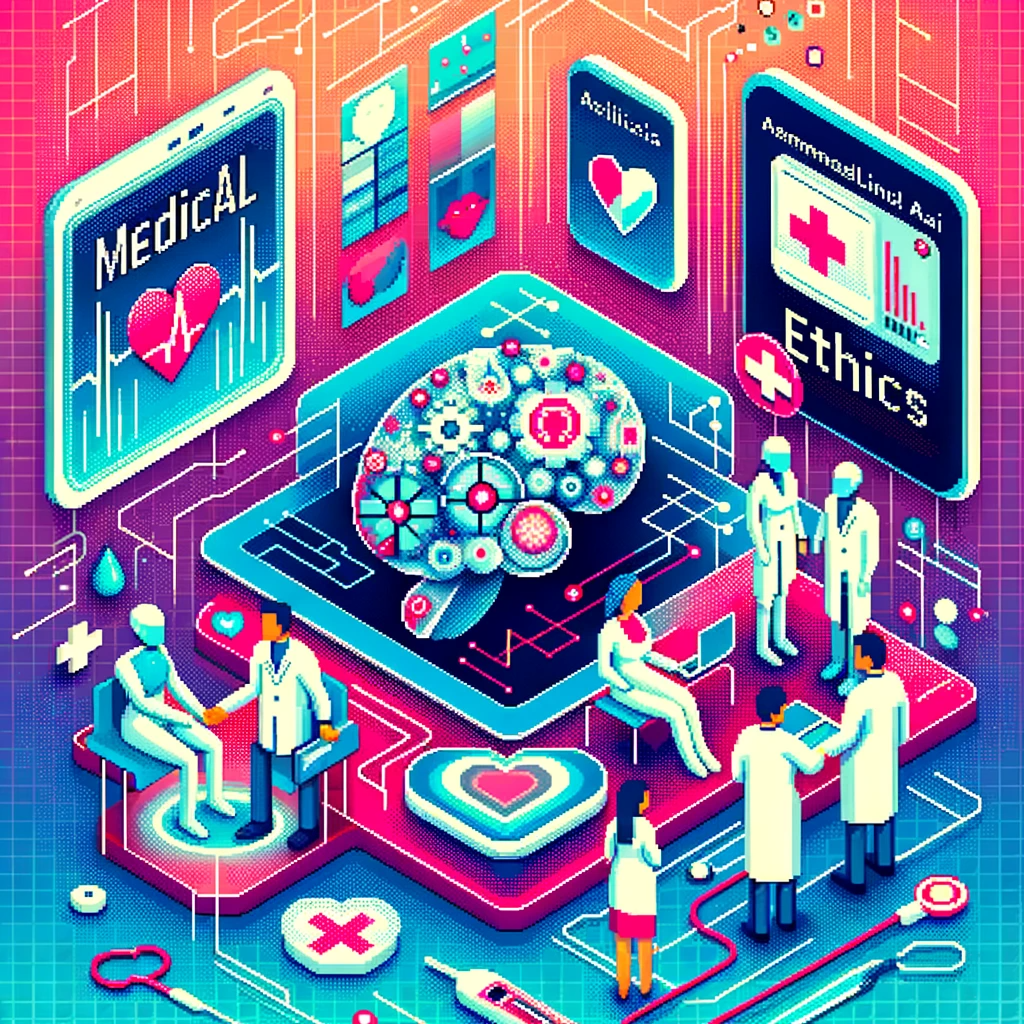
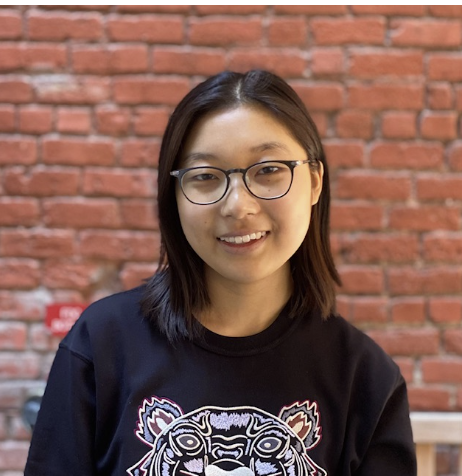
🔬 Research Summary by Sofia Woo, a recent graduate from McGill University who studied History and Political Science, emphasizing scientific history and political economics.
[Original paper by Jie Zhang and Zong-Ming Zhang]
Overview: The increased use of medical AI in clinical settings has set off concerns surrounding the ethics and trustworthiness of these tools. This paper uses a multidisciplinary approach to analyze the factors that influence trustworthiness in medical AI at the design and application levels and offer governance recommendations.
Introduction
Can we trust machines with our medical data—one of our live’s most personal and private aspects? As AI is becoming increasingly utilized in medical institutions to assist clinicians in diagnosing and treating patients, this has added a new dimension to bioethics. This topic is analyzed through a multidisciplinary approach on the design level (which refers to the technology’s reliability) and on the application level (which refers to the human impact on the use of AI). This paper frames the issue of AI and bioethics through the lens of technology, law, and healthcare stakeholders. In tackling these issues, the authors focus on five subjects that affect medical AI’s trustworthiness: data quality, algorithmic bias, opacity, safety and security, and responsibility attribution. They found that while AI systems have been shown to streamline treatment processes successfully, they are limited in terms of being only as good as the quality of data provided to them. As data given to medical AI tools is at times non-standardized, unstructured, and contains errors, the authors propose measures to institute AI tools that are more ethical and trustworthy.
Key Insights
The Importance of Data Quality
Because medical AI tools retrieve information via the data that clinicians input, a significant factor influencing just how trustworthy and accurate these machines are depends on how high quality the data is. It should be emphasized that it is not just data accuracy and representativeness but data annotation that is crucial. The paper highlights that it is the most frequently inconsistent data in the annotation process that poses the most problems. For example, differences between doctors’ biases and formats for labeling data and discrepancies between hospitals’ equipment and software have resulted in confusion and conflicting data being fed to medical AI systems.
The Two Sides of Algorithmic Bias
There are two aspects to algorithmic bias: human-induced and data-induced. Human-induced bias is defined as being intentional or unintentional, and these biases (like data-induced ones) are often reinforced and amplified as new iterations of algorithms accumulate past data. The data is unrepresentative and/or insufficient in the case of data-induced bias. While there are ways to combat algorithmic biases (such as taking measures to ensure that the data is diverse and has been reviewed before feeding it to medical AI tools), the fact that deep learning is a black box makes biases easily undetectable—thus making it easier to deliver discriminatory treatment (whether it is intentional or unintentional).
Opacity and Lacking Information
Connected to deep learning is the concept of opacity. The machine’s opaqueness creates a lack of understanding among users. In turn, this negatively affects how patients and clinicians view AI tools and could potentially establish distrust toward clinicians who use said tools. The paper outlines three main reasons opacity exists: algorithms are trade secrets, the algorithm is complex, and lay people cannot understand it. Explainable AI (XAI) has been proposed as a possible solution. However, it is important to note that there is often an inverse relationship between performance and machine transparency, with the highest-performing AI tools usually being the least transparent.
The Issue of Safety and Security
Risks involving machine errors, cybersecurity, and the constant need for adequate testing are other crucial aspects of medical AI trustworthiness. Blind spots in data (as explained above), hacking risks, and problems of how to go about treatments when the machine and clinician have conflicting views are all ethical issues that need to be addressed.
An Independent Entity?
With the above four aspects in mind, whether AI is an independent legal responsibility entity subject to liability is an ever-evolving debate. While current medical AI tools do not yet carry their own consciousness, what should institutions and AI developers do when the machines reach this point? The paper asserts that technology should serve to advance human society. As such, machine autonomy should not forgo the need for human subjectivity. In other words, the authors argue that measures should be taken to prevent the development of fully independent AI systems that can function well beyond human control.
Incorporating Bioethics Into Medical AI
Bioethics principles must be applied for medical AI to gain clinician and patient trust. One of the biggest issues is bridging the gap between promising ethical tools and actual applications. The authors propose embedding ethical algorithms into AI systems. However, regardless of which approaches are taken to embed these algorithms, the paper suggests that developers and clinicians should factor in the nuances of different medical cases rather than establishing universal ethics guidelines.
The authors argue that countries should add liability fees to the selling prices of AI tools. Additionally, they promote establishing a government or industry-led insurance and reserve system with public and private parties paying for the fees and forming an independent pool of funds to pay legal liability for medical AI tools.
With regard to data, hospitals should make strides in better structuring and standardizing data annotation to provide less biased medical treatments and recommendations. Hospitals, AI developers, and the government should work closely to ensure safe and responsible technology is used. If the government establishes an AI oversight committee, medical AI tools can be better regulated—especially in cases where algorithms are updated (thus requiring new regulatory policies).
Between the lines
Incorporating bioethics principles into medical AI algorithms is undoubtedly a crucial aspect of creating trustworthy technology that serves to advance society. While the paper highlights multiple critical topics that should be considered and offers robust solutions, gaps and questions remain. More research needs to be done regarding when these AI tools are considered to be conscious of their own actions and, by extension when liability is placed on humans or the machines. Additionally, while the authors’ proposed solution of instituting liability fees and other systems between public and private parties is an interesting point to consider, it may be difficult to establish in countries such as the United States, where the healthcare system is incredibly disjointed. Additionally, in places with many competing private healthcare companies, it should be considered that these parties do not necessarily have patients’ best interests at heart. Instead, they tend to prioritize profits over patient well-being—thus adding another obstacle to ensuring ethical medical AI is instituted.