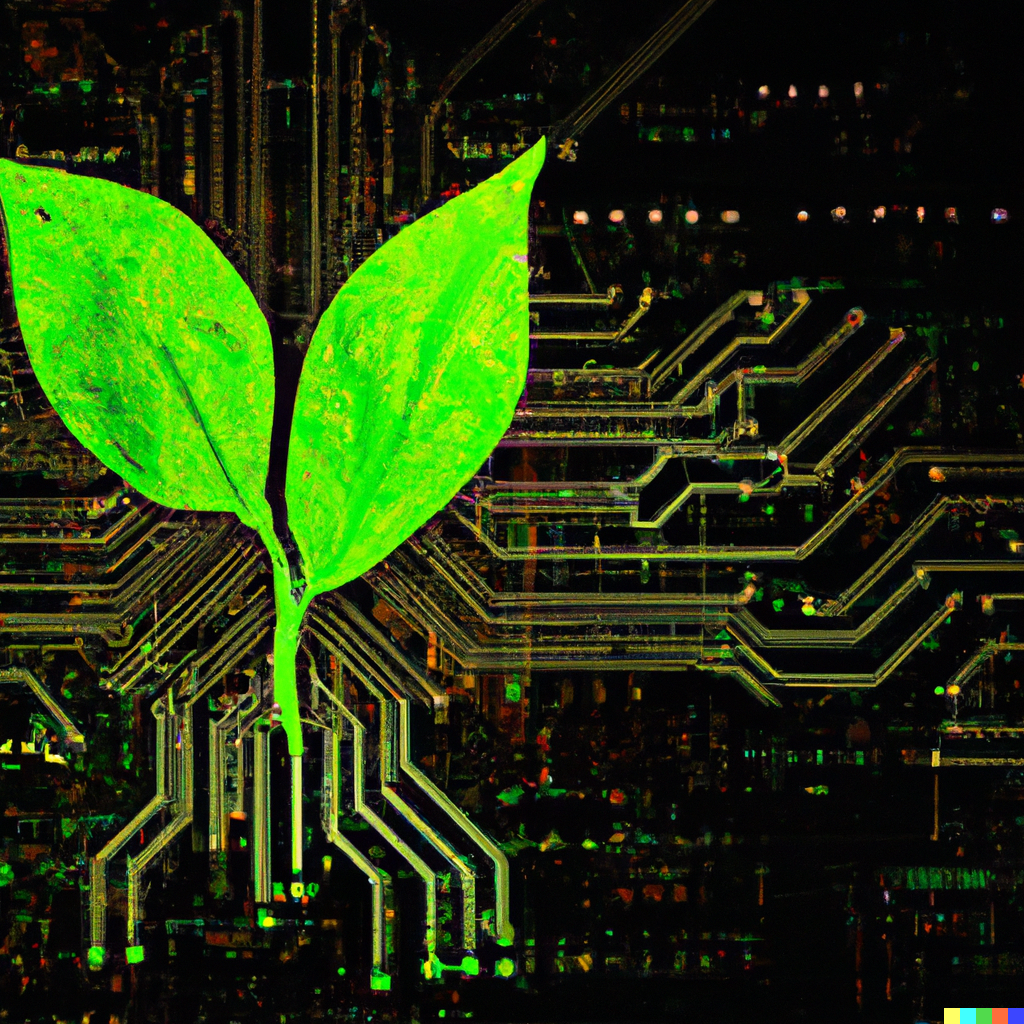
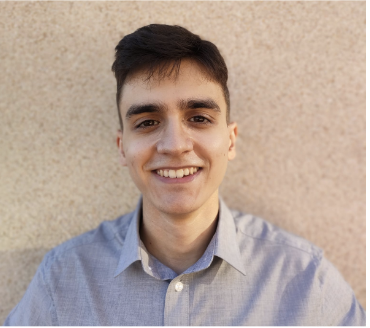
🔬 Research Summary by Joel Castaño Fernandez, a Data Science and Engineering undergraduate student and Research Assistant at the Polytechnic University of Catalonia working on Green AI on the assessment and study of ML carbon efficiency.
[Original paper by Joel Castaño Fernandez, Silverio Martínez-Fernández, Xavier Franch, and Justus Bogner]
Overview: The paper explores insights regarding the carbon emissions of machine learning models on Hugging Face, uncovers current shortcomings in carbon consumption reporting, and introduces a carbon efficiency classification system. It emphasizes the need for sustainable AI practices and improved carbon reporting standards.
Introduction
As the world collectively moves towards greener solutions, the impact of Artificial Intelligence (AI) on our environment has taken center stage. The energy-hungry nature of AI models during training and deployment has raised serious environmental concerns, leading to the emergence of Green AI – the development of AI systems with minimal environmental impact while maintaining performance. In this light, we analyzed the carbon efficiency of Machine Learning (ML) models on the Hugging Face platform, a popular repository for pre-trained ML models. Our research focused on understanding how carbon consumption is measured and reported and the influencing factors on carbon consumption during model training. We discovered a surprising gap in energy reporting practices and a minor decrease in reported energy consumption. We identified correlations between carbon emissions and various attributes, such as model size, dataset size, or performance metrics. For example, we found no substantial evidence of a relationship between model performance and energy consumption, suggesting that energy-efficient designs may not need to compromise on performance. Finally, we proposed an initial carbon efficiency classification system, providing a starting point for a more comprehensive evaluation of the carbon footprint of ML models.
Key Insights
In recent times, global attention towards sustainability has grown significantly. The technology industry is no exception, particularly with the increasing environmental impact of information and communication technologies (ICTs). The advent and rapid evolution of AI-based systems have heightened these concerns due to their increasing computational demands and energy requirements. The paradigm of Green AI aims to address these issues by developing AI systems that minimize environmental impact while maintaining performance. In this context, we analyzed the carbon emissions of various machine learning (ML) models and datasets on the Hugging Face, a prominent repository for pretrained ML models.
Our Approach
Our primary aim was to understand the carbon efficiency of ML models during their training phase. We divided our research questions into two parts:
- How is energy consumption measured and reported in the ML models on the Hugging Face Hub?
- What factors influence the carbon consumption of ML models during training?
What We Discovered
Delving deeper into the analysis, we uncovered various intriguing trends, correlations, and potential implications.
How Do Users Report Carbon Emissions?
Despite the escalating popularity of the Hugging Face platform, there is a surprising lack of improvement in the proportion of models reporting carbon consumption. This suggests that while this platform is widely used, there is a clear disconnect in emphasizing the importance of energy reporting practices among AI developers. This lapse could hamper the broader goal of creating sustainable AI systems, indicating a need for the AI community to increase awareness and implement energy reporting practices.
We noted a minor decrease in reported carbon consumption over the past few years. This trend, while modest, is indeed encouraging. It suggests that we may be making strides toward creating more energy-efficient models. However, it is crucial to remember that the existing energy consumption data is still sparse. Even so, this reduction could be seen as a sign of progress, an indicator that our efforts toward more efficient modeling are starting to bear fruit.
It was also interesting to note that natural language processing (NLP) models were the chief contributors to the carbon emissions reports, indicating the significant carbon footprint of these models. However, despite being fewer in number, computer vision models have demonstrated a higher propensity for emissions reporting within their domain in recent quarters. This could suggest that the computer vision community is perhaps more conscious about their carbon footprints, which could lead to the development of more energy-efficient algorithms in the future.
Upon analyzing the carbon reporting practices, as we already discussed, we were disappointed to discover that most models provide neither energy data nor context (e.g., the hardware used during training). This gap underscores the need for stricter guidelines and standards on energy reporting. However, there is a silver lining: about 75 models did report both carbon data and context, indicative of growing energy efficiency awareness among some model developers.
What Impacts Carbon Emissions?
Regarding correlations, our analysis revealed insufficient evidence to consider a relationship between carbon consumption and model performance. This finding, while interesting, calls for a cautious interpretation. It suggests that improved performance does not automatically equate to higher energy costs, and energy-efficient designs may not necessarily have to sacrifice performance. However, it is critical to note that the relationship between efficiency and performance in models is complex. It is an intricate dynamic that is not easily reduced to a simple binary, so more research is needed to explore this relationship fully.
Nevertheless, we found a clear link between model size and dataset size with energy consumption. Larger models and more extensive datasets invariably lead to greater energy consumption during training, underscoring the need for more efficient, compact model designs and optimized data management strategies.
Moreover, we found no substantial evidence that Machine Learning (ML) application domains, such as NLP versus Computer Vision, significantly impact carbon emissions. This could mean that the issue of energy consumption and carbon emissions in AI is universal and not confined to a specific domain, reinforcing the need for concerted efforts across the entire field to address these challenges.
Finally, we proposed an initial approach toward a carbon efficiency classification system to assess ML models’ energy consumption. This system sorts models into five categories, from “A Label” for those exhibiting high energy efficiency to “E Label” for those marked by elevated CO2 emissions and weaker performance. The final rating is obtained by considering multiple metrics, focusing on CO2 emissions reduction, size efficiency, model reusability, and performance integrity. It is a preliminary step towards a holistic method of evaluating the carbon footprint of ML models. We refer interested readers to our full paper for a more detailed discussion of this approach and its intricacies.
Between the lines
These findings underscore the pressing need for more comprehensive energy reporting practices and energy-efficient models in AI. The fact that energy consumption data is largely unreported, particularly in widely-used platforms like Hugging Face, suggests a significant oversight within the field. The marginal decrease in reported carbon consumption could be promising, albeit caution must be exercised in interpreting this as conclusive evidence of progress toward energy efficiency due to data sparsity. The lack of correlation between model performance and the energy consumption is intriguing and warrants further investigation, as it challenges the common perception that higher performance necessarily comes at a cost of higher energy usage. These results set the stage for future studies to delve into the complexities of this relationship. Additionally, the proposed carbon efficiency classification system, albeit preliminary, is a constructive step in recognizing and addressing the environmental impact of ML models, pushing the narrative of green AI forward.