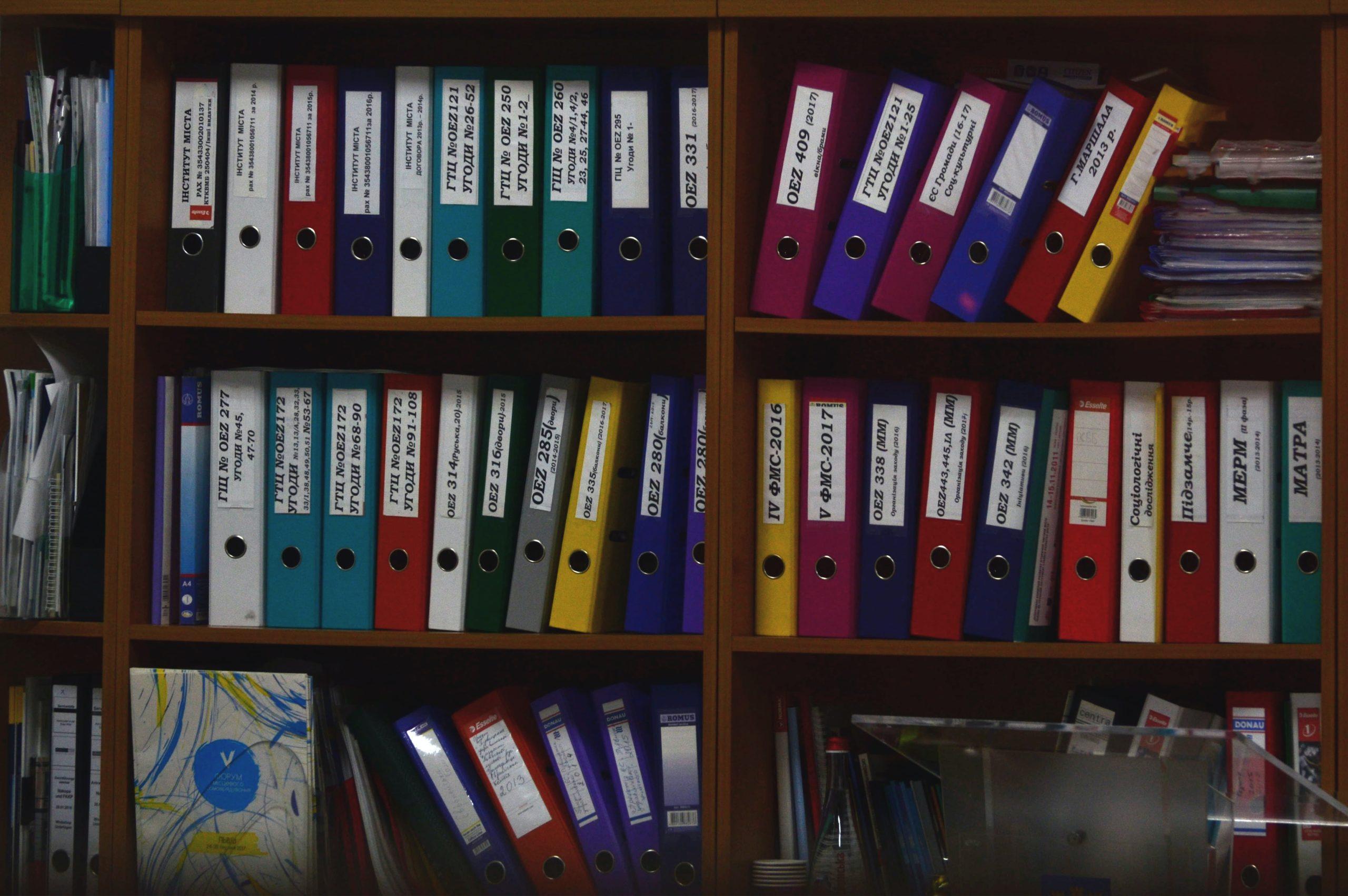
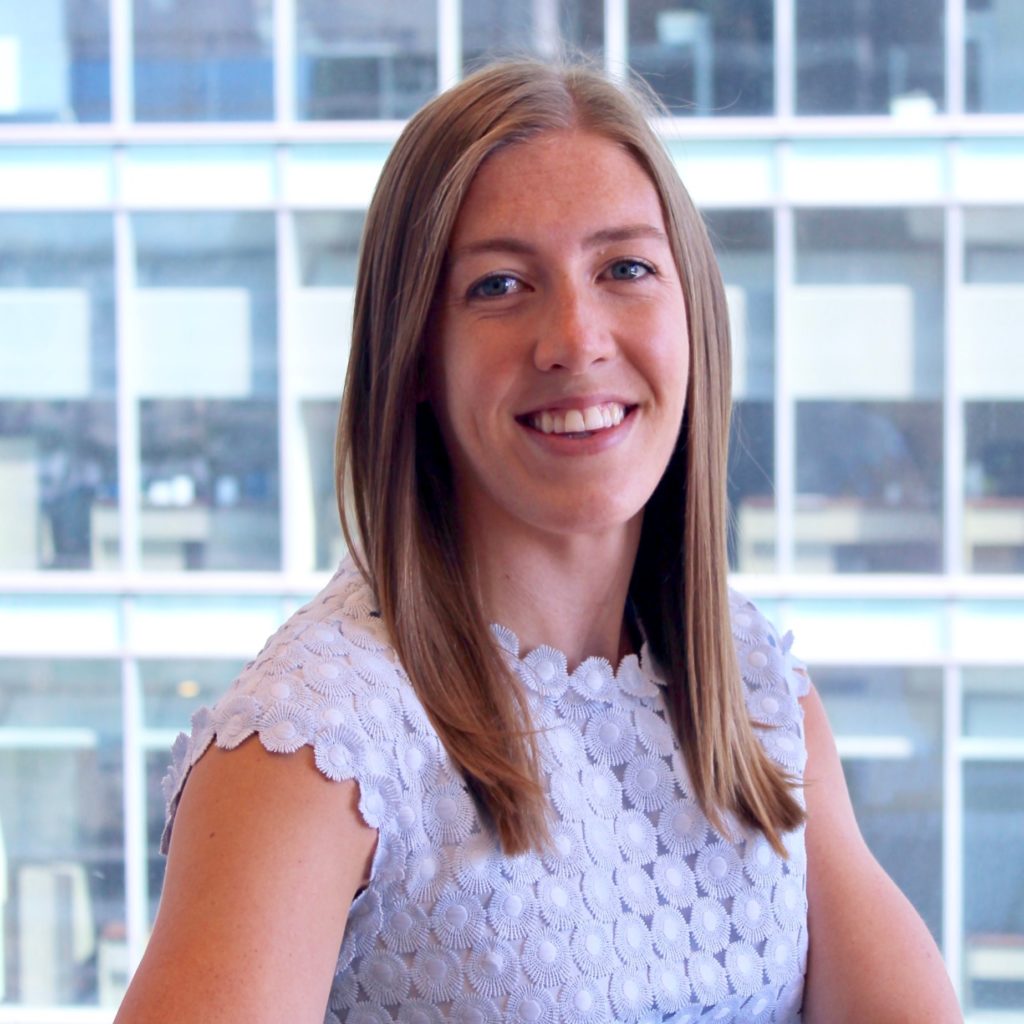
🔬 Research summary by Stephanie Kelley, a PhD Candidate in Management Analytics at the Smith School of Business, Queen’s University where she studies the ethical of artificial intelligence, data, and analytics.
[Original paper by Stephanie Kelley]
Overview: The proliferation of ethical issues related to the use of artificial intelligence (AI) has led many organizations to turn to self-regulatory initiatives, most commonly AI principles. This study investigates what organizations can do to effectively adopt these AI principles, in hopes of reducing the ethical issues related to the use of AI technologies in the future.
Introduction
Reports of organizations proliferating bias and discrimination, jeopardizing customer privacy, and making other ethical failures in their use of AI continue to dominate headlines. Organizations like Apple, Goldman Sachs, IBM, Microsoft, and Amazon have all been accused of unethical uses of AI in the past few years. Many of these organizations have put self-regulatory initiatives, including AI principles, in place as a possible method to reduce the ethical issues – but is having AI principles enough to prevent ethical harm?
This paper explores this by asking “according to the perceptions of employees who work with AI, what components might relate to the effective adoption of AIPs?”
To answer this question, 49 in-depth semi-structured interviews were conducted with individuals employed in financial services organizations who work with AI. The interview transcripts were coded following a general inductive approach, drawing on a positivist epistemology (which assumes the interviewees are direct holders of accurate information).
The findings of the analysis suggest that there are eleven components that are important for the effective adoption of AI principles: communication, management support, training, ethics officer, reporting mechanism, enforcement, measurement, accompanying technical processes, sufficient technical infrastructure, organizational structure, and interdisciplinary approach.
Key Insights
Before proceeding to a summary of the main findings, a definition of AI principles (AIPs) is provided. AIPs are a formal document developed or selected by an organization that states normative declarations about how artificial intelligence ought to be used by its managers and employees. Given this definition, the task was then to determine what components relate to their effective adoption?
Components for effective adoption: Lessons learned from business codes
While AI principles are a new form of ethical documentation, business codes have been around for several decades, and have consequently been studied significantly more than their nascent AI-focused counterparts. These past studies suggest that having a business code by itself does not lead to a reduction in unethical behavior, but that there are several components that impact the adoption of business codes to improve their effectiveness. Building upon the study of business codes, this study used semi-structured questions to ask AI experts whether these same components might also impact the effective adoption of AIPs.
Indeed, employee perceptions on the effective adoption of AIPs suggest that there are eight components common to the effective adoption of business codes. These components are discussed herein:
Communication
Communication, the act of making employees aware of an AIP is an important first step in its effective adoption, as simply having an AIP is not enough to ensure effective adoption. Specifically, participants suggested five aspects of communications that could create effective adoption:
- reach,
- distribution channel,
- sign-off process,
- reinforcement,
- communication quality, and
- external communication.
Management Support
Management support, or employees knowing that one or both of local management or senior management support the company’s AIP, aids in effective adoption. Support includes actions such as modeling appropriate ethical behavior, talking about the code, knowing and understanding the code, or generally taking the code seriously.
Training
Offering AIP training, whereby employees attend a training session or class to educate them on the AIP was found to impact the effective adoption of AIPs. While the idea of mandatory training received pushback from participants, simply the existence of AIP training suggested that the AIPs were important, ultimately impacting effective adoption. Participants also highlighted the importance of internal training, over external.
Ethics Office(r)
Having either an ethics officer, a specific department or group that deals with ethics and conduct issues; or an ethics committee, a group of people in an organization that employees can turn to with their AIP concerns were found to impact the effective adoption of AIPs.
Reporting Mechanism
The existence of a reporting mechanism whereby an employee can report ethical concerns via a telephone line, app, email address, or other means has been found to impact effective adoption. Participants also noted the importance of a standardized procedure: a clear routine for reporting the ethical issue or complaint.
Enforcement
The use of enforcement mechanisms, such as audits, penalties for breaching the code, communicating violations, and incentive policies all aid in the effective adoption of AIPs.
Measurement
The use of some method of evaluating the achievement and/or failures related to the AIP may suggest an organization is serious about the document. While only a couple of organizations today are measuring adherence to AIPs, many organizations noted interest in future measurement, suggesting its potential importance for effective adoption.
Components for effective adoption: Unique learnings for AIPs
In addition to the components that jointly affect the effective adoption of business codes and AIPs, four unique components were identified to independently impact AIP adoption, through open-ended questioning of participants:
Accompanying technical processes
The existence of an accompanying technical process that provides detailed technical guidance on how to implement the AIP aids in its effective adoption. The processes are referred to as checklists, frameworks, assessments, and guidelines.
Sufficient technical infrastructure
Having a sufficient technical infrastructure, specifically, a complete inventory of AI projects, and data and system compatibility are important for the effective adoption of AIPs. Participants suggested that a complete AI inventory helped to “cascade” the AIPs to relevant individuals, and ensure all models are “going through the checklist.”
Organizational structure
Participants suggested that centralized AI teams would aid in the effective adoption of AIPs, as the organizational structure would aid in gathering a complete AI inventory, distribution, and reinforcement of the AIPs.
Interdisciplinary approach
Almost every participant noted the effective adoption of AIPs is a highly complex problem that has not yet been solved but suggested that one way to deal with it is to use an interdisciplinary approach. This includes the creation of interdisciplinary teams, combining AI ethics with data ethics, hiring the right people, engaging with third-party experts, and engaging with regulators.
Between the lines
The findings of the study suggest that there are eleven components that are important for the effective adoption of AI principles: communication, management support, training, ethics officer, reporting mechanism, enforcement, measurement, accompanying technical processes, sufficient technical infrastructure, organizational structure, and interdisciplinary approach.
What this means is that simply having AI principles will not be enough for organizations to prevent unethical AI outcomes, and instead, they should consider additional AI ethics components, per the eleven uncovered in this study, to aid in effective AI principle adoption. The findings also suggest that organizations and researchers should treat AIPs as separate entities from existing business ethics codes.
There are, of course, limitations to the study: participants were all employed in one industry, public organizations and NGOs were not studied, snowballing sampling could have created selection bias, and the qualitative nature of the study relies on self-reported data which could be affected by social desirability bias. Future studies should explore additional industries, public organizations, NGOs, and measure direct behavioral evidence. Additional research to clarify the quantitative importance of each of the components is warranted to help organizations prioritize their AIP efforts and ultimately help reduce unethical AI outcomes.