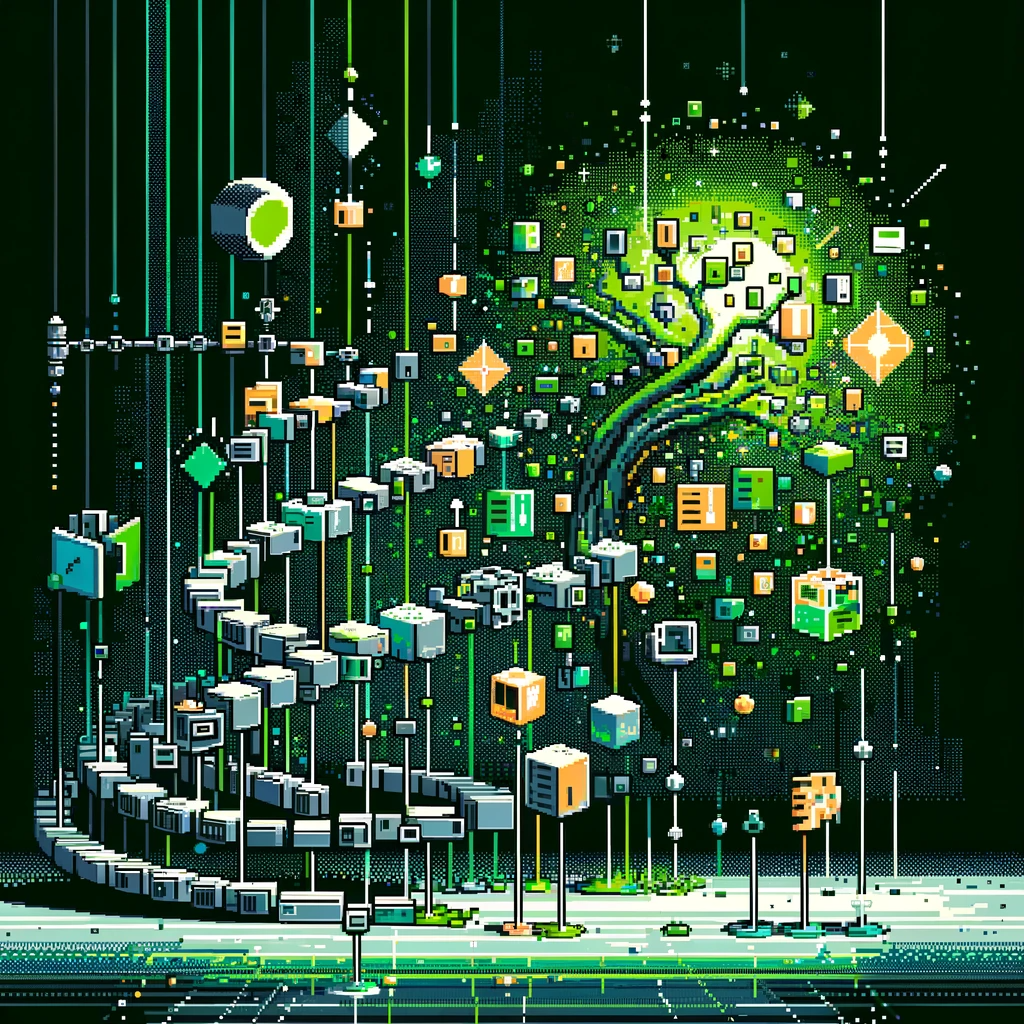
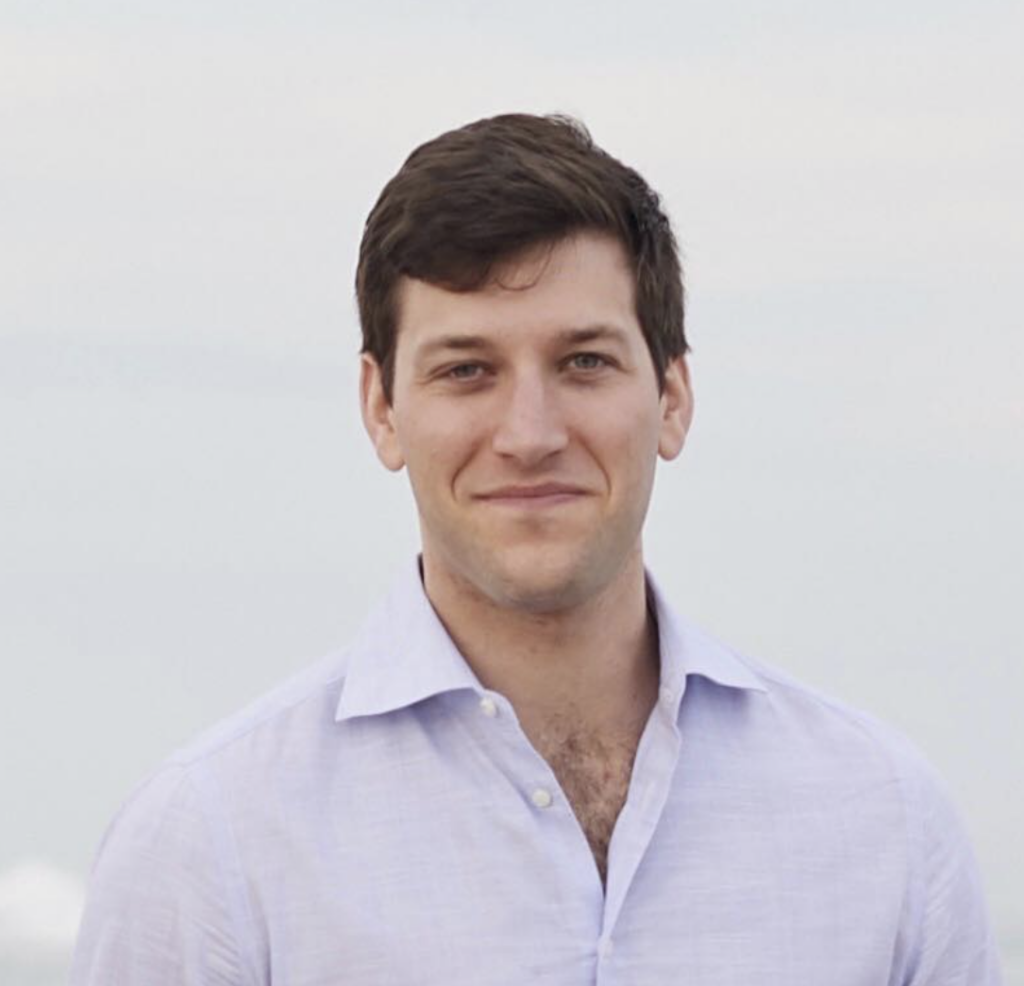
🔬 Research Summary by Griffin Adams, a final year NLP PhD student at Columbia University under Noémie Elhadad and Kathleen McKeown, who will be starting as the Head of Clinical NLP for Stability AI in 2024.
[Original paper by Griffin Adams, Alexander R. Fabbri, Faisal Ladhak, Eric Lehman, and Noémie Elhadad]
Overview: Selecting the “right” amount of information to include in a summary is difficult: a good summary should be detailed and entity-centric without being overly dense and hard to follow. To better understand this tradeoff, we solicit increasingly dense GPT-4 summaries with what we refer to as a “Chain of Density” (CoD) prompt. Specifically, GPT-4 generates an initial entity sparse summary before iteratively incorporating missing salient entities without increasing the length.
Introduction
Automatic summarization has come a long way in the past few years, largely due to a paradigm shift away from supervised fine-tuning on labeled datasets to zero-shot prompting with Large Language Models (LLMs), such as GPT-4 (OpenAI, 2023). Careful prompting can enable fine-grained control over summary characteristics, such as length, topics, and style, without additional training. An overlooked aspect is the information density of a summary. Theoretically, as a compression of another text, a summary should be denser–containing a higher concentration of information–than the source document. Given the high latency of LLM decoding, covering more information in fewer words is a worthy goal, especially for real-time applications. Yet, how dense is an open question. A summary is uninformative if it contains insufficient detail. However, if it contains too much information, it can become difficult to follow without increasing the overall length. Conveying more information subject to a fixed token budget requires a combination of abstraction, compression, and fusion. There is a limit to how much space can be made for additional information before becoming illegible or even factually incorrect.
Key Insights
In this paper, we seek to identify the optimal balance between detail and readability by using GPT-4 to generate increasingly entity-dense (e.g., detailed) summaries and have humans provide preference assessments.
The Prompt
The Chain of Density (CoD) prompt is achieved with a single prompt to GPT-4, which is tasked with writing five summaries of a provided article. At each step, 1-3 additional details (entities) are added to the previous summary without increasing the length. Existing content is re-written to make room for new entities (e.g., compression, fusion).
The Data
We randomly sample 100 articles from a CNN/DailyMail news article collection.
Human Feedback
We conduct a human evaluation to assess the impact of densification on human assessments of overall quality. Specifically, the first four authors of the paper were presented with randomly shuffled CoD summaries, along with the articles, for the same 100 articles (5 steps * 100 = 500 total summaries). Based on the same definition of a “good summary,” each annotator indicated their top preferred summary. Our results indicated that humans prefer summaries that are almost as dense as human-written summaries and more dense than summaries generated from a simple GPT-4 prompt: “Write a VERY short summary of the Article. Do not exceed 70 words.”
Between the lines
We study the impact of summary densification on human preferences for overall quality. A degree of densification is preferred, yet it is very difficult to maintain readability and coherence when summaries contain too many entities per token. We open-source annotated test sets and a larger unannotated training set for further research into the topic of fixed-length, variable-density summarization. Future work should identify the optimal information level to include for each unique article. Given the rise of open-source LLMs (LLama, Mistral), this expensive Chain of Density prompt could be distilled into a single model through fine-tuning.