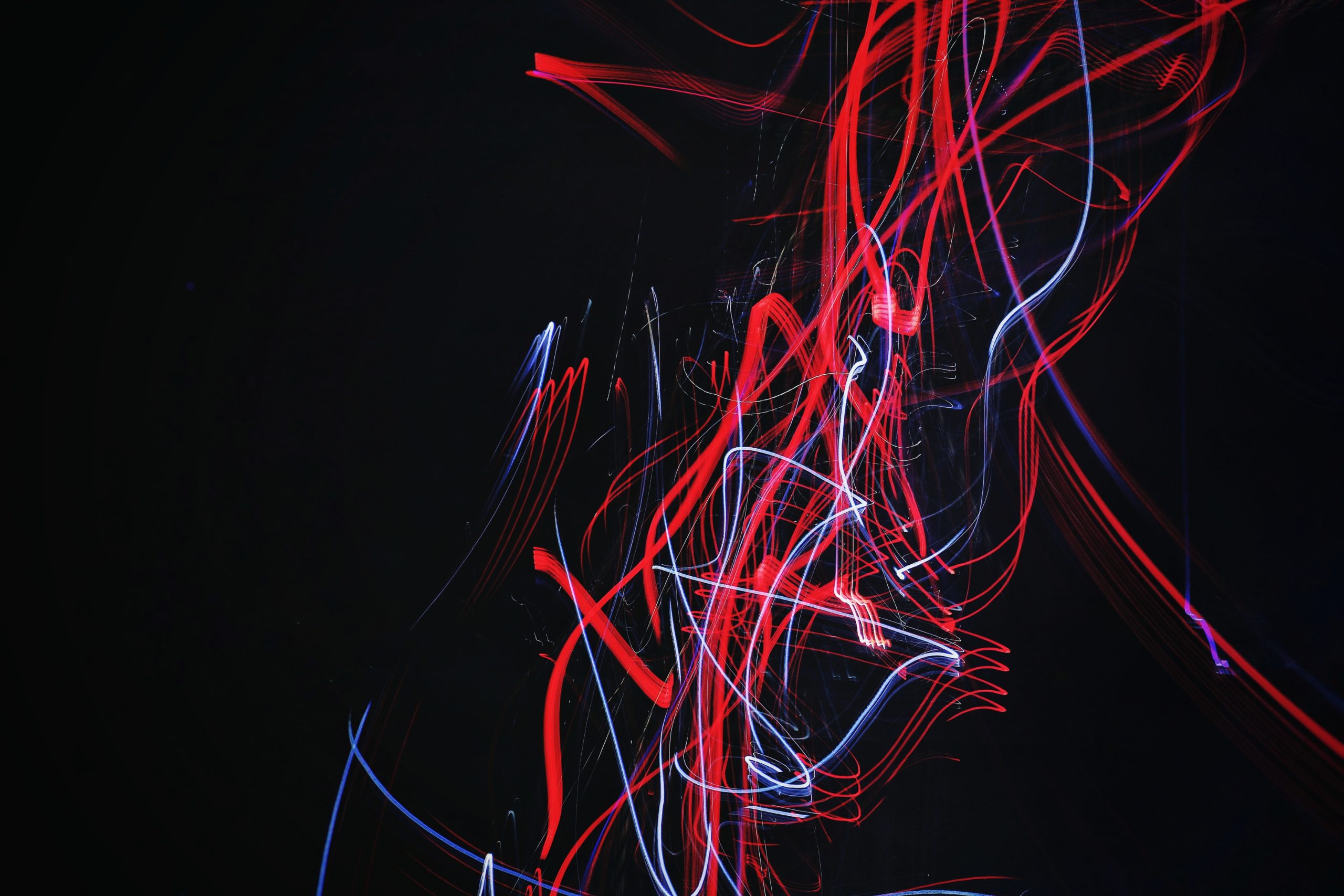
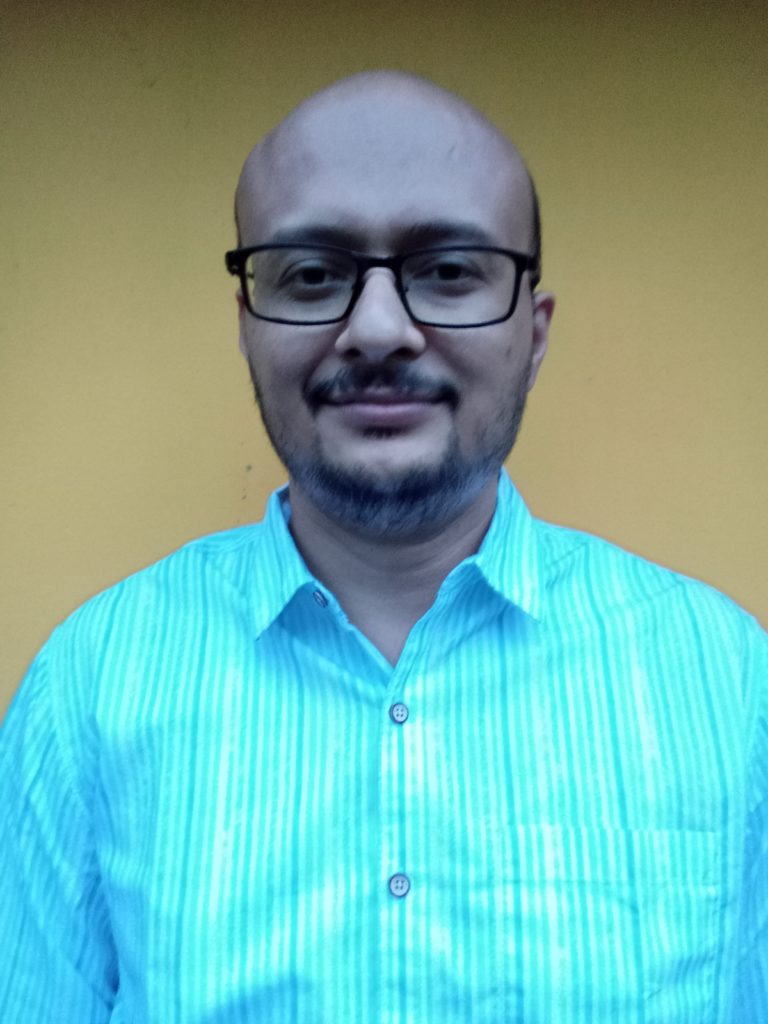
š¬ Research summary by Angshuman Kaushik, Researcher in AI Policy, Governance and Ethics.
[Original paper by John Basl, Ronald Sandler and Steven Tiell]
Overview: Ā AI or data ethics principles or frameworks meant to demonstrate a commitment to addressing the challenges posed by AI are ubiquitous and are an āeasy first stepā. However, the harder task is to operationalizeĀ them. This report, inter alia, stipulates strategies for putting those principles into practice.Ā Ā Ā
Introduction
Amidst the chaotic AI Ethics principles landscape, this report emerges as a much-needed guide in understanding the entire gamut of issues related with the application of those principles in governance scenarios. It emphasizes the complexities associated with moving from general commitments to substantive specifications in AI and data ethics. According to it, much of this complexity arises from three key factors;
- ethical concepts such as justice and transparency that often have many senses and meaning;
- which senses of ethical concepts are operative or appropriate is often contextual; and
- ethical concepts are multidimensional e.g., in terms of what needs to be transparent, to whom, and in what form.
Further, the objectives of the report are to;
- demonstrate the importance and complexity of moving from general ethical concepts and principles to action-guiding substantive content, i.e., normative content;
- provide detailed analysis of two widely discussed and interconnected ethical concepts, justice and transparency; and
indicate strategies for moving from general ethical concepts and principles to more specific normative content and ultimately to operationalizing that content.
AI Ethics – Understanding the challenges
The report talks about considerable convergence among the many AI ethics frameworks that have been developed. They coalesce around core concepts, some of which are individual-oriented, others society-oriented and still others system-oriented. However, according to the researchers, enunciating ethical values and principles is only the first step in addressing AI and data ethics challenges and it is in many ways the easiest. The much harder work is the following;
- substantively specifying the content of the concepts, principles and commitments; and
- building professional, social and organizational capacity to realize these in practice.
An example from the field of bioethics
In order to better comprehend the obstacles encountered in moving from general ethical concepts to a functioning AI framework (normative content), the paper takes the case of informed consent in bioethics, which is widely recognized as a crucial component of ethical clinical practice. Informed consent operationalizes the principle of individual autonomy. Practically, it requires the fulfillment of three conditions namely;
- disclosure ā provision of clear, accurate and relevant information to the subjects;
- comprehension ā information is provided to the subjects in a way that they can understand; and
- voluntariness – the subjects make the decision without undue influence or coercion.
The enforcement of these three conditions is the task of bioethicists, hospital ethics committees and institutional review boards. They prepare guidelines, best practices, procedures etc., for meeting the above informed consent conditions.
According to the researchers, while informed consent is meant to protect the value of autonomy and express respect for persons, a general commitment to the principle of informed consent is just the beginning. The principle must be explicated and operationalized before it is meaningful and useful in practice. The same is true for principles of AI and data ethics. The researchers then, narrow down their focus on the complexities involved in moving from core concepts and principles to operationalization of the normative content for two prominently discussed and interconnected AI and data ethics concepts: justice and transparency.
Meaning of justice in AI
The report mentions that the concept of justice is a complex one, and can mean different things in different contexts. To determine what justice in AI and data use requires in a particular context, it is imperative to clarify the normative content and underlying values. Only then it is possible to specify what is required in specific cases, and in turn how or to what extent justice can be operationalized in technical systems. According to the report, the general principle of justice is that all people should be equally respected and valued in social, economic and political systems and processes. However, there are many ways this very general principle of justice intersects with social structures and systems. As a result, there is a diverse set of more specific justice-oriented principles such as, procedural, distributive and recognition justice.
What does committing to justice mean?
The researchers consider context to be critically important in determining which justice-oriented principles take precedence. Therefore, the first step in specifying the normative content is to identify the justice-oriented principles that are crucial to the work that the AI system does. Only then can a commitment to justice be effectively put into practice. Articulating the relevant justice-oriented principles will also require considering organizational missions, the types of products and services involved, how those products and services could impact communities and individuals etc. In identifying these, it will be helpful to reflect on similar cases and carefully consider the sorts of concerns that people have raised about AI systems. The researchers have cited two hypothetical cases to illustrate this. Further, the report states that the diversity of the justice-oriented principles and the need to make context-specific determinations about which are relevant and which to prioritize expose the limits of a strictly algorithmic manner in incorporating justice in AI systems. The reason being, firstly, there is no singular, general justice-oriented constraint, optimization or utility function and secondly, there will not be a strictly algorithmic way to fully incorporate justice into decision-making, even once the relevant justice considerations have been identified. The report then goes on to ask the question as to how and to what extent can the salient aspects of justice be achieved algorithmically. According to the researchers, accomplishing justice in AI will require developing justice-informed, techno-social or human-algorithm systems.Ā AI systems can supportĀ social workers in service determinations, admissions officers in college admissions determinations, or healthcare professionals in diagnostic determinations and they might even be able to help reduce biases in those processes. According to the researchers, a commitment to justice in AI involves remaining open to the possibility that sometimes an AI-oriented approach might not be a just one. They stress on the fact that, organizations that are committed to justice in AI will require significant organizational capacity and processes to operationalize and implement their commitment, in addition to technical capacity and expertise. Reliance upon techno solutionism or on standards developed in other contexts is not desirable.
Transparency in AI
In the view of the researchers, in spite of the role that transparency plays in helping to achieve justice, it can also play an important role in realizing other concepts and values. They also lay down the many ways in which a decision system could be made transparent. The forms that commitments to transparency may take are as follows;
- Interpretability ā requiring AI systems to be interpretable;
- Explainability ā a decision-making system is explainable when it is possible to offer stakeholders an explanation that can be understood as justifying a given decision;
- Justified Opacity ā transparency about the reasons for adopting opaque systems can serve to justify other forms of opacity; and
Auditability ā a carefully constructed audit can provide assurance that decision-making systems broadly are trustworthy, reliable and compliant.
Way forward
The researchers point out that for organizations to be successful in realizing their ethical commitments and accomplishing responsible AI, they must think broadly about how to build ethical capacity within their organizations. Some of the initiatives cited are as follows;
- creating AI and data ethics committees that can aid in developing policies and other governance measures;
- meaningfully engaging with impacted communities to better comprehend ethical issues and other ways to broaden perspectives and collaborations;
- training and education;
- integrating ethics into practice; and
- building an AI and data ethics community.
Between the lines
The plethora of vaguely formulated AI Ethics principles, guidelines, standards etc., that have come to dominate the AI Ethics space in the last few years have hardly aided in operationalizing ethical AI in practice. With the passage of time such principles have begun to sound banal and an appendage to an organizationās other āsignificant documentsā. In such a scenario, this report serves as a guidepost by laying down strategies for moving from general ethical concepts and principles to more specific normative content and ultimately to operationalizing that content. Further, the report simplifies comprehension of the complexities involved in such a transition, by the use of illustrations. The report can prove handy and a āgo-to guideā not only for those entities that are struggling to formulate ethical principles but also to those that are trying to get an AI Ethics framework up and running.Ā