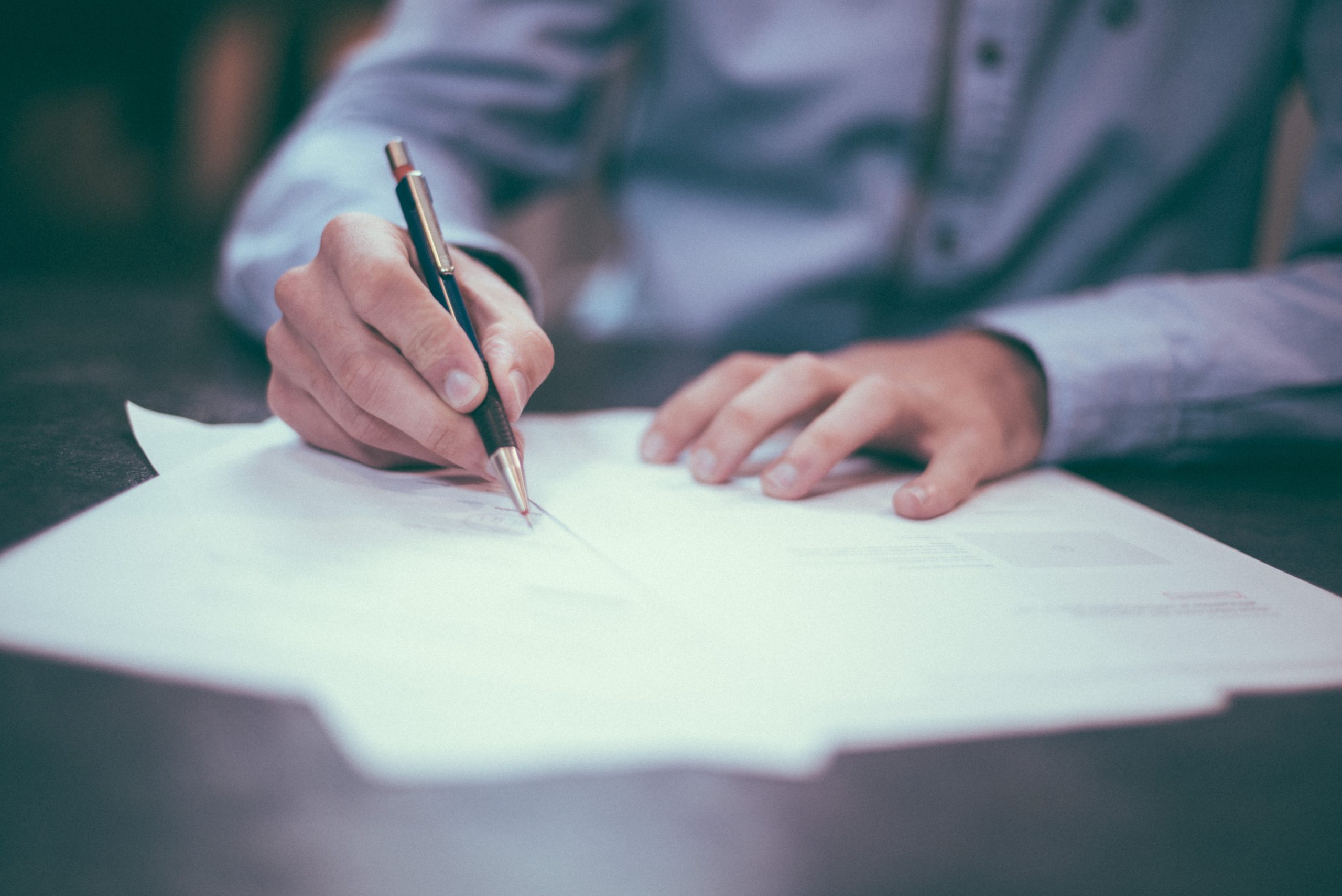
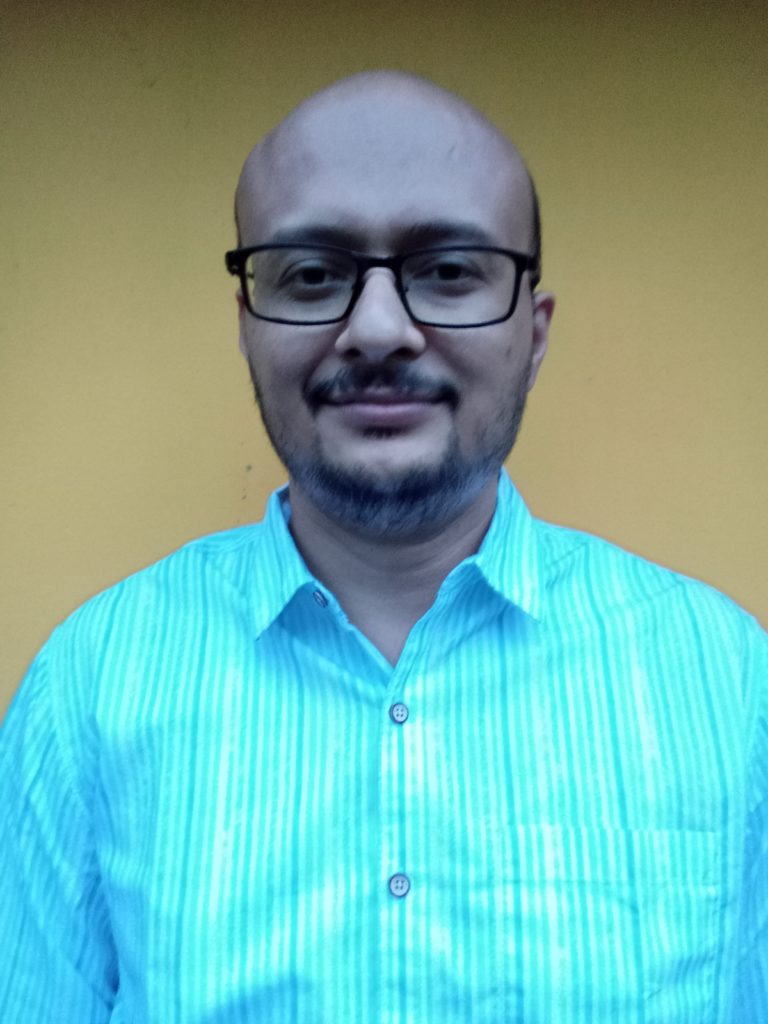
š¬ Research summary by Angshuman Kaushik, Researcher in AI Policy, Governance and Ethics.
[Original paper by Joachim Roski, Ezekiel J. Maier, Kevin Vigilante, Elizabeth A. Kane and Michael E. Matheny]
Overview: Ā The trust of users in AI systems used in the healthcare sector is waning as the systems are not generating their publicized breakthroughs. Given such a scenario, this paper describes a process for how a diverse group of stakeholders could develop and define standards for promoting trust, as well as AI risk-mitigating practices through greater industry self-governance.Ā
Introduction
AI systems can be used to derive value from exponentially growing health data. According to the researchers, the expectations of stakeholders are high for AI technologies to effectively address contemporary health challenges. However, prior periods of AI enthusiasm were followed by āAI Winters,ā where AI investment and adoption withered. In fact, they also warn about the risk of another āAI Winter,ā if current promises for AI solutions are not met by adequate performance. Recent examples underlining the growing disquiet over inappropriate AI outcomes include racial bias by algorithms in healthcare decision-making, poor performance in cancer diagnostic support etc. According to the researchers, such risks may be considered a āpublic risk,ā denoting threats to human health or safety. They also refer to a report by the National Academy of Medicine (NAM), where the authors detailed early evidence for promising AI solutions for use by patients, clinicians, administrators, public health officials and researchers. This paper expands on that work by identifying 10 groups of widespread AI risks and 14 groups of recently identified mitigation strategies aligned to NAMās AI implementation life cycle. It also describes how AI risk mitigation practices can be promulgated through strengthened industry self-governance, specifically through certification and accreditation of AI development and implementation organizations. The aforementioned NAM report on AI & Health describes a four-phased AI implementation life cycle that serves as an organizing schema to understand specific AI risks and mitigation practices. The four-phases are as follows;
- Phase 1 ā Needs Assessment;
- Phase 2 ā Development;
- Phase 3 ā Implementation; and
- Phase 4 – Maintenance
Industry Self-Governance Programs
The researchers stress on the fact that evidence-based AI risk mitigation practices should be more widely implemented by AI developers and implementers which can be ensured through government regulation of AI. Additionally, they can also resort to industry self-governance. The paper differentiates industry self-governance from organizational self-governance. The latter refers to the policies and governance processes that a single organization relies on to provide overall direction to its enterprise, guide executive actions and establish expectations for accountability, which is unlikely to effectively ameliorate AI risks. On the contrary, relying on industry self-governance in defining and monitoring adherence can offer several advantages. It has the capacity to act faster and with greater technical expertise than the government in defining and enforcing standards for products and services. Further, it may also be more insulated from partisan politics, which can lead to legislative or regulatory deadlocks. The paper refers to industry self-governance in the US healthcare sector such as, National Committee for Quality Assurance (NCQA) accreditation, ISO9000 certification, Baldridge awards etc. According to the researchers, to counter growing mistrust of AI solutions, the AI/health industry could implement similar self-governance processes, including certification/accreditation programs targeting AI developers and implementers.
Implementing industry self-governance programs
The paper describes essential steps for the implementation of an AI industry self-governed certification or accreditation program, which are listed below;
- Multi-stakeholder Participation – Self-governance efforts requiring trust by a broad set of stakeholders must incorporate multiple perspectives. Stakeholders may include patients, clinicians and institutional providers, AI developers, relevant governmental agencies etc. They could be effectively convened by an independent third-party organization that has expertise in the field and enjoys the trust of all stakeholders. A governing board of this organization should include representatives of all critical stakeholder groups. One example of an independent third-party organization cited in the paper is the Institute for Electrical and Electronics Engineers (IEEE) which has recently launched a Global Initiative on Ethics of Autonomous and Intelligent Systems and issued an iterative playbook of standards and best practices called āEthically Aligned Design,ā which is intended to inform governments, organizations, businesses and stakeholders around the world;
- Develop consensus goals and framework – A stakeholder-consented framework to enhance trust in AI and certification/accreditation program goals must be developed to promote and verify effective implementation of risk-mitigation practices;
- Operationalize program design ā Clear definitions of the certifiable/accreditable entity must be identified. A range of standards should be defined in accordance with an overarching framework and program goals. A measurement system that allows for an independent verification of whether entities have met the standards must be developed. The certification/accreditation program must also strike the right balance between ensuring meaningful adherence to standards without stifling ongoing innovation and improvements over time. Standards and assessment methods shall be continuously reviewed;Ā
- Create market demand – Verified adherence to best practices through certification/accreditation can improve AI developersā and implementersā brand through the ability to publicize adherence to standards. For example, being branded as a trusted developer and user of AI products or services may increase demand from customers; and
- Evaluation of program effectiveness – Certification/accreditation programs should be evaluated to ensure they meet their objective of increasing trust and adherence to best practices.
AI Legislation
The paper points to the fact that till date, the rise of AI has largely occurred in a regulatory and legislative vacuum. Apart from a few US statesā legislation regarding autonomous vehicles and drones, few laws or regulations exist that specifically address the unique challenges raised by AI. This is where industry self-governance can step in. It can establish standards for globally distributed products and services across jurisdictions, reducing the potential of inconsistent regulations, as well as the need and resources potentially required to achieve international harmonization of government regulations at a later point. Further, in spite of the fact that there exist regulations and/or industry self-governance mechanisms in place, it does not obviate the need for addressing liability arising out of the use of AI systems. Unlike an accrediting organization or regulatory agency, which play a role of enforcing the prevention of any harm from the use of AI products/services, courts are reactive institutions as they apply tort law and adjudicate liability in individual cases of alleged harm, after the same has occurred. At present, there is no precedent set by the courts regarding who should be held liable if an AI system causes harm. Consequently, the principles contained in the established law would be taken into account by the courts to adjudicate liability. Therefore, in order to establish legal links between certification and liability, the paper proposes the framing of an Artificial Intelligence Development Act (AIDA) that could stipulate a certification scheme under which designers, manufacturers, sellers, and implementers of certified AI programs would be subject to limited tort liability, while uncertified programs that are offered for commercial sale or use would be subject to stricter joint and severally liability.
Considerations for effective self-governance
The paper calls for consideration and mitigation of a number of risks and potentially unintended consequences when relying on industry self-governance as a complement to other legislative or regulatory efforts to foster responsible use of AI. Self-governance will fall short when the costs of self-governance to industry are higher than the alternatives. Importantly, self-governance is likely only successful if all stakeholders have confidence that standards and verification methods were developed by appropriately balancing perspectives of patients, clinicians, AI developers, AI users etc. The paper concludes by referring to the fact that the advancement of AI is actively being promoted by the US government, other governments and supranational entities like the European Union. Further, governmental management of public risks such as AI risks typically occurs in democratic societies through the actions of the legislative, executive and judicial branches of government. However, AI-specific legislation, regulation or established legal standards or case law largely do not exist worldwideāor they apply only to a narrow subset of AI health solutions. In such a scenario, evidence-based risk mitigation practices, promulgated through self-governance and certification and accreditation programs, could prove effective in promoting and sustaining user trust in AI.
Between the lines
A system based on industry self-governance has the potential to be a feasible solution for an effective and expedient response to the challenges of AI regulation. It creates a belief amongst the industry players that the growth and the survival of the industry are dependent on self-regulation. However, to be successful in a broader sense, the self-regulatory mechanism shall have an effective enforcement mechanism, built into it. To add to the above, the self-governing system has to be transparent with respect to its internal working. With the expansion in the use of AI systems, and the regulatory struggle to keep it within bounds, the mechanism of industry self-governance can prove handy for the AI stakeholders in particular, and the society, in general.