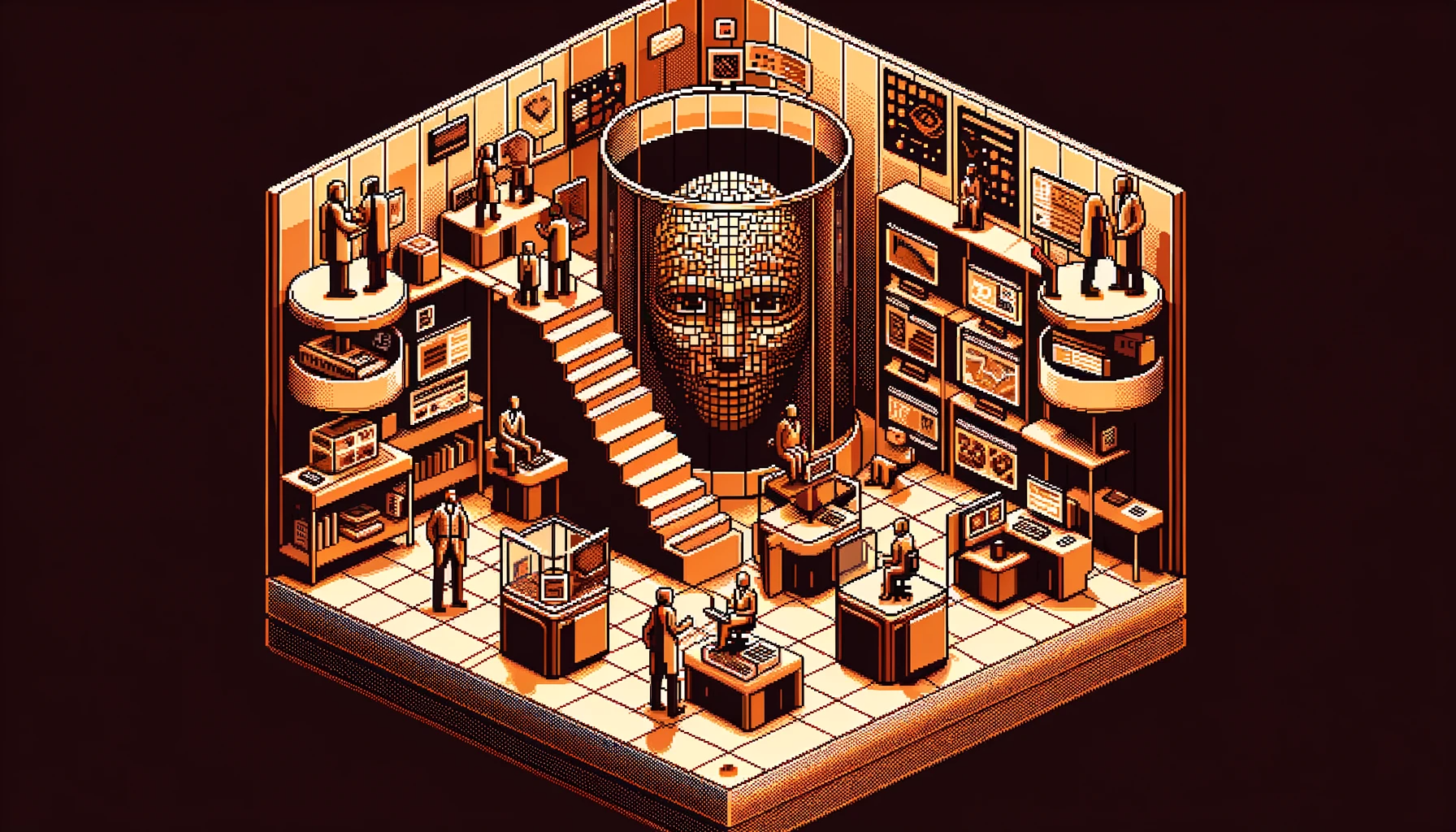
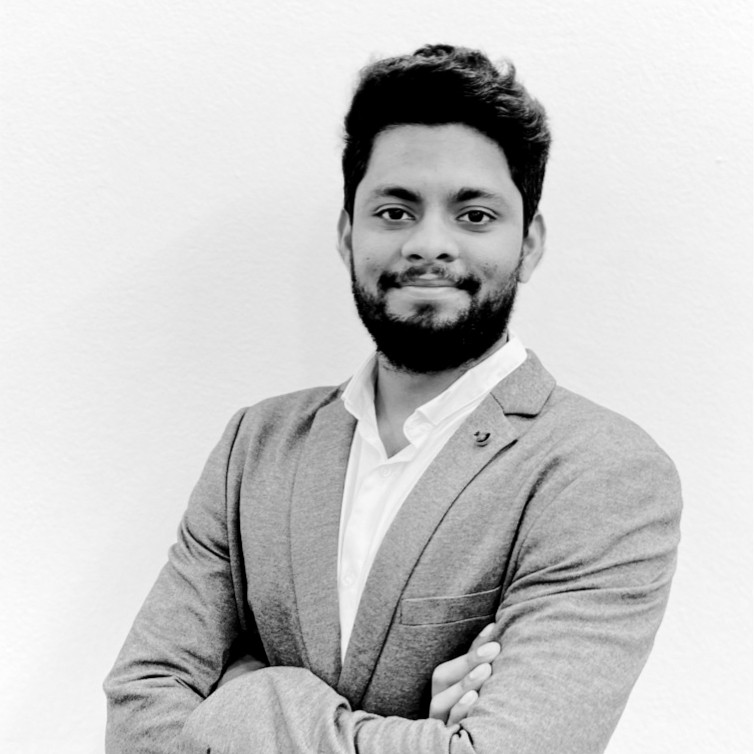
🔬 Research Summary by Arun Teja Polcumpally, a Technology Policy Analyst at Wadhwani Institute of Technology Policy (WITP), New Delhi, India).
NOTE: “The views in this article are expressed solely by the author and do not reflect the views of the Wadhwani Institute of Technology and Policy.”
[Original paper by Benedikt Fecher, Marcel Hebing, Melissa Laufer, Jörg Pohle, and Fabian Sofsky]
Overview: This article investigates the multifaceted impact of Language Model Models (LLMs) in scientific research, addressing their potential applications, limitations, ethical considerations, and the evolving landscape of competencies for researchers. Employing the Delphi method, the study engaged experts through consultations, highlighting insights from 72 initial responses, and in the second stage, the authors surveyed 52 experts. In this stage, they delved deeper into the factors identified from the first stage. While acknowledging LLMs’ assistance in tasks like research summaries and initial paper drafts, shift in the researcher’s competencies, and assisting in language proficiency, concerns emerged regarding their suitability for scholarly write-ups and the potential to undermine the peer review process. There are regulatory challenges, including accountability, privacy, and intellectual property issues.
The findings underscore the need for transparency in LLM-generated content, citing concerns about bias, lack of specificity, and the risk of misinformation. Despite opportunities to streamline administrative work, caution is advised due to the risk of over-reliance on LLMs, potentially hampering researchers’ critical thinking abilities and perpetuating misinformation in scientific discourse.
Introduction
With the influx of numerous LLM applications in the market that assist users in generating content, presentations, images, and videos, the way users work with narratives and discourses is set to change. While multiple sectors affect the narratives and discourses, research is the most important area as it sets the foundation for knowledge. This article timely focuses on this area, providing an analytical take on the impact of LLMS on the research. To evaluate the impact of LLMs, this article adopts the Delphi method to investigate the following research questions.
- What are the potential applications and limitations of using LLMs in the scientific research domain?
- What are the positive and negative effects of LLMs on the science system?
- What are the regulatory and ethical considerations associated with using LLMs in science?
- What are the necessary Competencies and capacities for effectively utilizing LLMs?
Key Insights
The Delphi method involves collecting expert opinions, validating the constructed hypothesis, finding the answer/exploring the research question. The authors used convenience sampling to conduct two-stage consultations with the experts to get their opinions. Taking such sampling makes sense as the Delphi method is used to agree with the experts on a topic/concept. Convenience sampling is more doable than random sampling in this case. In the first stage, the authors collected 72 responses to 12 open questions on the impact of LLMs on the scientific process. These responses were analyzed, and a codebook was prepared to proceed to the second stage of the survey. In this stage, 11 questions were prepared as per the code book themes. The second stage received only 52 responses.
Studying the previous work in this area, the paper asserts that LLMs assist in making research summaries, making grammar corrections, and preparing a literature review. They are considered helpful in generating research ideas and creating the first draft of a paper. LLMs are opined to assist the researchers in investing their time in deciding what to communicate rather than how to write the research outcomes (section 2.2). However, there are apprehensions about using these LLMs in making scholarly writeups.
From the previous works, the paper also identified that the competencies of researchers would take a slight shift because of the adoption of LLMs. As they assist in writing, grammar, and articulation, researchers are expected to develop critical thinking, problem-solving approaches, and innovation (Section 2.3). In addition, regulatory issues arise when LLMs are provided authorship or content generated by LLMs is placed in the research papers. Further, clear regulatory guidance is absent for LLMs and related tools on issues such as privacy, security, accountability, copyright violations, disinformation, misinformation, and other forms of abuse and misuse of LLMs and LLM-based tools (section 2.4).
Results of the Research
The paper asserts that the opportunities provided by the LLMs are worth considering by the academic institutions. Easing administrative work and reducing mundane work such as routine report analysis and writing literature reviews topped the opportunities provided by the LLMs. The research also asserts that bias is the biggest risk of these LLMs (section 4.2). However, every researcher will have a bias while conducting a scientific study; the best they can do is to reduce it by making the research replicable. This particular factor is not difficult for LLM developers to incorporate.
In conclusion, LLMs are deemed to be unfit for scientific paper authorship (section 4.4). The reason for that is the lack of accountability for the text it generates and the lack of transparency in how the responses are generated. If the algorithmic functioning is made for the researchers, the responses provided by LLMs could be incorporated directly into the research papers, citing the approach of the algorithm and the inputs. However, that might not be feasible as it makes little sense for a business entity as the user base becomes limited. Another highlighted issue is that overdependence on the LLMs might deter the researchers’ critical thinking capacity (section 4.4). The most concerning outcome is that the LLMs would push misinformation.
Between the lines
The constant engagement of the scientific community facilitates advancements in science. This can be observed mainly in research publications where fellow scientists perform blind peer reviews evaluating the validity and reliability of the research. With the help of LLMs, writing a research paper (not a research paper, but it looks like one) becomes easy, and such submissions to journals and institutions will take away the reviewers’ precious time. Further, they disturb the entire process of peer review and validation from the scientific community in the research publications.
The pain points identified by the research, like transparency and lack of specificity in the answers or outcomes of the LLMs, show that these are market openings. Companies should address these pain points if they are looking into the academic market. Text is the foundation for communicating scientific knowledge, and thus, there will not be any compromise on factual correctness, scientific methods, and procedural arguments. If LLMs are to be made for research, the following factors have to be incorporated:
- Citation (with proper format) of the source from where the argument is considered.
- Assist in thematically analyzing the text and detailing the method used. For example, the LLM should explain if it has used Latent Dirichlet Allocation and Named Entity Recognition.
- Offer an upload feature for research papers to generate a literature review, yet caution is warranted before directly incorporating it into research papers. Establishing a robust method to assess the credibility and dependability of these outcomes is essential. One approach could involve random sampling of research papers to verify if the arguments in the generated literature review align accurately. This verification process could even be automated to gauge the match percentage.
The basic factors will assist in the literature review for any scientific research. Further, an LLM model can be trained to provide philosophical and theoretical conversation to anchor the research to a particular theory.