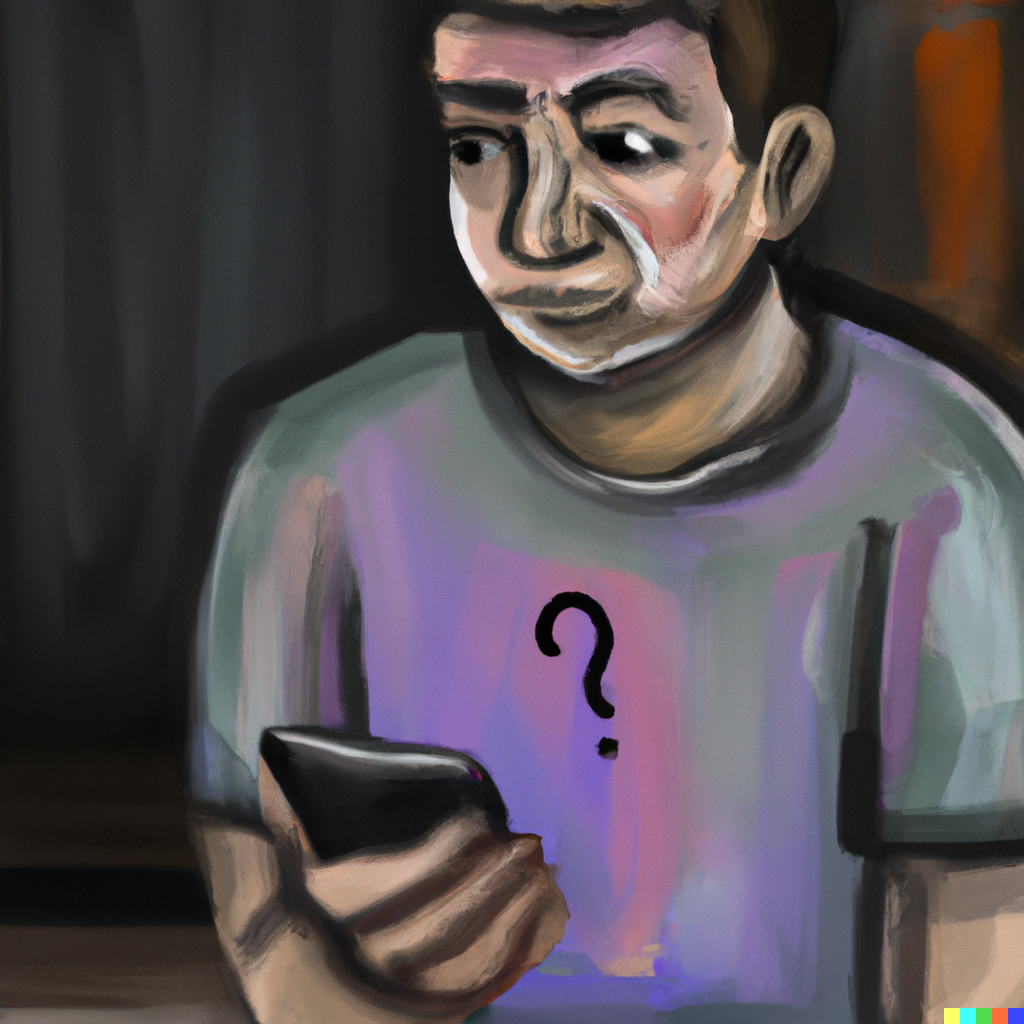
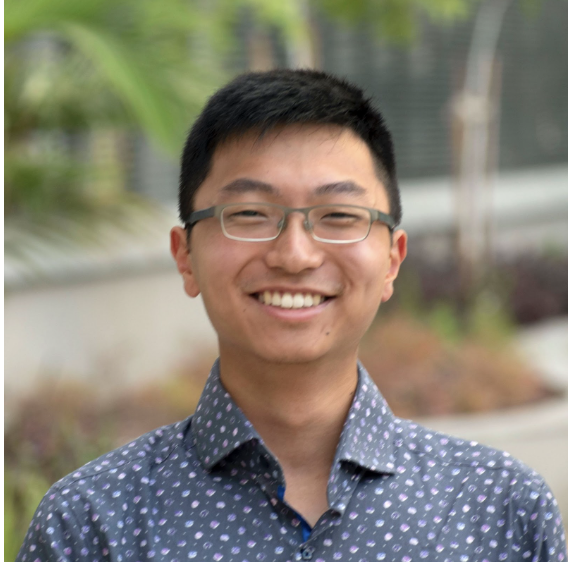
🔬 Research summary by Kevin Feng, a PhD student in human-computer interaction at the University of Washington, where his research focuses on enabling adaptive sociotechnical systems at scale through novel interfaces and interaction techniques.
[Original paper by K. J. Kevin Feng, Nick Ritchie, Pia Blumenthal, Andy Parsons, Amy X. Zhang]
Overview: Emerging technical provenance standards have made it easier to identify manipulated visual content online, but how do users actually understand this type of provenance information? This paper presents an empirical study where 595 users of social media were given access to media provenance information in a simulated feed, finding that while provenance does show effectiveness in lowering trust and perceived accuracy of deceptive media, it can also, under some circumstances, have similar effects on truthful media.
Introduction
Imagine scrolling through social media and seeing an image you think might be photoshopped, but you can’t be sure. This scenario is all too common in a world where media editing tools are increasingly powerful and accessible, especially with the rise of multimodal generative AI. Countless manipulated images and videos deceive users on social media every day. Fortunately, recent advancements in technical provenance standards—such as the C2PA standard—offer a promising solution. However, there has been little research on how users perceive media provenance information in social media settings and how it changes their credibility judgments.
To investigate, we conducted an online experiment with 595 social media users from the US and UK. We found that provenance information often lowered trust and caused users to doubt deceptive media, particularly when it revealed that the media was a composite of two or more images or videos. We also found that whether the provenance information itself could be trusted significantly impacted participants’ accuracy perceptions and trust in media, leading them, in some cases, to disbelieve honest media. We discuss how design choices may contribute to provenance (mis)understanding and put forth implications for usable provenance systems in practice.
Key Insights
Experimental setup
We used a repeated-measures study to measure the impact of provenance information on trust and accuracy perceptions of image and video content. 595 participants who were regular social media users based in the US and UK were recruited via Prolific, a crowdsourcing platform. Following some preliminary questions about social media use, our participants completed two rounds of a media-rating activity using mock Twitter feeds we built. The first round was done on a standard feed. In contrast, the second used a provenance-enabled feed where media provenance information could be accessed via clickable UI elements (see Fig. 1). The content seen by any one participant remained consistent across the two rounds. We also tested different UI design variations by creating feeds with varying designs and randomly assigning them to participants.
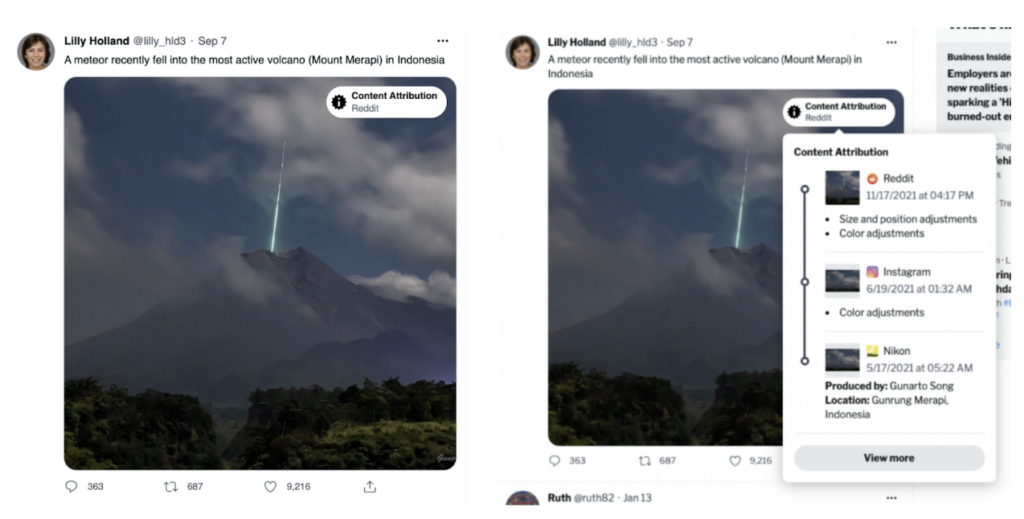
Fig. 1: Example of a UI that provided media provenance information from our study. Clicking on the button on the top right corner of the image would open up a panel with provenance details.
The media we used in the study was intentionally selected to cover a range of topics, including both deceptive and honest content, and had varying degrees of editing. Participants were asked to rate each piece of media they saw on two dimensions via a 5-point Likert scale: trust (how much they trusted the image or video to provide reliable information) and perceived accuracy (agreement with a claim about what the image or video depicted). We finally collected all ratings and quantitatively analyzed them via a series of statistical tests, as well as open-ended feedback, which we analyzed through qualitative coding.
Did provenance make a difference to credibility perception?
In short, yes. We found statistically significant differences in trust and perceived accuracy in media ratings across the no-provenance and provenance-enabled rounds. Specifically, trust and perceived accuracy tended to decrease after viewing provenance information. Trust and perceived accuracy dropped more significantly for content that underwent heavy editing than content with lighter or no editing, indicating that participants changed their judgment after accessing provenance details.
Now that we know provenance significantly impacts media perception, is this impact productive? Or can it mislead users even more? We calculated a metric we call correction for changes in perceived accuracy; correction is the distance the user’s perceived accuracy rating for a piece of content moved closer to that content’s ground truth accuracy rating. We found a positive correction in most cases, meaning that provenance information successfully led users closer to understanding what an image or video truly depicted. However, there were a minority of cases where the correction was negative. These cases often featured honest media, indicating that provenance can cause users to perceive the content as less accurate than it actually was.
What was the impact of provenance states on perception?
In our study, provenance could take on one of three states: normal, incomplete, and invalid. Normal indicated that the provenance information was complete and verified. An incomplete state indicated missing information, possibly due to editing the media with a tool that did not support provenance standards. An invalid state meant that the provenance information has been deliberately tampered with and should, therefore, not be trusted (see Fig. 2).
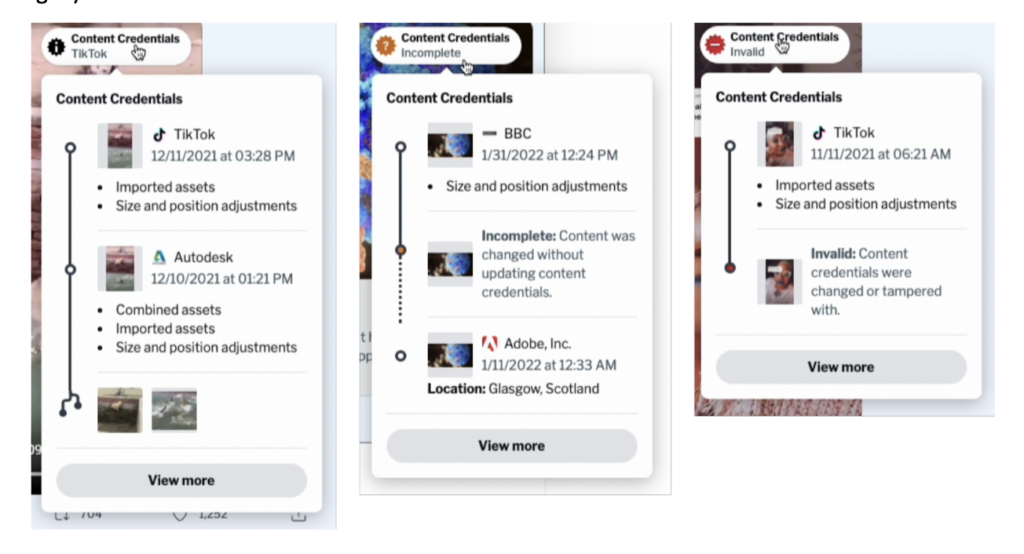
Fig. 2: the different provenance states shown in our study’s UI.
We found that the provenance state significantly affected the trust and perceived accuracy of media. Participants who saw incomplete provenance states generally trusted the media less and perceived them to be less accurate—sometimes at the expense of negative correction (moving farther away from the media’s ground truth)—than those who saw the same media in a normal state. The same effect was further amplified for invalid states. This points to the blurriness of the line between content credibility and provenance credibility. An authentic, unedited image may bear an incomplete state due to improper updating of provenance by the original creator. Should it be perceived as less trustworthy and accurate? Articulating connections between content and provenance credibility remains a tricky, open-ended issue.
What feedback did participants have for the provenance UIs?
Media ratings aside, we also received many pieces of open-ended feedback about the provenance UIs from our study. Some pointed out that certain terms within the UI should be more clearly defined, especially those that described the types of edits being made. Others wanted to learn more about particular edits and suggested a separate detailed pop-out view. Some suggested using color in certain UI parts to attract attention to potentially untrustworthy content. However, it’s also important to note that provenance is not meant to be prescriptive. Instead of telling users what to trust, we want to empower users to make informed decisions about credibility in their viewing contexts. Overall, many responded positively to the provenance UIs and expressed excitement at seeing them implemented in online platforms.
Between the lines
Today, many state-of-the-art machine learning techniques for detecting visual information offer a reactive approach: deceptive media is first posted, and then the system flags it. Provenance standards are one proactive approach to tackling this problem by embedding provenance information securely in media metadata in tandem with content creation. We see great potential for provenance to empower users to make more informed decisions about the credibility of the media they see.
That said, many questions beg to be solved before provenance standards are deployed on social media platforms. First, not all edits are created equal. Some edits may change a small number of pixels but can significantly alter the meaning of an image, like smoothing out an important detail. How do we identify and highlight these consequential edits from the rest? Also, users were unsure how to separate the orthogonal (albeit related) concepts of content credibility and provenance credibility. How do we encourage users to differentiate the two, and what kinds of user education measures are needed? We hope progress toward answers can extend our work and pave the way for the successful deployment of usable provenance online.