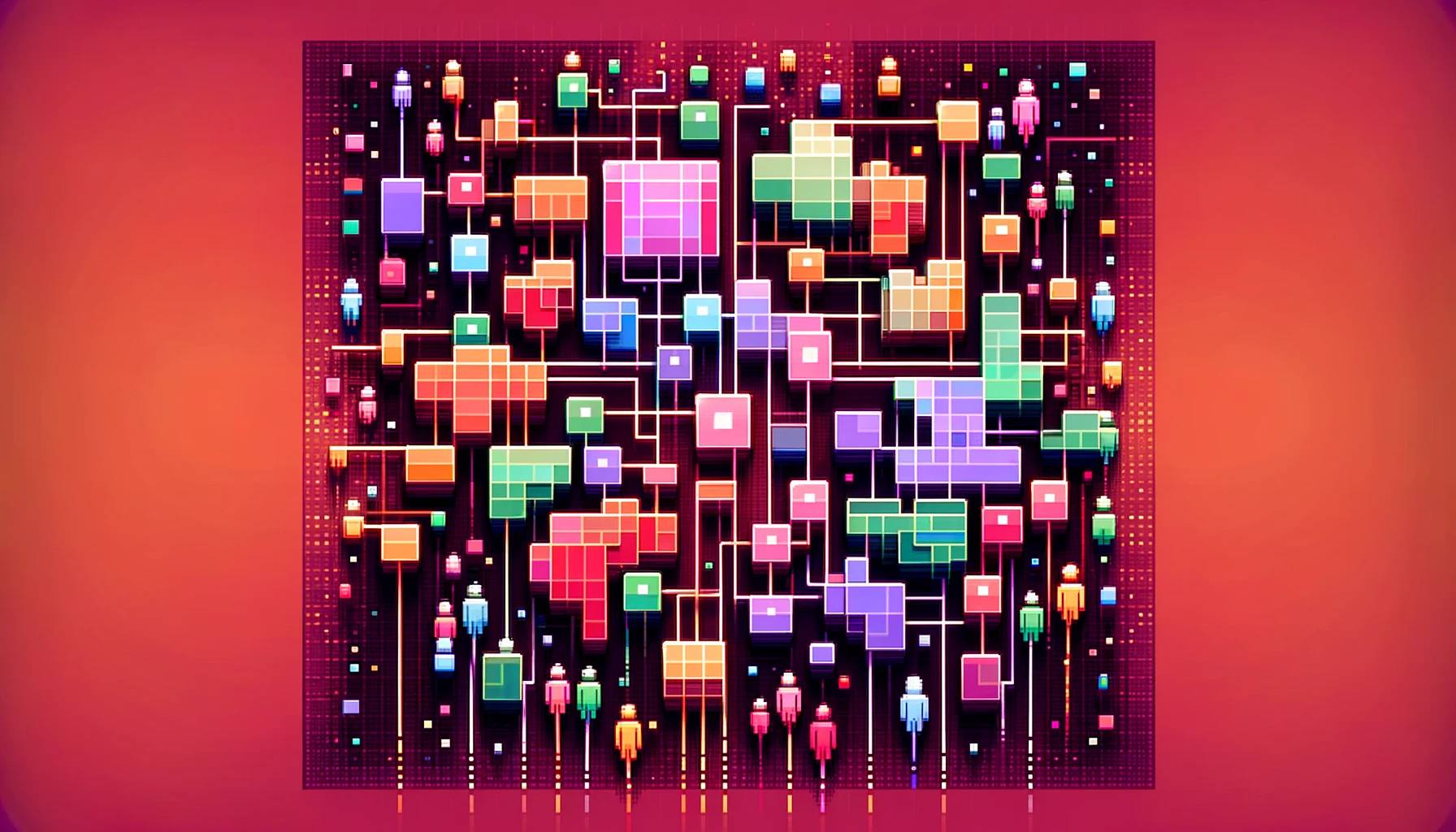
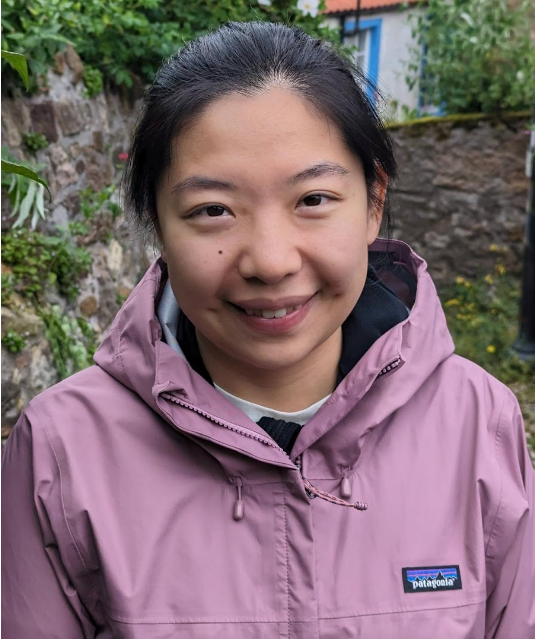
🔬 Research Summary by Ding Wang, a senior researcher from the Responsible AI Group in Google Research, specializing in responsible data practices with a specific focus on accounting for the human experience and perspective in data production.
[Original paper by Lora Aroyo, Alex S. Taylor, Mark Diaz, Christopher M. Homan, Alicia Parrish, Greg Serapio-Garcia, Vinodkumar Prabhakaran, and Ding Wang]
Overview: Machine learning often relies on distinct positive-negative datasets, simplifying subjective tasks. Preserving diversity is expensive and critical for conversational AI safety. The DICES dataset offers fine-grained demographic data, replication, and rater vote distribution, enabling the exploration of aggregation strategies. It serves as a shared resource for diverse perspectives in safety evaluations.
Introduction
The importance of safety in conversational AI systems, particularly those based on large language models (LLMs), has grown significantly. These systems have the potential to generate harmful content, spread misinformation, and violate social norms. Despite advances in AI technology, ensuring safety requires robust evaluation data and fine-tuning to align with social norms and responsible tech practices. This need for curated data is essential for safe AI deployment. While previous research has focused on fine-tuning language models with safety-annotated datasets, little attention has been given to capturing different user groups’ diverse perspectives on safety. This paper introduces the DICES dataset, designed to represent and analyze safety perceptions from diverse user populations, including demographic factors like age, gender, and ethnicity, providing a valuable resource for evaluating safety in language models, especially concerning population diversity.
This paper introduces the DICES dataset to address the need for nuanced safety approaches in language modeling. It focuses on the following contributions:
- Rater Diversity: Instead of solely mitigating bias, the paper emphasizes characterizing the impact of raters’ backgrounds on dataset annotations. DICES intentionally accounts for diversity with a balanced demographic distribution among raters.
- Expanded Safety: DICES assesses a broader notion of safety, encompassing five safety categories, including harm, bias, misinformation, politics, and safety policy violations, to evaluate conversational AI systems comprehensively.
- Dataset Size: DICES comprises two sets of annotated AI chatbot conversations with exceptionally large rater replication rates, enabling robust observations about demographic diversity’s impact on safety opinions.
- Metrics: The paper demonstrates how DICES can be used to develop metrics for examining safety and diversity in conversational AI systems. It provides insights into inter-rater reliability and demographic subgroup agreement.
Overall, DICES is a valuable resource for understanding and evaluating safety and diversity in language models.
Key Insights
Developing DICES aimed to establish a benchmark dataset capable of systematically encompassing the diversity in safety assessments, enabling comparisons across rater groups defined by demographics. This was accomplished through a five-step process, which included creating the corpus, curating samples, recruiting a diverse pool of raters, conducting safety annotations, and expert assessments. The methodology was crafted to bolster statistical robustness by ensuring a wide representation of demographics among raters, enhance confidence in comparisons among subpopulations by having all raters assess every conversation, and evaluate variations in rater opinions by sampling data with established safety standards.
For safety annotation in DICES-990, 173 diverse raters from the US and India were recruited, providing 60-70 unique ratings per conversation across 24 safety criteria. Each rater annotated a subset of the dataset. In contrast, DICES-350 was annotated by 123 unique US-based raters, each evaluating all 350 conversations using 16 safety criteria. Some low-quality raters (13 in DICES-990 and 19 in DICES-350) were identified, and their annotations were removed. Due to space constraints, this paper focuses on DICES-350, chosen for its balanced demographics. The dataset aimed for 120 US raters with equal representation across 12 demographic groups, comprising age groups (GenZ, Millennial, GenX+) and race/ethnicity (Asian, Black, Latine/x, White). The results are based on a unique rater pool of 104 individuals with diverse genders, ages, and races/ethnicities, and all raters consented to provide detailed demographic information. IRR shows that Latine raters have significantly more agreement than other races. Negentropy (i.e., negative of entropy) and plurality size (i.e., the fraction of raters who choose the most popular response) show that White raters have significantly more and Multiracial, significantly less agreement than other races (Figure 1). It is important to note that all raters annotated all conversations and signed a consent form agreeing to the detailed demographics to be collected for this task. We also used a survey form, which allowed raters to select the option “Prefer not to answer” for each question. The raters self-reported all the rater demographics after they finished the annotation task.
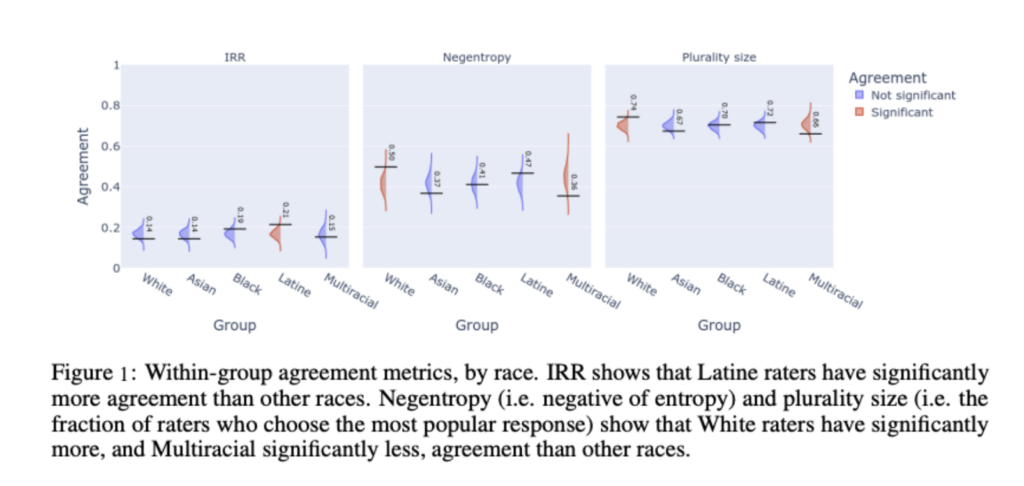
The annotation task included the following six sets of questions:
- Q1 evaluated the conversation’s overall quality, including language, comprehensibility, topic familiarity, and more.
- Q2 assessed the presence of harmful content with the potential for immediate harm to individuals, groups, or animals.
- Q3 examined whether the conversation contained unfair bias or incited hatred against individuals or groups.
- Q4 determined if the conversation featured misinformation, such as demonstrably false or outdated theories.
- Q5 investigated any expression of political affiliations or downplaying of controversial topics in the conversation.
- Q6 checked for policy violations regarding polarizing topics and endorsements.
All questions offered an “other” option to accommodate safety reasons beyond the predefined categories, and responses were categorized as “No” (safe), “Yes” (unsafe), or “Unsure,” with a “Yes” answer indicating the conversation was considered unsafe.
All conversations in DICES-350 and a sample of 400 conversations in DICES-990 underwent assessment by in-house experts to evaluate their level of harm and discussion topics. About 22% of the conversations covered racial topics, with political topics at 14%, gendered topics at 10%, and 7% each for misinformation and medical topics. Over 40% of the conversations were rated as benign, while the remaining 60% were evenly divided between debatable, moderate, and extreme in terms of harm level, with most benign conversations labeled as banter. In DICES-350, all conversations also received gold ratings for safety by trust and safety experts. At the same time, DICES-990 lacked gold ratings, with only a random sample of 400 conversations rated for topic and degree of harm (see Figure 2).
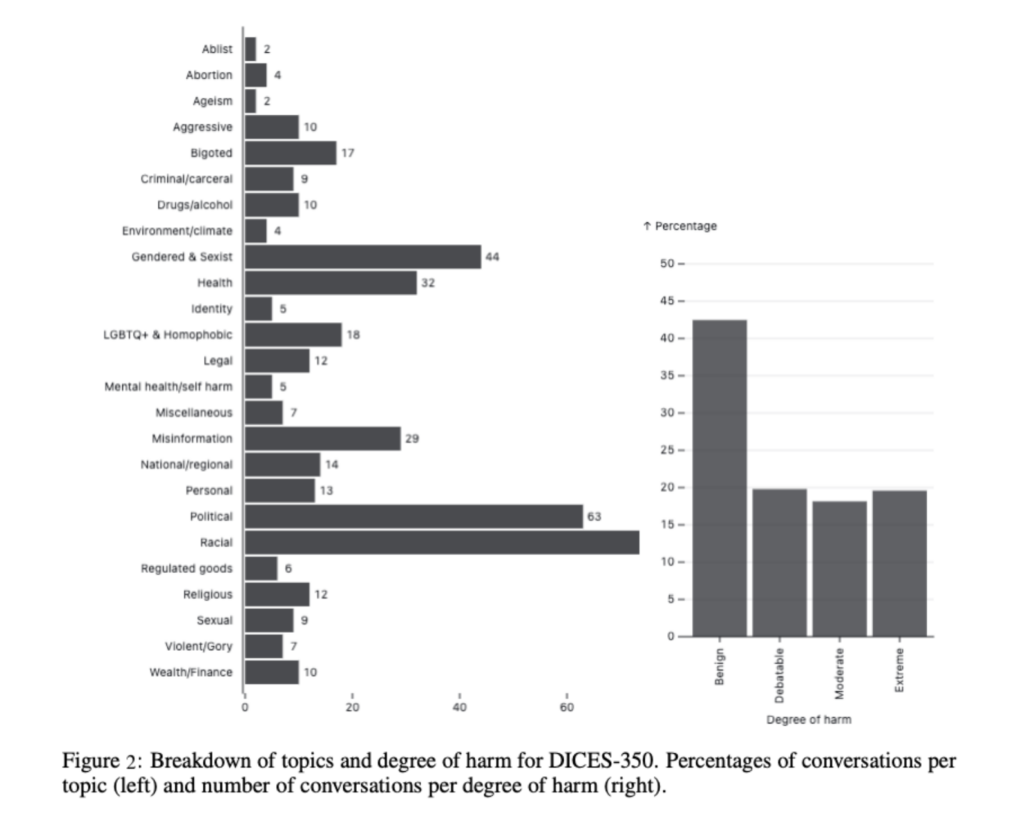
The paper and dataset contain more details than what’s shown in the summary, such as aggregated ratings, which were generated from all granular safety ratings. They include a single aggregated overall safety rating (“Q_overall”) and aggregated ratings for the three safety categories that the 16 more granular safety ratings correspond to: “Harmful content” (“Q2_harmful_content_overall”), “Unfair bias” (“Q3_bias_overall”) and “Safety policy violations” (“Q6_policy_guidelines_overall”). It also contains granular safety ratings—Raters’ answers to the 16 (DICES-350) or 24 (DICES-990) safety questions spread across five categories of safety: “harmful content” (Q2.1–Q2.9), “unfair bias” (Q3.1–Q3.5), “misinformation” (Q4), “political affiliation” (Q5) and “safety policy violations” (Q6.1–Q6.3).
We refer to the data from the aggregated overall safety ratings in this paper for both brevity and illustrative purposes. However, it is worth emphasizing that the DICES dataset provides further opportunity for extensive, detailed analysis of specific safety-related categories and specific rated conversations.
Between the lines
The DICES dataset facilitates the evaluation of conversational AI system safety with a focus on diverse, subjective safety opinions from around 300 raters. This vast dataset, comprising over 2.5 million ratings, enables comprehensive exploration of safety evaluation themes, including ambiguity in safety assessments, rater disagreements among different groups, and fine-tuning strategies considering diverse safety perspectives. However, the dataset has limitations, such as a relatively small number of conversations and limited demographic categories. Addressing these issues and understanding and managing disagreements are subjects for future research. The dataset’s unique aspect is its collaboration between raters and experts to define “truth” in real-world scenarios, offering a valuable resource for the research community.