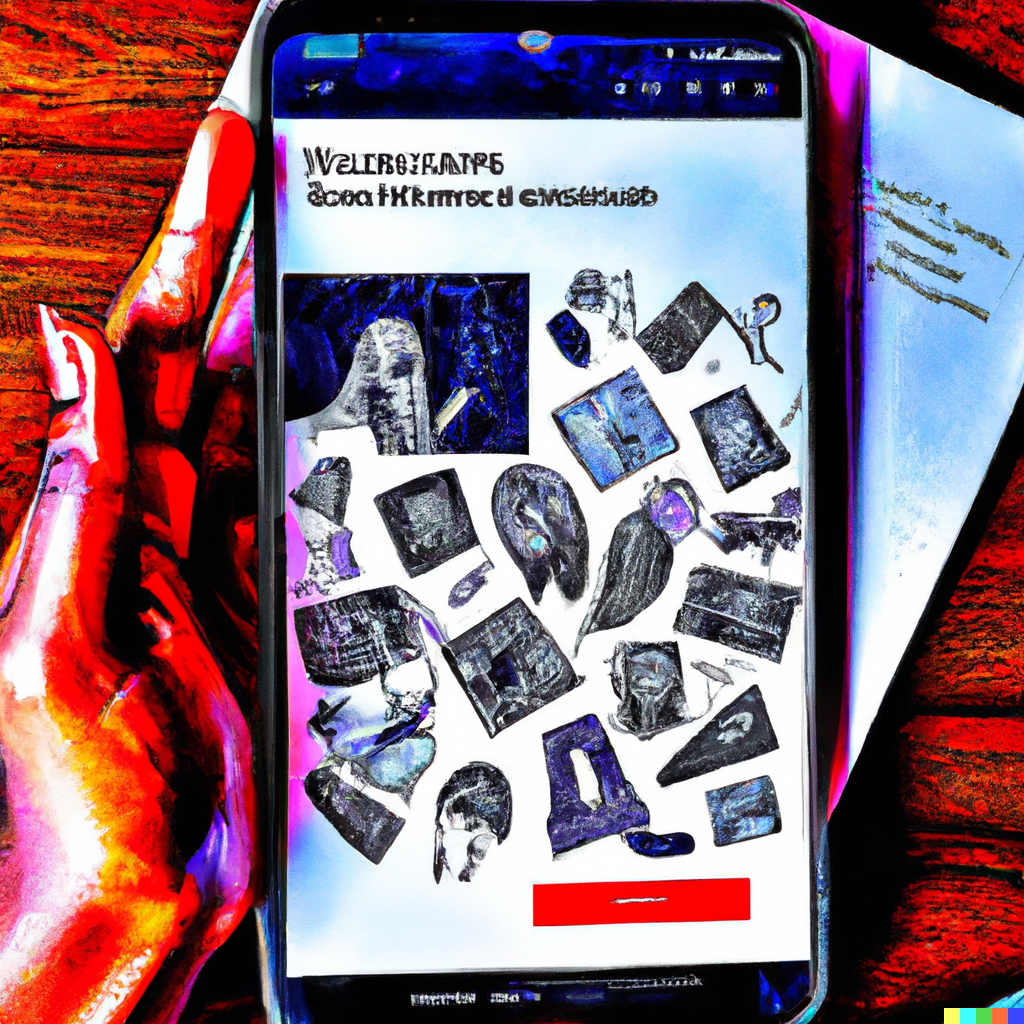
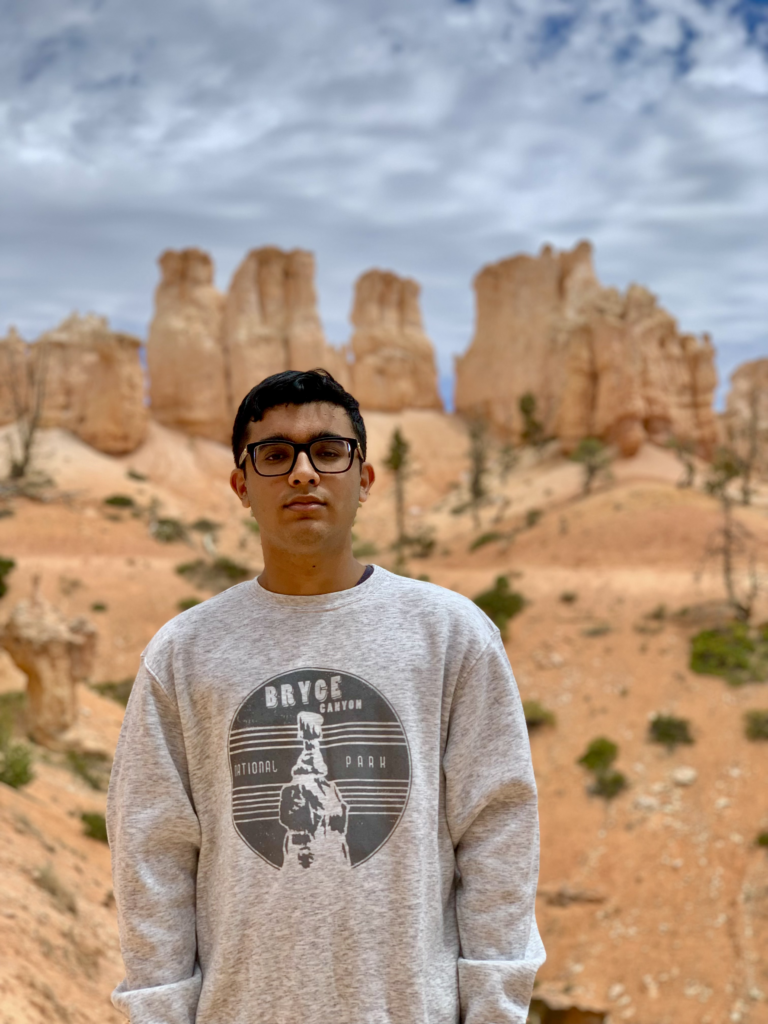
🔬 Research Summary by Amogh Joshi, a high school researcher at the Inf.Eco Lab at the iSchool of the University of Maryland.
[Original paper by Amogh Joshi and Cody Buntain]
Overview: The US Department of Justice and others have alleged foreign interference in US politics through campaigns of malevolent online influence. Our recent work assesses the visual media shared by disinformation accounts from four of these online influence campaigns: Iran, Venezuela, Russia, and China. Our models demonstrate consistencies in the types of images these campaigns share, especially as they pertain to political ideologies, with each campaign tending toward conservative imagery.
Introduction
Investigations into foreign influence campaigns show these efforts span various online platforms and modalities, from text to images. However, most of the research in this domain largely focuses on the text shared in these campaigns, leaving open questions about the role and use of media in manipulating online audiences.
Creating media may be more time- and resource-intensive than sharing links to news and amplifying other accounts. One such question is whether inauthentic accounts present a consistent persona across these modalities, as evidence shows multimedia drives more engagement than text sharing. How inauthentic actors use these modalities affects their detection and quality in the online information ecosystem.
Key Insights
Identifying Specific Image Types Shared in a Political Context
Images can generally be separated into several different types. E.g., you can often find images categorized based on their primary subjects, such as humans, objects, and landscapes, or the circumstance or event in which they are shared. To understand imagery in a political context, however, we develop a similar categorization of images shared by US politicians.
Images are a unique modality in that they can contain many features. To obtain the features of greatest importance from the images in our dataset, we use a pre-trained deep learning model (ResNet50) to create dense embeddings that characterize these images. ResNet50 is originally trained on the ImageNet object-detection dataset, which consists of common household objects to animals.
These embeddings are then used to train a model to cluster these images into eight types, which we broadly describe as belonging to the following categories: text-based documents (as in cluster 0), infographics and advertisements (as in clusters 6 and 7), Americana-style patriotic imagery (as in cluster 1), and various types of images featuring people (in clusters 2, 3, 4, and 5). Further analysis of these types can be found in our work at https://arxiv.org/abs/2110.01183.
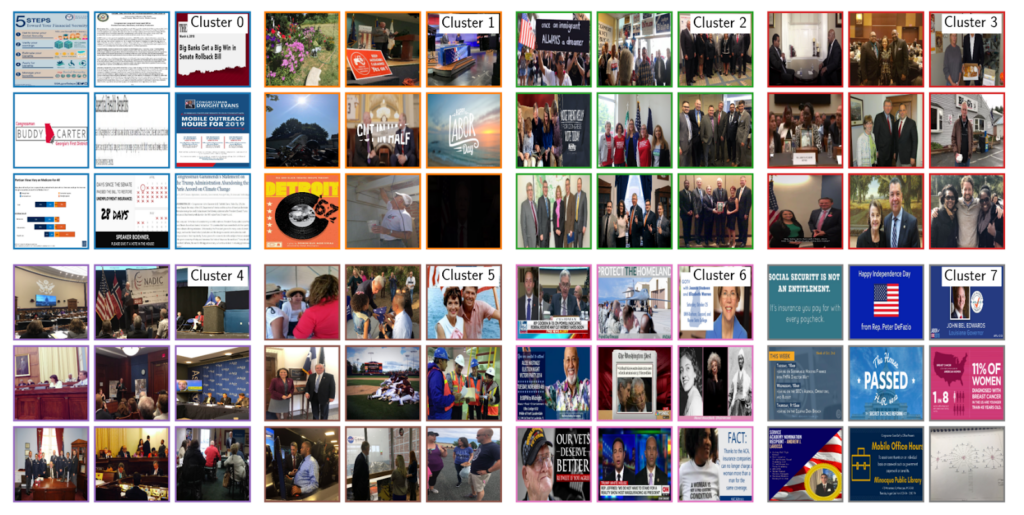
Correlating Imagery With Ideology
After identifying unique imagery types, we next determine whether a correlation exists between these image types and political ideology. To this end, we fit a linear regression model to the proportions of images US congressperson shares across each of these eight clusters, along with their corresponding political ideologies–using well-established ideology measures. Our results from this regression model demonstrate significant correlations between certain types of imagery and ideology–in particular, document-style imagery, infographics, and photos of groups of people are correlated with a more liberal congressperson. In contrast, patriotic and Americana-style imagery is associated with a more conservative congressperson.
With these results demonstrating a significant connection between visual presentation and ideological position, we next use these methods to assess the ideology of images used by foreign influence campaigns–extrapolating our conclusions regarding images shared in US political contexts.
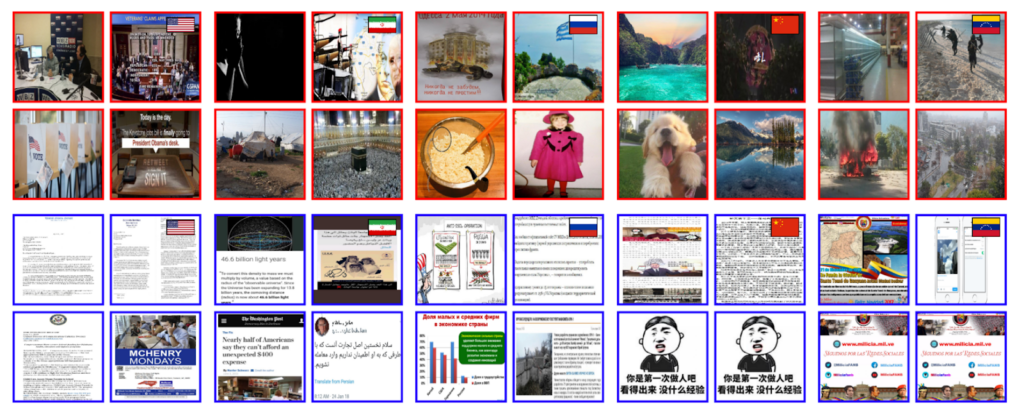
We develop a random forest regression model using the image types we have collected–for each influence campaign account, we sample images, extract features, and obtain the distribution of images they have shared in each cluster. Since this model collapses the number of input features to a small subset of types, we also use a model trained directly on the raw embeddings as a consistency check.
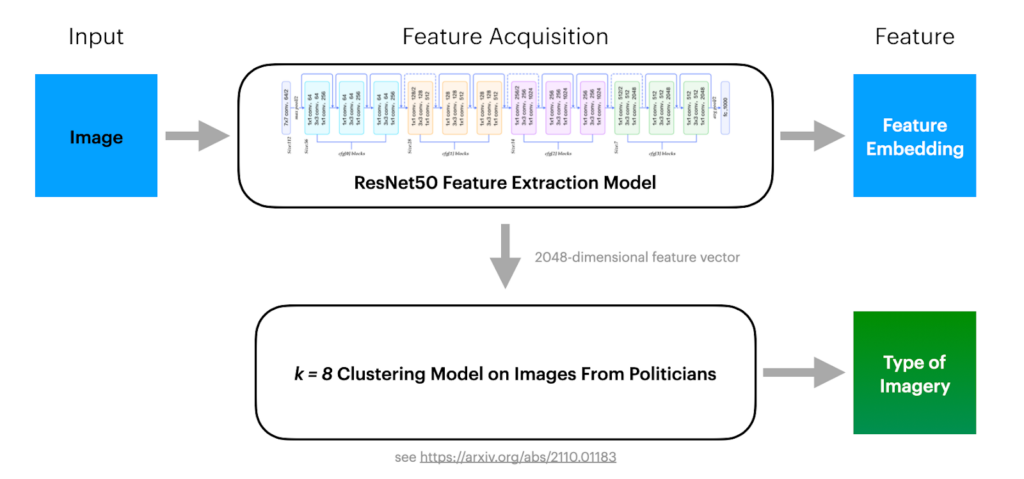
Influence Campaign Ideology Predictions
For this work, we present a slightly refined version of our raw embeddings model–we use embeddings from EfficientNetB0, a newer image characterization model, and we only use image types that correlate with political ideology (i.e., we discard images from clusters that have no significant ideological correlation), as determined by our k=8 clustering model. The model reveals that each of the four influence campaigns tends to lean moderately conservative, with most accounts–especially in Venezuela and Russia–having a conservative ideology.
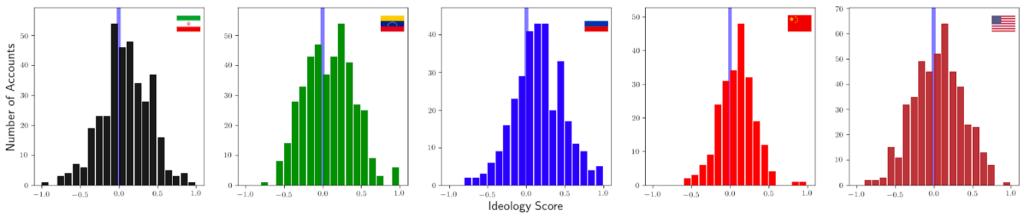
Between The Lines
This research demonstrates a correlation between the types of imagery shared by foreign influence campaigns and a conservative ideology. Our approach demonstrates the viability of using image characterizations alongside raw features when assessing imagery in a political context.
A notable limitation in using a model-based feature extraction approach, however, is modeling bias toward certain ideological positions. The off-the-shelf, pre-trained models used herein are trained in separate, apolitical contexts (i.e., object detection), suggesting the need for models trained in political contexts for a more suitable analysis of political discourse. Furthermore, additional analysis shows that our regression models, though generally performing well overall, underperform for conservative politicians relative to liberals. This effect may be attributed to the reduced diversity we observe in the imagery shared by conservative accounts.
This work suggests that imagery is increasingly relevant when assessing political influence campaign accounts. Prior work has suggested that Russian disinformation accounts are ideologically diverse in the text-based news they share. In turn, our image-oriented work provides new insights into coordination axes and further suggests that accounts may not necessarily present consistent ideological positions across different modalities, e.g., a caption and an image may have different meanings in different contexts.