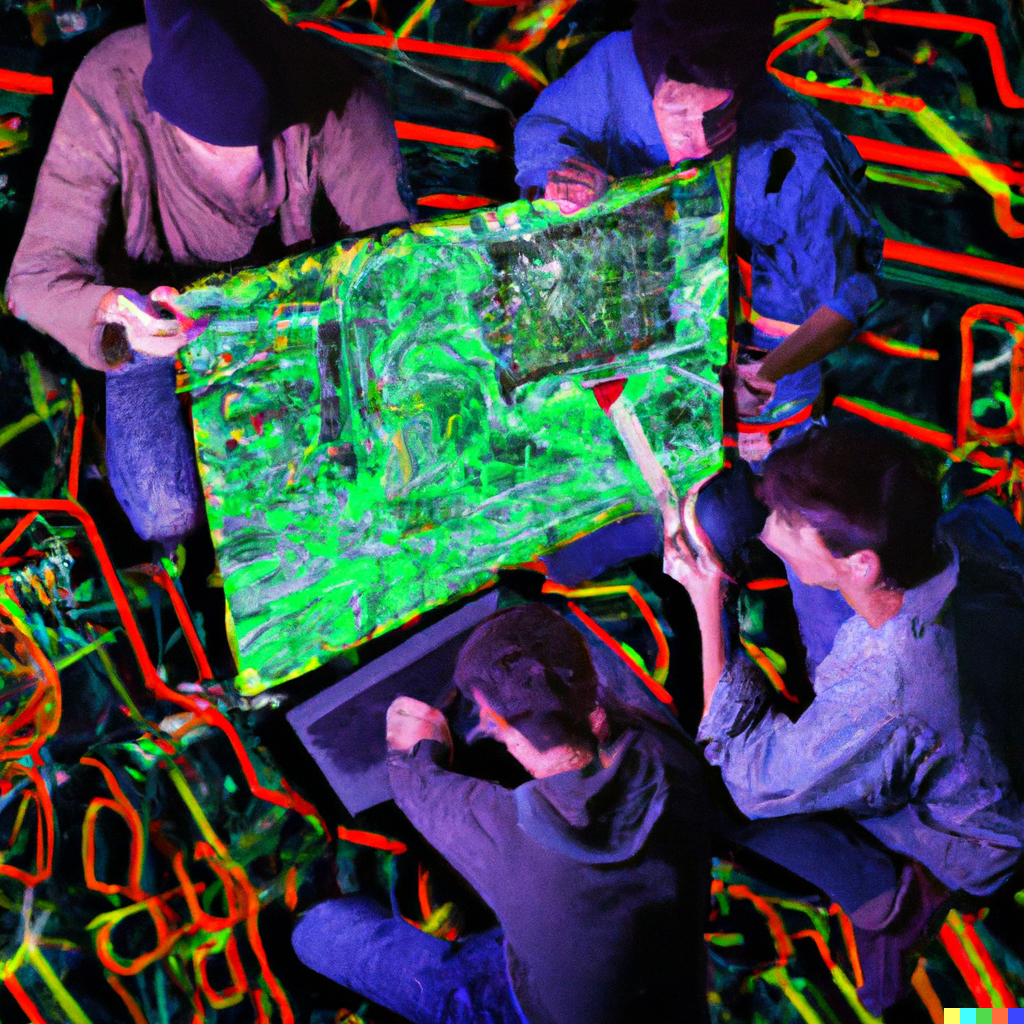
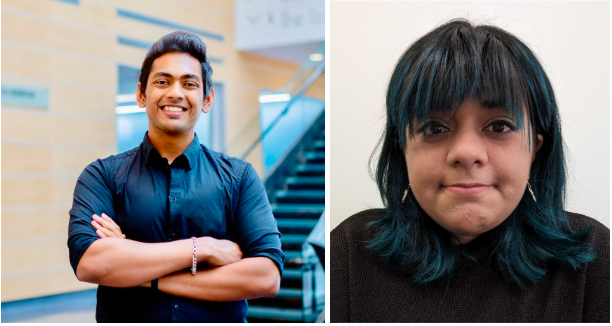
🔬 Research Summary by Avijit Ghosh and Dhanya Lakshmi.
Dr. Avijit Ghosh is a Research Data Scientist at AdeptID and a Lecturer in the Khoury College of Computer Sciences at Northeastern University. He works at the intersection of machine learning, ethics, and policy, aiming to implement fair ML algorithms into the real world.
Dhanya Lakshmi is an ML engineer who has worked in many industries, including ML Ethics and cybersecurity. Her work lies in researching and understanding the adverse effects of algorithmic harms on the internet across disparately affected groups.
[Original paper by Avijit Ghosh and Dhanya Lakshmi]
Overview: The increasing prominence of Generative Artificial Intelligence is evidenced by its use in consumer-facing, multi-use text and image-generating models. However, this is accompanied by a wide range of ethical and safety concerns, including privacy violations, misinformation, intellectual property theft, and the potential to impact livelihoods. To mitigate these risks, there is a need not just for policies and regulations at a centralized level but also for crowdsourced safety tools. In this work, we propose Dual Governance, a framework that combines centralized regulations in the U.S. and safety mechanisms developed by the community to tackle the harms of generative AI. We posit that implementing this framework promotes innovation and creativity while ensuring generative AI’s safe and ethical deployment.
Introduction
Motivation
With the rise of text-to-image Generative AI models, independent artists have discovered an influx of artwork whose style is very similar to theirs. Their work has been crawled without permission, and now there are applications producing images in their distinctive art style given a prompt. Options for recourse are very limited. Sure, the artists may be able to sue the company, but a successful lawsuit takes years. They may be able to use some open-source tools they find to stop future scraping of their work, but this also requires finding reputable and well-maintained tools that fit their needs.
Furthermore, this situation affects independent artists, large companies, and, of late, actors and writers. Artists have launched a lawsuit against Stability AI and Midjourney, Getty Images is suing Stability AI for unlawful scraping, and recently, the use of ChatGPT and Generative AI to generate scripts for television and recreate actors’ likenesses has become a key point of contention between AMPTP and the Writer’s Guild and SAG-AFTRA in the ongoing strikes.
There are two main problems from these events: Firstly, the absence of centralized government regulations and the lack of access to a channel to report problems encountered, and secondly, the absence of a trustworthy repository of tools that are available for stakeholders to use in such situations.
Proposed Policy Framework
To tackle these problems and create guardrails around the use of generative AI, we propose a framework that outlines a partnership between centralized regulatory bodies and the community of safety tool developers. We analyzed the current AI landscape and highlighted key generative AI harms. Next, by considering the stakeholders for AI systems, including rational commercial developers of AI systems and consumers of AI products, we defined criteria that would need to be fulfilled by an effective governance framework. We then reviewed policies to tackle AI harms by U.S. agencies, such as the Federal Trade Commission (FTC) and the National Institute of Standards and Technology (NIST). Similarly, we examined crowdsourced tools developed by ML practitioners in the industry and evaluated how well they fit the defined criteria individually.
Finally, we introduced our framework and described how crowdsourced mechanisms could exist alongside federal policies. Crucially, our framework includes establishing standards for developing and using AI systems through a federal agency (or a collaboration of agencies), methods to certify crowdsourced mechanisms to create trust with users and a method to create and add new policies to the framework. We also demonstrate how our framework satisfies the previously stated criteria.
Key Insights
Criteria for an Integrated Framework
This paper identifies six criteria that a governance model for AI systems must fulfill to be effective:
- Clarity: The framework’s policies must be easy to understand and technically feasible to implement as solutions. This will also make the framework widely accessible.
- Uniformity: The compliance standards should be clearly defined so stakeholders interpret them uniformly. Having templates where possible will make this easier.
- Availability: Complying with the policies in the framework should not be expensive, time and money-wise, and should allow innovation. The tools available in the framework should apply to multiple situations.
- Nimbleness: The proposed framework should be able to keep up with new developments in generative AI and equip consumers with new safety tools as new generative AI models emerge. This is important to assure consumer safety while centralized regulation is being debated by governments.
- Actionable Recourse: Consumers should know where to go to contest decisions made by a system that uses AI for decision-making and request an alternative, non-automated decision-making method. There should also be a way to report any violation of laws that they encounter.
- Transparency: The safety tools and mechanisms in the framework should be public, where reasonable, to increase trust in the governance model.
Policies Implemented by U.S. Governance Agencies
The regulatory developments in the U.S. by five agencies are assessed in this paper. The consumer-focused nature of these agencies informs their laws, and a variety of focus areas, including finance, employment, and law, are covered by at least one of these five agencies. AI regulations created in different domains protect consumer rights across multiple avenues. There is sometimes collaboration between agencies, meaning that policies and laws created by one agency can inform or be adapted by another. Finally, a new joint initiative from the EEOC, FTC, CFPB, and CRT can allow for more effective collaboration and potentially standardizing terminology in AI systems. The scope and policies implemented by the five agencies discussed are summarized below.
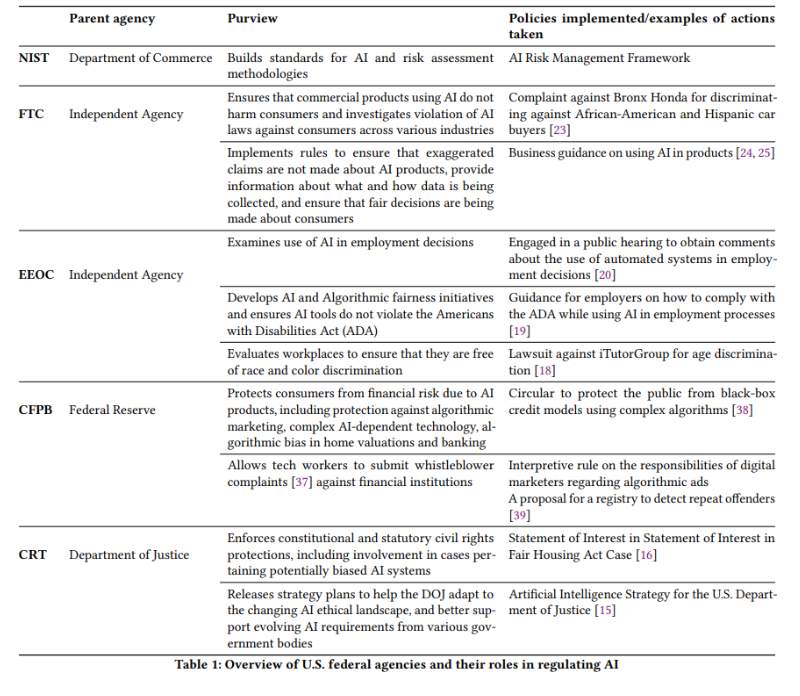
Merits of Crowdsourced Safety Mechanisms
The research paper also elaborates on different types of crowdsourced safety tools. While centralized regulation is necessary to provide assured protections against AI harm to consumers, keeping up with new and evolving AI models can be hard. In the interim, crowdsourced tools are especially useful in allowing users to protect themselves. The most commonly used techniques in the tools are described below:
- Training modifications: Tools like Glaze can help modify users’ artwork to interfere with AI models’ ability to read data on their artistic style. There is also a new line of research that is exploring ways to edit models to remove specific information from trained images or delete memories from transformer-based language models. Similarly, there are tools for Deepfake prevention that modify images of the faces of potential victims so that the generated artifacts using these images are undesirable and easy to detect.
- Data Provenance and Watermarking: Another set of tools deals with the images used or produced by these AI models. Watermarking helps protect models’ outputs, while data provenance tools identify whether consumers’ images are present in the dataset used to train a generative AI model. These tools are really useful in associating ownership with the generated images and searching through large datasets.
- Licensing and Hackathons: Community standards such as the Responsible AI Licenses (RAIL) provide developers with a way to limit the application of their AI technologies. On the other hand, hackathons, bug bounties, and red-teaming activities allow a diverse community to identify wide-ranging harms in AI applications.
Advantages of Dual Governance: A Unified Framework
Our proposed framework, Dual Governance, aims to integrate crowdsourced safety tools with a centralized regulatory body such that there is synergy between the laws being implemented to protect users and tools available to users to protect themselves. At a high level, the framework involves an existing federal regulatory body (or a collaboration between agencies) that would establish guidelines and standards for developing and using AI systems. The framework can incorporate crowdsourced safety mechanisms, involving stakeholders in assessing and improving AI systems.
To bring clarity to existing regulations, the Dual Governance framework pairs them with clear technical solutions. A registry of ways to comply with regulations and an established way to interpret them makes the framework uniform and transparent. New frameworks and existing tools should be periodically reviewed so that the framework remains nimble and relevant. Finally, the framework will have methods to alert agencies to malpractice. It can borrow from centralized regulatory agencies such as CFPB and the human alternatives proposed in the Blueprint for an AI Bill of Rights so that consumers have paths for recourse.
Between the lines
The increasing usage of generative AI in the mainstream highlights the increasing need for regulation. Our research analyzes existing AI harms, centralized regulation, and the steps taken by various government agencies to regulate AI and crowdsourced safety tools. We define the criteria required for governance models to ensure the safety of the users in this fast-evolving environment. Then, we introduce the Dual Governance framework, which proposes an alliance between centralized regulation and crowdsourced mechanisms. We believe that implementing this framework will more efficiently regulate generative AI harms and provide consumers and small and big tech companies free rein to innovate on generative AI while ensuring the safety of the user community. We hope this work will be an important step in how we think about governing AI systems and provide a blueprint for further research in building, testing, and securing unified governance models.