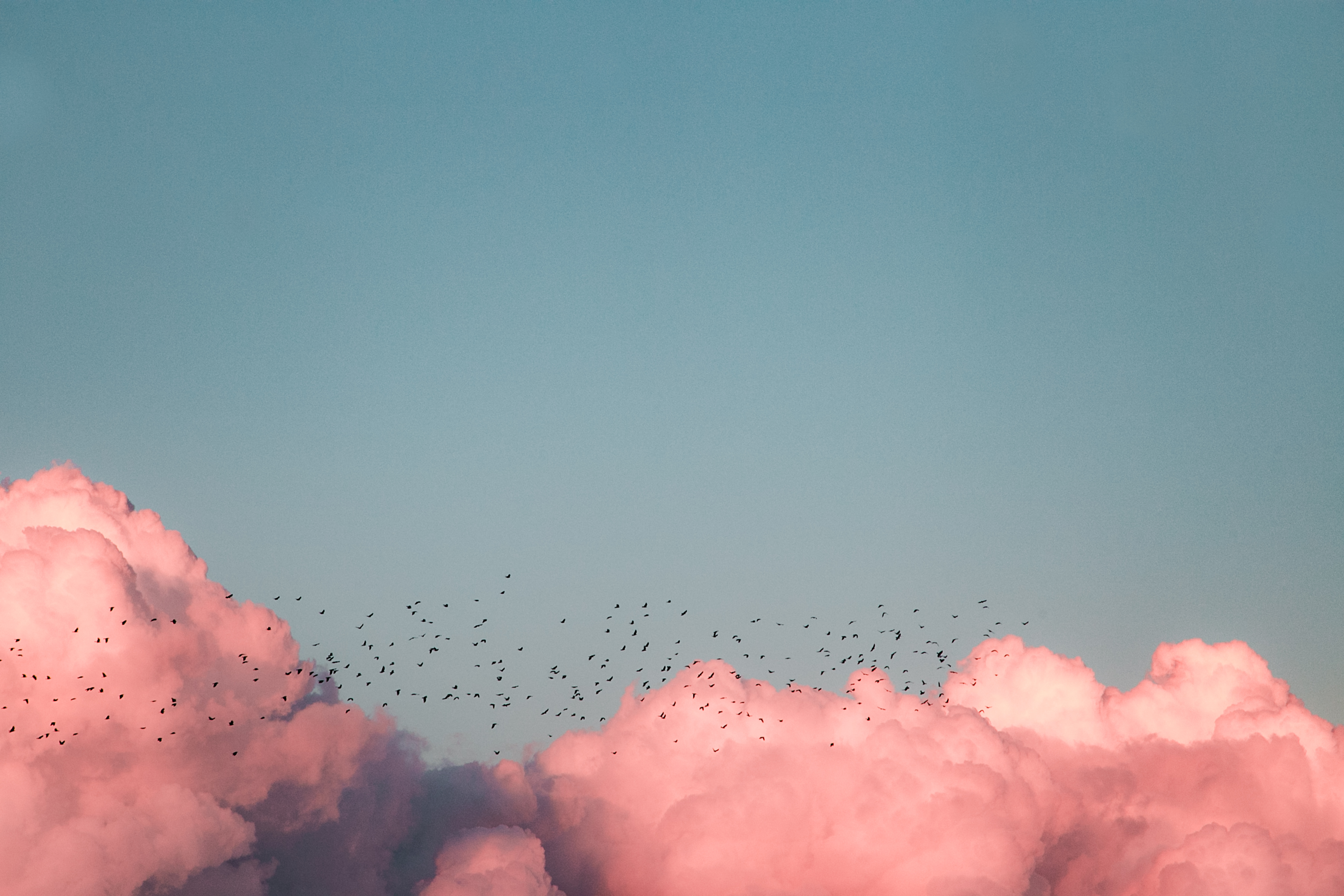
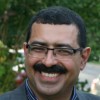
đŹ Research Summary by Ali El-Sharif, a college professor teaching data analytics and cybersecurity at the St. Clair Zemelman of School of IT in Windsor, Ontario. He earned his Ph.D. from Nova Southeastern University.
[Original paper by Daniel Vale; Ali ElâSharif; Muhammed Ali]
Overview: The paper argues that the use of post-hoc explanatory methods is useful in many cases, but that these methods have limitations that prohibit reliance as the sole mechanism to guarantee fairness of model outcomes in high-stakes decision-making. By way of an ancillary function, the inadequacy of European Non-Discrimination Law for algorithmic decision-making is demonstrated too.
Introduction
For high- stakes decision-making in the criminal justice, medicine, and banking domains, black-box machine learning models have generated unjustified social ills. Post-hoc explanation methods are a popular approach to gain insight into the logic of black-box models.
This paper demonstrates the limitations of post-hoc explain- ability methods in demonstrating prima facie discrimination. Post-hoc explainability methods lack the orientation towards illustrating outcome parity, which is essential for EU non- discrimination law. Moreover, their technical shortcomings mean that they are in some cases unstable and suffer from low fidelity. Subsequently, they cannot faithfully demonstrate the absence of discrimination.
Key Insights
Non-Discrimination Law: Legal Definitions
This paper discusses fairness and non-discrimination will be limited to a European legal perspective in terms of direct and indirect discrimination. Direct discrimination requires, more specifically, that (a) a protected class, (b) when compared to a non-protected class, (c) received less favourable treatment (d) based on the application of a criterion that (e) directly appealed to a prohibited ground of discrimination. Somewhat distinctly, indirect discrimination entails that (1) a protected class, (2) when compared to a non-protected class, (3) received less favourable treatment (4) based on the application of a seemingly (5) neutral criterion that does not directly appeal to a prohibited ground of discrimination, but indirectly so.
Mapping Model Outcome Bias & Non-Discrimination Law
A machine learning model is said to be discriminatory (hereafter âbiasedâ) in outcome if (1) group membership is not independent of the likelihood of a favorable model outcome (âType (1) Model Biasâ), or (2) under certain circumstances, membership in a subset of a group is not independent of the likelihood of a favourable model outcome (âType (2) Model Biasâ).
Post-Hoc Explainability Methods & Black-Box Models
Black-box models refer to automated decision systems that map user features into a decision class without exposing how and why they arrive at a particular decision. The internals of black-box models are either unknown or not clearly understood by humans. The terms black-box, grey-box, and white-box refer to the level of exposure of the internal logic to the system user – i.e., human examiners.
Post-hoc explainability takes a trained model as input and extracts the underlying relationships that the model had learned by querying the model and constructing a white-box surrogate model. Post-hoc explanations mimic model distillation as they transfer the knowledge from a large, complex model (the black-box model) into a simpler, smaller one (the white-box surrogate model). In doing so, they represent an estimated explanation of what the larger, complex model is doing, but not exactly how or why it arrived at a prediction. They, therefore, only generate an approximation of the functioning of the black-box model. Although this approximate explanation is not an exact match, it is often thought to be close enough to be useful in understanding the black-box modelâs logic.
Given the utility of post-hoc explainability methods, proponents of black-box models claim that, despite their complexity, sufficient interpretability can be generated to allow for human oversight post-model deployment. This, in turn, justifies their use for high-stakes decision-making. Any malfunctions, such as discrimination, can be detected and mitigated through renewed design. However, post-hoc explainability methods suffer pitfalls, which ought to substantively challenge this belief.
The first is that post-hoc explainability methods only approximate their underlying models. They are, therefore, not faithful and suffer from low fidelity). This runs the risk that the interpretability generated might inaccurately reflect feature spaces of underlying models. Second, post-hoc explainability methods suffer from instability. This is best demonstrated in the presence of uncertainty in Local Model-Agnostic Explanations (âLIMEâ) due to their randomness in sampling and procedure. Furthermore, some post-hoc explainability methods are permutation-based and make the incorrect assumption of feature independence which could generate misleading explanations.
Therefore, post-hoc explainability methods lack the orientation towards illustrating outcome parity, which is essential for EU Non-Discrimination Law. Moreover, their technical shortcomings mean that they are in some cases unstable and suffer from low fidelity. Subsequently, they cannot faithfully demonstrate the absence of discrimination (the null hypothesis). Finally, the limited bias types unearthed through post-hoc explainability methods mean that their use must be confined and contextually appreciated. The utility of post-hoc explainability methods is useful, especially in model design and development, but they are possibly limited for regulatory use. They, therefore, cannot be championed as silver bullets and/or can longer be appreciated alone in a void ignorant of broader fairness metrics.
Between the lines
The goal of this paper is to encourage legal practitioners and compliance officers to embrace a more holistic view of the inherit risks involved in deploying machine learning model in high stakes decision making and to recognize the insufficiency of some post-hoc explanation methods as the sole mechanism in achieving fairness, accountability, and transparency, where issues of non-discrimination ought to be of principal concern.