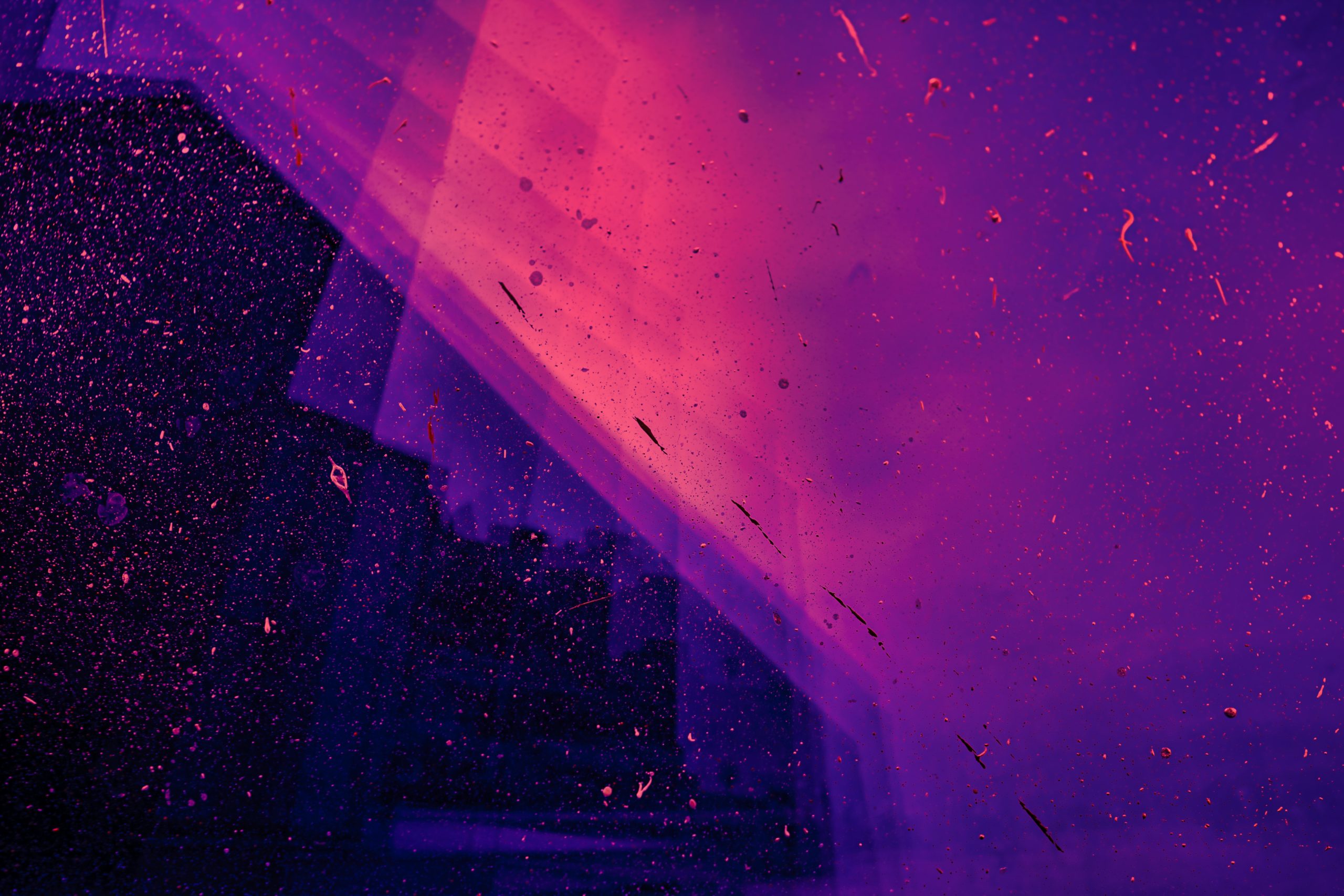
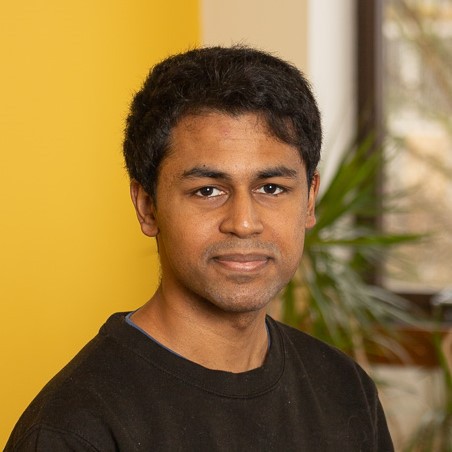
🔬 Research summary by Ashwin Acharya, an AI Governance and Strategy Researcher at Rethink Priorities.
[Original paper by Helen Toner and Ashwin Acharya]
Overview: Problems of AI safety are the subject of increasing interest for engineers and policymakers alike. This brief uses the CSET Map of Science to investigate how research into three areas of AI safety — robustness, interpretability and reward learning — is progressing. It identifies eight research clusters that contain a significant amount of research relating to these three areas and describes trends and key papers for each of them.Â
Introduction
Applying today’s machine learning systems in complex, high-stakes situations is a risky prospect. Safety-critical industries like aviation have a culture of caution when developing and deploying new automation. A growing list of AI accidents and incidents demonstrates the failure modes of machine learning systems, even as the upward trends on AI benchmarks demonstrate machine learning’s promise.
AI safety research aims to identify the causes of unintended behavior in machine learning systems and develop tools to ensure these systems work safely and reliably. The field is commonly divided into three categories:
- Robustness aims for guarantees that a system will continue to operate within safe limits even in unfamiliar settings;
- Interpretability seeks to establish that it can be analyzed and understood easily by human operators;
- Reward learning is concerned with ensuring that its behavior aligns with the system designer’s intentions.
By identifying and analyzing AI safety-related clusters of research in the CSET Map of Science — comprising more than 15,000 papers — this data brief investigates how research into these challenges is progressing in practice. It finds that research into these areas has grown significantly over time, and is led by researchers from the United States.
This brief makes use of CSET’s Map of Science to investigate what AI safety research looks like in practice so far. This resource was constructed by grouping research publications into citation-based “research clusters,” then placing those clusters on a two-dimensional map based on the strength of citation connections between them.
Operational definitions of AI safety categories
Robustness research focuses on AI systems that seem to work well in general but fail in certain circumstances. This includes identifying and defending against deliberate attacks, such as the use of adversarial examples (giving the system inputs intentionally designed to cause it to fail), data poisoning (manipulating training data in order to cause an AI model to learn the wrong thing), and other techniques. It also includes making models more robust to incidental (i.e., not deliberate) failures, such as a model being used in a different setting from what it was trained for (known as being “out of distribution”). Robustness research can seek to identify failure modes, find ways to prevent them, or develop tools to show that a given system will work robustly under a given set of assumptions.Â
Interpretability research aims to help us understand the inner workings of machine learning models. Modern machine learning techniques (especially deep learning, also known as deep neural networks) are famous for being “black boxes” whose functioning is opaque to us. In reality, it’s easy enough to look inside the black box, but what we find there—rows upon rows of numbers (“parameters”)—is extremely difficult to make sense of. Interpretability research aims to build tools and approaches that convert the millions or billions of parameters in a machine learning model into forms that allow humans to grasp what’s going on.
Reward learning research seeks to expand the toolbox for how we tell machine learning systems what we want them to do. The standard approach to training a model involves specifying an objective function (or reward function): typically, to maximize something, such as accuracy in labeling examples from a training dataset. This approach works well in settings where we can identify metrics and training data that closely track what we want, but can lead to problems in more complex situations. Reward learning is one set of approaches that tries to mitigate these problems. Instead of directly specifying an objective, these approaches work by setting up the machine learning model to learn not only how to meet its objective but what its objective should be.
Methodology
The authors identified clusters related to each topic using a combination of a keyword search for relevant terms and manual filtering based on a sample of papers in the cluster. They found three clusters related to robustness research, two related to interpretability, and two related to reward learning.
Trendlines
Robustness and interpretability research have grown at a much more rapid pace since roughly 2017. The United States leads in publication output for both categories. Chinese researchers are increasingly interested in robustness research, while the European Union has long been active in interpretability research.
Reward learning has also grown in recent years, but less explosively. The authors note that some work they grouped under “reward learning” applies to robotics work unrelated to deep learning, and that reward learning may generally be a fuzzier category that fits less neatly under the “AI safety” label.
Conclusions
Based on the research clusters identified here, it appears that work in all these areas is growing at a significant pace worldwide. The United States appears to lead in every area, with China showing substantial growth in robustness research and the EU producing a large amount of interpretability work.
Notably, despite the significant growth of the AI safety–related clusters analyzed in this paper, they still represent only a tiny fraction of total worldwide research on AI in general. The authors identified eight AI safety clusters containing a little over 15,000 papers; but when searching for AI literature in general, they find nearly 2,000 clusters containing over 1.9 million papers. These numbers imply that safety research may make up less than 1 percent of AI research overall.
The safety clusters identified show some promising progress on safety-related problems and may also point to some gaps. For instance, one could see the heavy emphasis on adversarial examples in robustness clusters in a negative light; perhaps the perceived prestige of that topic has led related problems to be relatively neglected.
Between the lines
These findings show that AI safety is becoming an increasingly active area of research, and is led by researchers from the United States. A particularly interesting trend is the growth of Chinese research into AI robustness (and, to a lesser extent, interpretability). Do Chinese researchers conceptualize of these areas, and of AI safety in general, in similar terms to their Western counterparts? Where do their views diverge?
The growth of AI safety work raises two important questions: what caused this growth, and how meaningful is it? The authors note some foundational papers for each AI safety field, but were those papers the key driver, or was progress driven by events such as the AI Asilomar Conference? And while AI safety work is growing rapidly, so too is work on AI in general. What do leading researchers and AI labs think of AI safety, and to what extent do they prioritize it when developing and deploying new technologies?