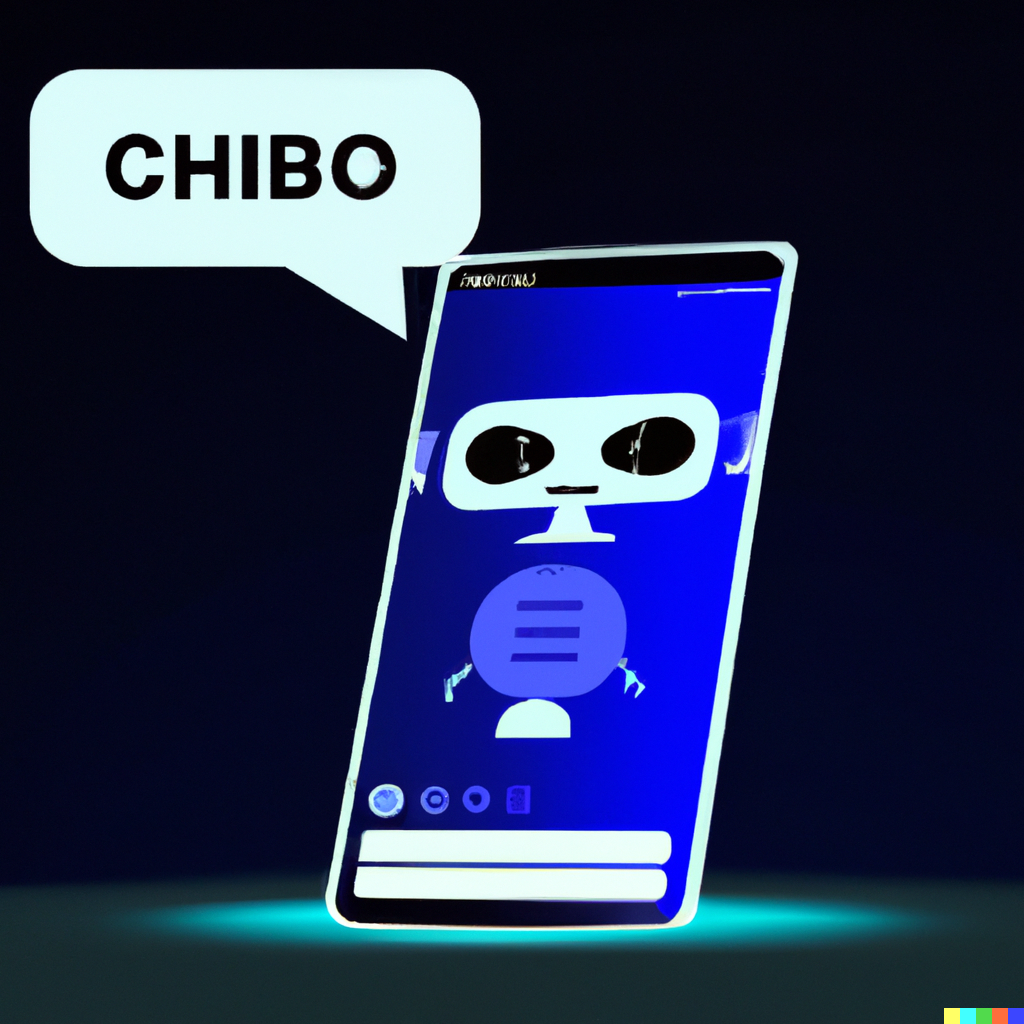
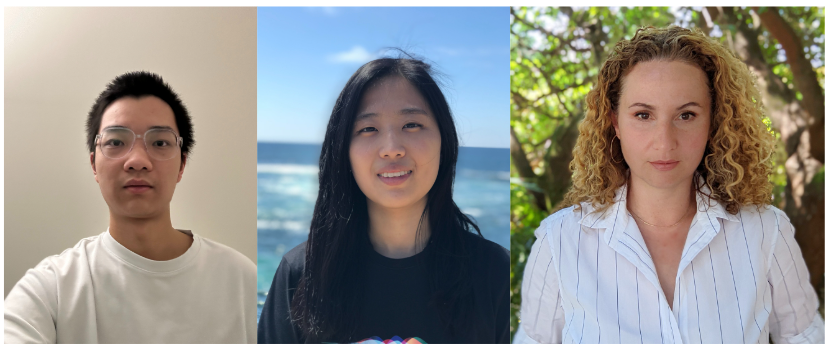
🔬 Research Summary by Shangbin Feng, Chan Young Park, and Yulia Tsvetkov.
Shangbin Feng is a Ph.D. student at University of Washington.
Chan Young Park is a Ph.D. student at Carnegie Mellon University, studying the intersection of computational social science and NLP.
Yulia Tsvetkov is an associate professor at University of Washington.
[Original paper by Shangbin Feng, Chan Young Park, Yuhan Liu, Yulia Tsvetkov]
Overview: This paper studies the political biases of large language models and their impact on fairness in downstream tasks and NLP applications. The authors propose an end-to-end framework, investigating how political opinions in language model training data propagate to language models and then to biased predictions in critical downstream tasks such as hate speech and misinformation detection. This paper uniquely finds that no language model will be entirely free from political biases. Thus, how to mitigate the political biases and unfairness of large language models is of critical importance.
Introduction
Language models (LMs) are pretrained on diverse data sources—news, discussion forums, books, and online encyclopedias. A significant portion of this data includes facts and opinions which, on the one hand, celebrate democracy and diversity of ideas and, on the other hand, are inherently socially biased. Our work develops new methods to (1) measure media biases in LMs trained on such corpora along social and economic axes and (2) measure the fairness of downstream NLP models trained on top of politically biased LMs. We focus on hate speech and misinformation detection, aiming to empirically quantify the effects of political (social, economic) biases in pretraining data on the fairness of high-stakes, social-oriented tasks. Our findings reveal that pretrained LMs have political leanings that reinforce the polarization present in pretraining corpora, propagating social biases into hate speech predictions and media biases into misinformation detectors. We discuss the implications of our findings for NLP research and propose future directions to mitigate unfairness.
Key Insights
Motivation and Methodology
It is well established in NLP and machine learning research that social biases in language, expressed subtly and seemingly benignly, propagate and are even amplified in user-facing NLP systems that are trained using the data from the internet. In recent years, many studies have highlighted the risks of such biases. However, we noticed two important gaps in existing work. First, studies that highlight the risks of bias in NLP models often use synthetic data, such as explicitly toxic or prejudiced comments, which are less common on the Web and are relatively easy to detect and automatically filter out from the training data of language models. Second, studies that propose interventions and bias mitigation approaches often focus on individual components of the machine learning pipeline rather than understanding bias propagation end-to-end, from Web data to large language models like ChatGPT and then to user-facing technologies that are built upon language models. We wanted to focus on a more realistic setting, with common examples of biases in language, and on a more holistic, end-to-end analysis of potential harms.
This is why we decided to focus on potential biases in political discussions because discussions about polarizing social and economic issues are abundant in pretraining data sourced from news, forums, books, and online encyclopedias, and this language inevitably perpetuates social stereotypes. As an example of more realistic user-facing systems built upon politically biased language models, we focused on high-stakes social-oriented tasks, such as hate speech and misinformation detection. We wanted to understand whether valid and valuable political discussions, possibly about polarizing issues—climate change, gun control, abortion, wage gaps, taxes, same-sex marriage, and more—that represent the diversity and plurality of opinions and cannot be simply filtered from the training data can lead to unfair decisions in hate speech detection and misinformation detection.
Findings and Impact
We show the study’s implications in evaluating the fairness of downstream NLP models. Our experiments demonstrate, across several data domains (Reddit, news outlets), partisan news datasets, and language model architectures, that different pretrained language models have different underlying political leanings, capturing the political polarization in the data. While the overall performance (e.g., classification accuracy) of hate speech and misinformation detectors remains relatively consistent across such politically biased language models, these models exhibit significantly different behaviors in hate speech detection against different identity groups and social attributes, such as gender, race, ethnicity, religion, and sexual orientation, and significantly biased decisions in misinformation detection with respect to political leanings of source media.
Importantly, our work highlights that pernicious biases and unfairness in NLP tasks can be caused by non-toxic and non-malicious data. This creates a dilemma because political opinions cannot be filtered from training data. This would lead to censorship and exclusion from political participation. However, keeping them in training data inevitably leads to unfairness in NLP models. Ultimately, this means that no language model can be entirely free from social biases and underscores the need to find new technical and policy approaches to deal with model unfairness.
Between the lines
An important conclusion from our research is that to develop ethical and equitable technologies, we need to look at more realistic data and realistic scenarios. There’s no fairness without awareness. This would require us to consider the full complexity of language (including understanding people’s intents and presuppositions) to ground our research in social science, policy research, in philosophy and develop more interesting, advanced technical approaches to machine learning model controllability and interpretability. In the paper, we discuss several concrete ideas for future research. Ultimately, we hope our findings will inspire more interesting, interdisciplinary research in computational ethics and social science.