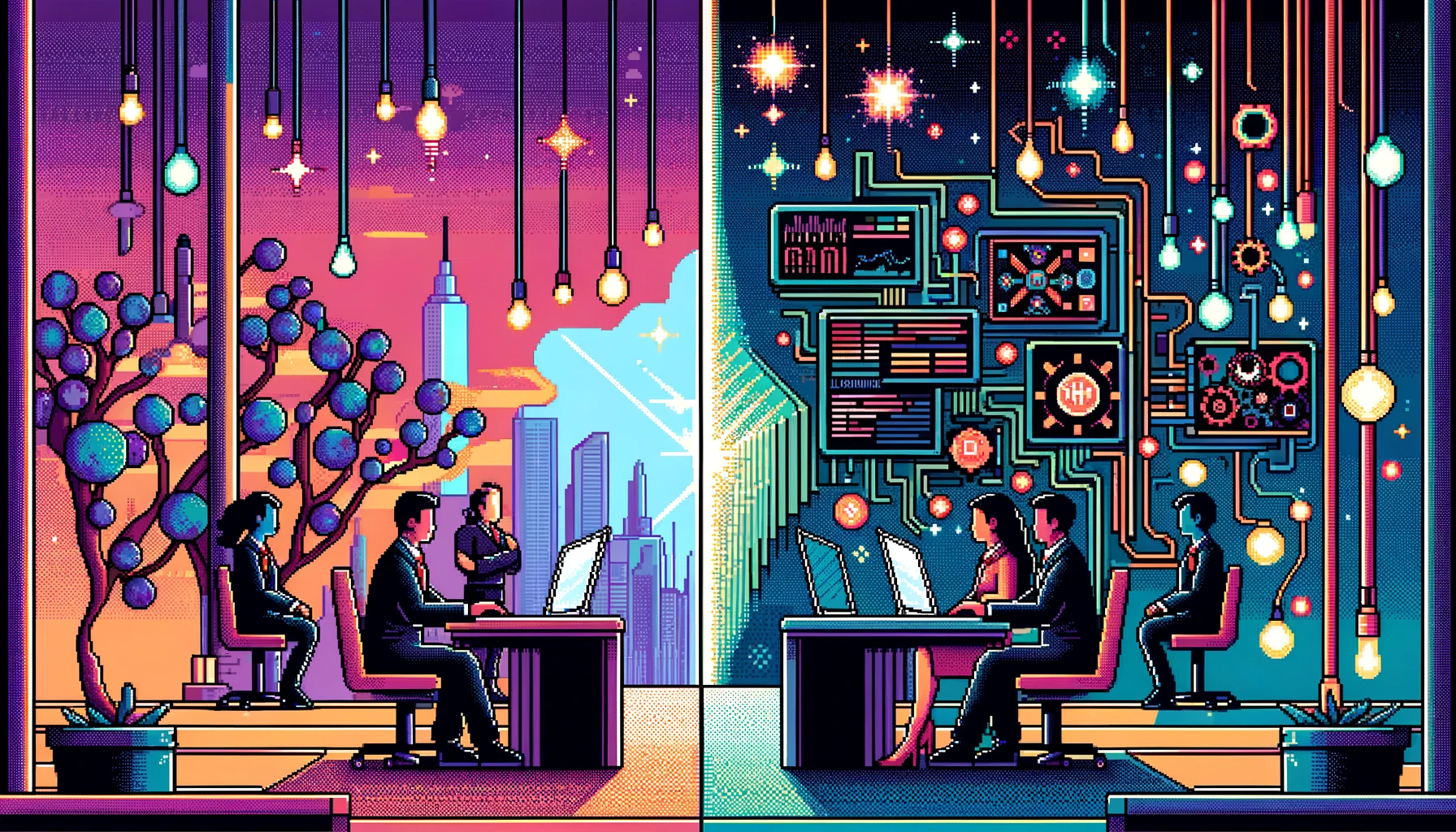
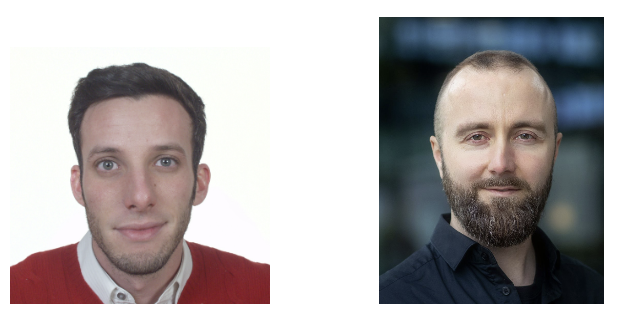
🔬 Research Summary by Alessandro Fabris and Matthew J. Dennis.
Alessandro Fabris is a postdoctoral researcher at the Max Planck Institute for Security and Privacy, studying algorithmic fairness and responsible data management.
Matthew J. Dennis is an assistant professor in the ethics of technology, working in the Philosophy & Ethics Group at TU Eindhoven.
[Original paper by Alessandro Fabris, Nina Baranowska, Matthew J. Dennis, Philipp Hacker, Jorge Saldivar, Frederik Zuiderveen Borgesius, and Asia J. Biega]
Overview: Employers are adopting algorithmic hiring technology throughout the recruitment pipeline. Algorithmic fairness is especially important in this domain due to its high stakes and structural inequalities. Unfortunately, most work in this space provides partial coverage, often constrained by two competing narratives, optimistically focused on replacing biased recruiter decisions or pessimistically pointing to routine discrimination of automation technologies. Whether, and more importantly, what types of algorithmic hiring can be less biased and more beneficial to society than low-tech alternatives currently remains unanswered, to the detriment of trustworthiness.
Introduction
Has artificial intelligence (AI) ever played a role in you getting a job? Does a society that uses algorithms to manage its human resources (HR) have a fairer hiring process? This is what a new wave of companies that have created AI hiring tools claim. Our article discusses algorithmic discrimination in hiring, focusing on its causes, measures, and possible remedies that consider a broader context of non-digital factors. Through a multidisciplinary analysis of the (otherwise scattered) literature on the topic, we show that many algorithmic recruitment tools tend to be more biased towards groups at the lower end of the socio-economic spectrum, as well as those who have been historically marginalized or who still suffer from structural inequalities. Furthermore, deploying algorithms in recruitment has often overlooked vital intersectional components, which means that job candidates who fall into more than one marginalized category can be discriminated against in ways that compound one another. This article aims to show how dire, complex, and rapidly evolving the problem is and point the way towards a better deployment of artificially intelligent tools in HR.
Key Insights
Sources of bias
Several factors in the hiring domain are likely to lead to biases in data and data-driven algorithms. This work presents over twenty, divided into three key categories. (1) institutional biases, including job segregation and elitism; (2) individual preferences hiding generalized patterns, such as job satisfaction and work gaps; and (3) technology blindspots such as advert delivery optimization and ableist technology.
Fairness flavours
Fairness in algorithmic hiring targets different constructs, including the following:
- Outcome fairness looks at predictions from the candidates’ perspective, measuring differences in their preferred outcome. This is the most common family of measures, including the 80% rule, a judicial rule of thumb applied in the US to ensure that the hiring probability is not too different across groups.
- Impact fairness relates algorithmic outcomes to downstream benefits or harms for job applicants, including application effort and expected salary.
- Accuracy fairness takes a closer perspective on the decision maker, requesting equalization of properties such as the groupwise average error.
- Process fairness is a notion that considers the equity of the procedure leading to a decision, regardless of its outcomes.
- Representational fairness relates to stereotyping and biases in representations. This is especially relevant considering the language of job descriptions.
The game is proxy reduction
Across many mitigating interventions to reduce biases and increase fairness in algorithmic hiring, the most common strategy is proxy reduction. It is based on reducing the influence of proxy features, i.e., features that are most strongly correlated with sensitive attributes. This approach aligns with legislation against disparate treatment (US) and direct discrimination (EU), which prohibit basing hiring decisions on protected attributes. It is, therefore, more appealing than post-processing or other approaches explicitly using sensitive attributes such as gender to modify algorithmic outcomes.
The Devil is in the Data
Missing data
Research on fairness in algorithmic hiring has a big problem of missing data for three distinct reasons. First, the employed datasets have low diversity: English is the dominant language, and US citizens are the most represented nationality. Second, the data only describe the early stages of the hiring pipeline, i.e., sourcing and screening, while neglecting selection and evaluation. Third, the data typically encodes gender and ethnicity as sensitive attributes. Disability, religion, and sexual orientation are simply missing, despite a long history of workplace discrimination, also due to strict data protection legislation.
Unstable targets
Target variables for algorithmic hiring models are very diverse and have different levels of face validity. Prediction targets encode different constructs (e.g., communication skills vs. commitment) annotated by people with different competencies (AMT workers vs. experts) from disparate data sources (YouTube videos vs. mock interviews). On the one hand, this reflects the length of hiring pipelines and the diversity of data sources. On the other hand, it points to a lack of established best practices around target variables in algorithmic hiring.
What’s good about videos?
Videos provide three types of signals: visual, verbal, and paraverbal. Across multiple studies, systems trained on video yield the largest disadvantages for protected groups. Visual signals have been removed from several products since they encode sensitive information like race and gender. They lack a solid foundation to justify their use in the hiring domain. Furthermore, speech and video processing often perform poorly for vulnerable groups, such as candidates with speech or vision impairments. Even if found to be accurate and fair in specific evaluation contexts, hiring algorithms based on face analysis are unlikely to predictably generalize and maintain accuracy or fairness properties. Finally, it is worth noting that recent regulation proposals (the EU AI Act, for example) are against the inference of emotions, states of mind, or intentions from face images in the workplace. This evidence invites particular caution before developing or deploying video-based systems for algorithmic hiring, even if accompanied by some form of bias mitigation and fairness evaluation.
Between the lines
Using data and algorithms in human recruitment comes with powerful advantages in removing traditional biases that still haunt non-digital hiring. Nevertheless, the promise of AI hiring technologies will be squandered unless urgent steps are taken to inform how they are currently deployed. Algorithmic fairness is indeed a key desideratum, but not enough on its own. We must augment it with a broader perspective.
One key factor that is consistently underestimated is how hiring algorithms do not operate in a vacuum. Instead, they are context-dependent tools that can precipitate other biases; they should be designed for recruiters and studied with human-machine interaction to better understand discriminatory effects. In addition, we need dedicated data curation efforts to study the impact of algorithmic hiring at every stage in the pipeline for vulnerable groups in different geographical areas. Furthermore, to avoid the risk of misguided fairness evaluations justifying unfit hiring tools, we should carefully scrutinize them through the lens of validity theory. Finally, we call for open conversations between policymakers and computer scientists, dialogues that guide and translate policy into responsible computing practices that practitioners can understand and adopt.