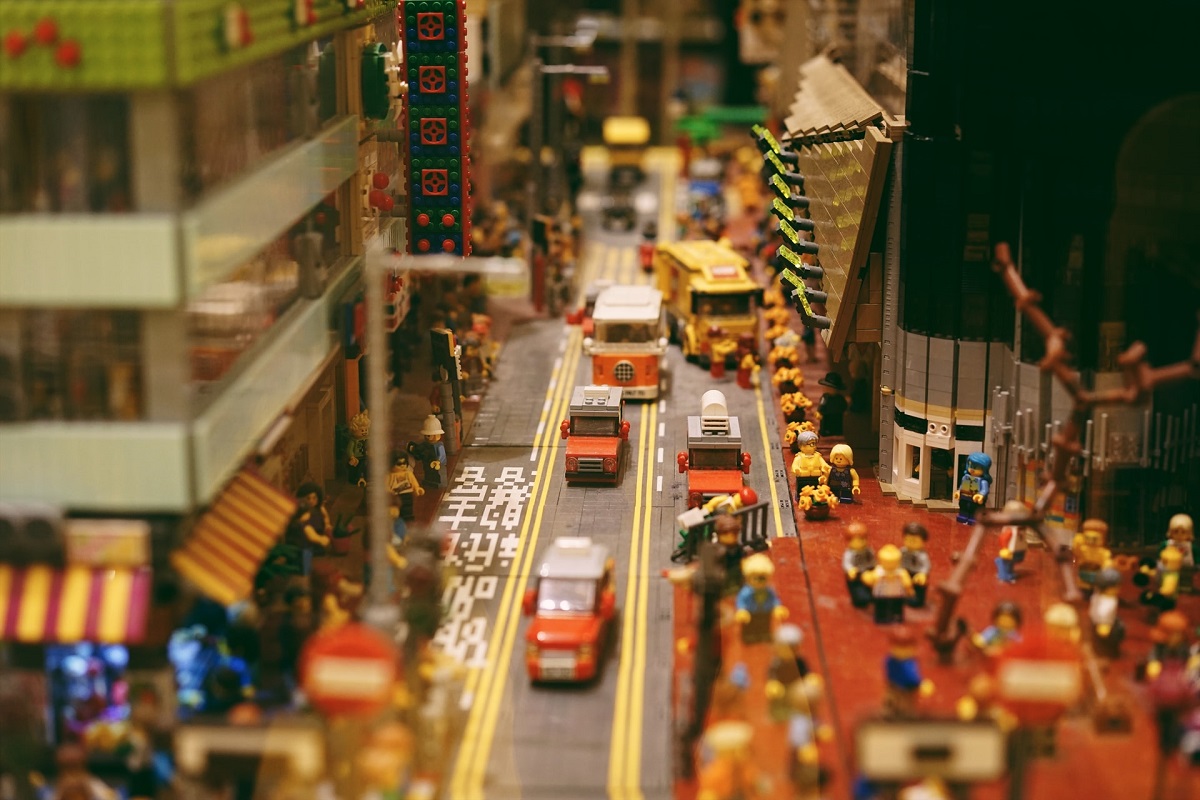
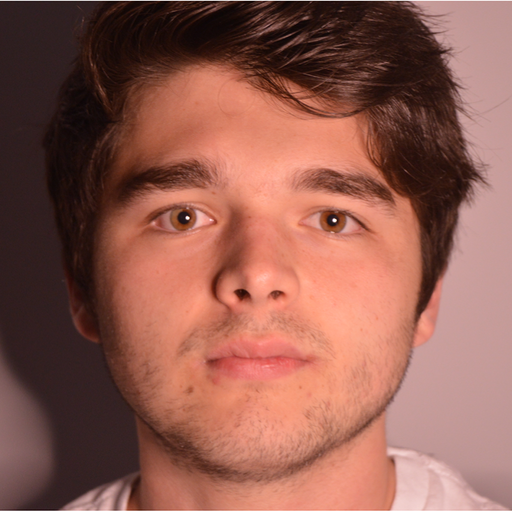
🔬 Research summary by Connor Wright, our Partnerships Manager.
[Original paper by Chao Fan, Cheng Zhang, Alex Yahja, Ali Mostafavi]
Overview: With the potential for a climate crisis looming ever larger every day, the search for solutions is essential. One such solution could then be found in the construction of a disaster city digital twin, allowing governments to simulate the potential effects of different disasters on physical cities through running simulations on their digital replicas. Doing so can help make more tangible the effects of a climate crisis, whether it be through flooding or unusual weather. AI, as a result, has a key part to play in making this a reality.
Introduction
Ever wondered what your digital twin city would look like? Well, the clue is in the title. A digital twin is a digital representation of a city, which the authors then utilise to explain how to integrate disaster city-modelling and AI in order to better predict potential disasters — for example, the effects of a climate crisis on the city. In order to do this, the improvement of information and communication technology is essential, with AI playing a key role. In order to best capture the paper, I’ll elaborate on 5 sections: the first is the importance of simulation. After that, I launch into the 4 aspects of the simulation process elaborated by the authors. I then offer some concluding words on how such modelling can play a key role in not only forecasting how to keep cities safe from a climate crisis, but also how to best keep our future cities safe and prepared.
The importance of simulation
How could such simulation actually help utilise AI to fight a climate disaster? Well, here are my 3 reasons I’ve taken from the text:
- AI will help improve the effectiveness and efficiency of disaster management, mainly seen through its ability to scan large amounts of data and report back in real-time.
- Disasters are super dynamic and so are constantly evolving and changing. This real-time data collection thus, becomes even more important.
- Thanks to this ability, AI will help decision-making, resource allocation and coordination through running simulations as part of the disaster city digital twin (DCDT), running off the data it has collected.
One aspect that the authors mention, which I believe lines up really well with what we do at MAIEI, is how “there is a dire need for interdisciplinary convergence towards a unified vision” (p.g. 2). Here, the DCDT model serves as a common output for multidisciplinary research to converge and aim towards. In order to confront a potential disaster effectively, the use of resources and services from all different parts of society will be required, hence, making collaboration between these different disciplines, paramount.
The authors identify 4 different aspects in this DCDT simulation process:
Sensing the data
Multiple source data sensing will consist of collecting data from multiple different sources to have a clearer picture of what’s going on in the digital city when a disaster takes place. Having a variety of sources then presents a higher probability of information being actualised in real-time, whether it comes from local fire departments or residents’ social media accounts.
To do this, the remote sensing used takes advantage of UAV and satellite technology to paint a picture of how the city was before, during and after a disaster. Thanks to AI technology’s scanning ability, objects having changed locations can then be flagged and sorted into relevant regions where such change is taking place. For example, the AI could identify how a building now no longer exists in the new set of images coming in, using its geolocation to then label its relevant region. This can potentially be represented through a human instructing the AI to compare the two images and then produce a similarity score, with a low score being down to the building collapse.
Collecting the data
In order for this to be possible, the data needs to be collected. Such data comes in 3 different departments:
- The aforementioned remote sensing with the UAV and satellites providing images on the current state of the disaster city in the simulation.
- Social sensing then focuses on social media accounts of local residents, gathering data on societal impacts due to infrastructure disruption and any personal emergencies.
- Crowdsourcing then makes sure that data is coming into the AI from different locations. This allows the AI to analyse the most up-to-date data and produce the most accurate analysis, which the CIEM in Norway is taking full advantage of.
It almost goes without saying that this collection raises some serious privacy concerns. However, such discussion deserves a whole other research summary, so I’ll move on to the analysis of the data for now.
Analysing the data
Due to the focus on crowdsourcing, data will be coming in thick and fast to the AI, as well as in all types of different formats, such as social media posts, images or even audio. So, part of the analysis process is to homogenise the data in order for the AI to better cope. Such analysis could consist of the following:
- A dynamic network analysis examining the type of coordination being done between different actors.
- A meta-network analysis extracting what each actor is doing within the simulation. For example, the previously mentioned dividing up of images into regions through using natural language processing to scan street names.
- The above-mentioned image-tracking can then better identify the different infrastructures being lost or moved.
- The already touched upon the use of social analysis social media being used to understand human behaviour during a disaster by providing real-time reactions.
Decision-making based on the data
Having now collected the data, how are decisions to be made as a result? Here, a Serious Game Learning Environment is developed based on the digital city, proving to be the “ use of scientific game-engine simulations of real-world phenomena to solve a problem.”. Here, what can be seen is how different areas are affected infrastructurally (such as flooding due to global warming), which can then allow researchers and developers to cater to the different needs of those regions. Resultantly, the development of common areas of interest in disaster prediction can be established, better allowing for multi-actor cooperation. For example, the simulation of the disaster city allows actors to see how their decisions can affect not only the situation and the people in danger but also other actors themselves.
Between the lines
I’m sure that we’ve all found predicting the future pretty hard at times given the current pandemic, even thinking ahead to the next day! When trying to forecast for years in advance, this difficulty then multiples further. However, what DCDT aims to do is give us a better chance to do so. Already being heavily conducted in China, DCDT could hold a key role in being able to accurately predict how we are to go about keeping our future cities safe from a potential climate crisis and prepared should one occur. Whether it be assessing how to guard against tsunamis or preserve wildlife, AI’s role in this process is not to be underestimated, both in humanistic and environmental terms.