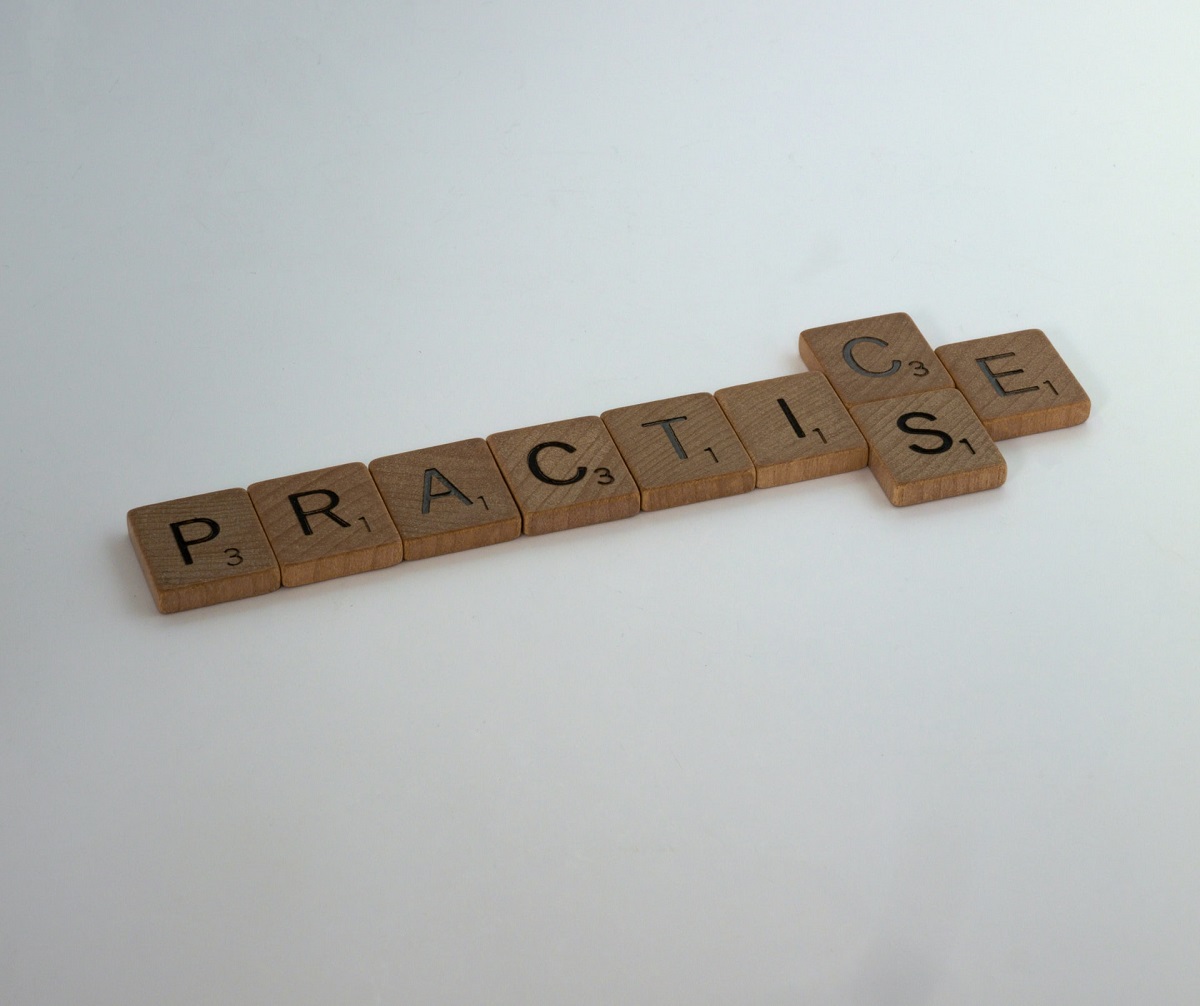
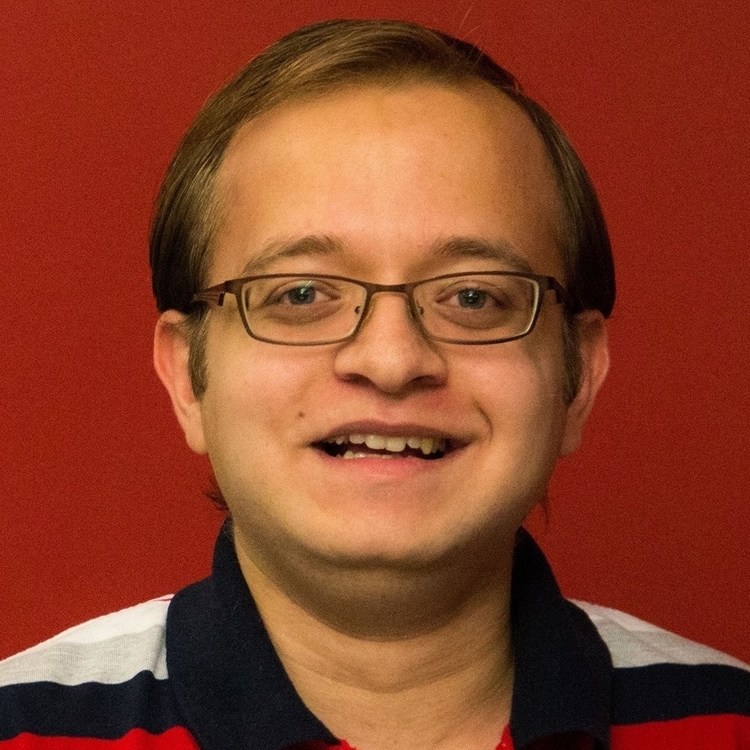
🔬 Research summary by Abhishek Gupta (@atg_abhishek), our Founder, Director, and Principal Researcher.
[Original paper by Daniel Schiff, Bogdana Rakova, Aladdin Ayesh, Anat Fanti, Michael Lennon]
Overview: As many principles permeate the development of AI to guide it into ethical, safe, and inclusive outcomes, we face a challenge. There is a significant gap in their implementation in practice. This paper outlines some potential causes for this challenge in corporations: misalignment of incentives, the complexity of AI’s impacts, disciplinary divide, organizational distribution of responsibilities, governance of knowledge, and challenges with identifying best practices. It concludes with a set of recommendations on how we can address these challenges.
Introduction
Have you found yourself inundated with ethical guidelines being published at a rapid clip? It is not uncommon to feel overwhelmed with many, often conflicting, sets of guidelines in AI ethics. The OECD AI repository alone contains more than 100 documents! We might also experience a gap in the actual implementation of these guidelines leaving much to be desired after several rounds of discussions. The authors attempt to structure these gaps into some common themes. They emphasize the use of impact assessments and structured interventions through a framework that is broad, operationalizable, flexible, iterative, guided, and participatory.
What are the gaps?
The paper starts by highlighting some initiatives from corporations outlining their AI ethics commitments. What they find is that these are often vague and high-level; in particular, without practical guidance for implementation and empirical evidence on their effectiveness, the claims of being ethical are no more than promises without action.
Starting with the incentives gap, the authors highlight how an organization should be viewed not as a monolith but as a collection of entities that have different incentives that may or may not be aligned with the responsible use of AI. They also warn people that companies might engage in the domain of AI ethics to ameliorate their position with their customers and to build trust, a tactic known as ethics shopping, ethics washing, or ethics shirking. Such an approach minimizes accountability on their part while maximizing virtue signaling. Thus, aligning the organization’s purpose, mission, and vision with the responsible use of AI can help alleviate this challenge, utilizing them as “value levers.”
AI’s impacts are notoriously hard to delineate and assess, especially when they have second- or third-order effects. We need to approach this from an intersectionality perspective to better understand the interdependence of these systems on the environment surrounding them. This is important because the harms from AI systems don’t arise in a straightforward way from a single product.
Thinking about these intersectional concerns requires working with stakeholders across disciplines but they come from different technical and ethical training backgrounds that make convergence and shared understanding difficult. Discussions also tend to focus sometimes on futuristic scenarios that may or may not come to pass and unrealistic generalizations make the conversation untenable and impractical. Within the context of an organization, when such discussions take place, there is a risk that the ethicists and other stakeholders participating in these conversations don’t have enough decision-making power to affect change. There is often a diffusion of responsibility laterally and vertically in an organization that can make concrete action hard.
Finally, there is now a proliferation of technical tools to address bias, privacy, and other ethics issues. Yet, a lot of them come without specific and actionable guidance on how to put them into practice. They sometimes also lack guidance on how to customize and troubleshoot them for different scenarios further limiting their applicability.
What an impact assessment framework can do
The authors propose an impact assessment framework characterized by the following properties: broad, operationalizable, flexible, iterative, guided, and participatory with brief explanations of each of these tenets. This framing also includes the notion of measuring impacts and not just speculating about them. In particular, contrasted with other impact assessment frameworks, they emphasize the need to shy away from anticipating impacts that are assumed to be important and being more deliberate in one’s choices. As a way of normalizing this practice more, they advocate for including these ideas in the curricula in addition to the heavy emphasis that current courses have on privacy and bias and their technical solutions. The paper concludes with an example about applying this framework to forestation and highlights how carbon sequestration impacts should also consider the socio-ecological needs, for example, those of indigenous communities.
Between the lines
It’s great to see frameworks that are centred on practical interventions more than abstract ideas. The gap between principles and practices today is stark and such an ontology helps an organization better understand where they can make improvements. We need more such work and the next iteration of such a research endeavour is to apply the ideas presented in this paper in practice and see if they hold up to empirical scrutiny.