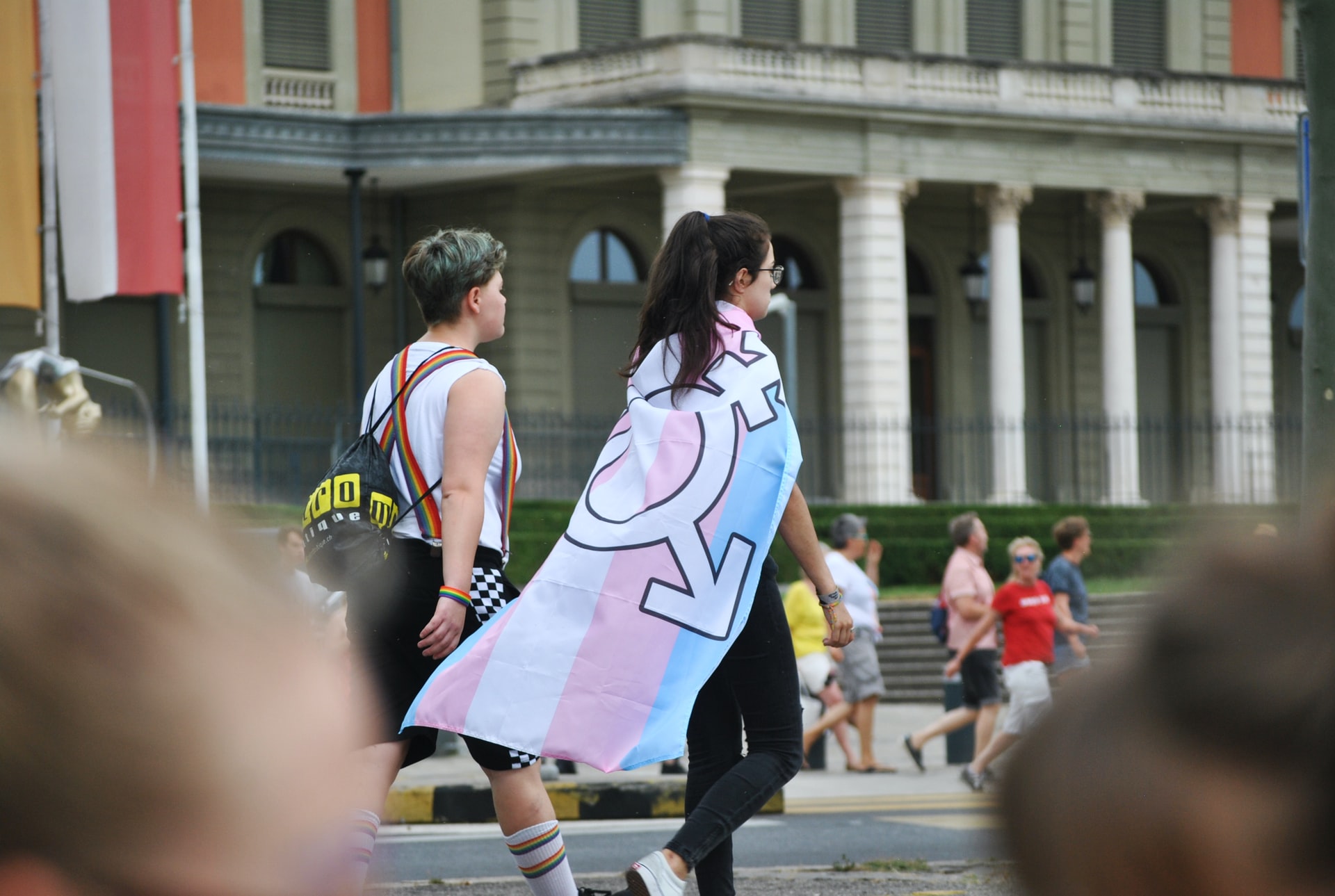
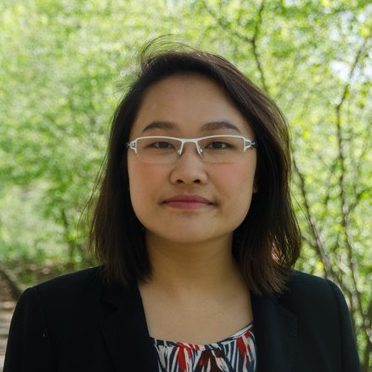
🔬 Research summary contributed by Nga Than (@NgaThanNYC), a doctoral candidate in the Sociology program at City University of New York – The Graduate Center.
✍️ This piece is part of the ongoing Sociology of AI Ethics series; read part 1 (introduction) here.
[Link to original paper + authors at the bottom]
Overview: This paper examines gender biases in commercial vision recognition systems. Specifically, the authors show how these systems classify, label, and annotate images of women and men differently. They conclude that researchers should be careful using labels produced by such systems in their research. The paper also produces a template for social scientists to evaluate those systems before deploying them.
Following the recent insurrection in the United States, law enforcement was quickly able to identify rioters who occupied the Capitol and arrested them shortly after. Their swift action was partly assisted by both professional and amateur use of facial recognition systems such as the one created by Clearview AI, a controversial startup that scraped individual pictures from various social media platforms. However, researchers Joan Donovan and Chris Gillard cautioned that even when facial recognition systems produce positive results such as in the case of arresting rioters, the technology should not be used because of myriad flaws and biases embedded in these systems. The article “Diagnosing gender bias in image recognition systems” by Schwemmer et al (2020) provides a systematic analysis of how widely available commercial image recognition systems could reproduce and amplify gender biases.
The author begins by pointing out that bias in visual representations of gender has been studied at a small scale in social sciences like media studies. However, systematic large scale studies using images as social data have been limited. Recently, the availability of image labeling provided by commercial image classification systems shows promise in social science research. However, algorithmic classification systems could be mechanisms for reproduction and amplification of social biases. The study finds that commercial image recognition systems can produce labels that are both correct and biased as they selectively report a subset of many possible true labels. The findings demonstrate the idea of “amplification process,” or a mechanism through which gender stereotypes and differences are reinscribed into novel social arenas and social forms.
The authors examine two dimensions of biases: identification (accuracy of labels), and content of labels. They use two different datasets of pictures of Congress Members of the United States. The first dataset contains high-quality official headshots, and the other set contains images tweeted by the same politicians. The two datasets are treated as treatment and control datasets. The first dataset is uniformed while the second varies substantially in terms of content. They primarily use results using Google Cloud Vision (GCV) for the analysis, then compare the results with labels produced by Microsoft Azure and Amazon Rekognition. To validate results produced by GCV, they hire human annotators through Amazon Mechanical Turks to confirm the accuracy of the labels.
The authors found two distinct types of algorithmic gender bias: (1) identification bias (men are identified correctly at higher rates than women), and (2) content bias (images of men received higher-status occupational labels, while female politicians received lower social status labels).
Bias in identification
The majority of bias literature focuses on this type of bias. The main line of inquiry is whether a particular algorithm predicts accurately a social category. Scholars have called this phenomenon “algorithmic bias,” which “defines algorithmic injustice and discrimination as situations where errors disproportionally affect particular social groups.”
Bias in content
This type of bias takes place when an algorithm produces “only a subset of possible labels even if the output is correct.” In the case of gender bias, the algorithm systematically produces different subsets of labels for different gender groups. The authors called this phenomenon “conditional demographic parity.”
The research team found that GCV is a highly precise system, which produced labels that human coders also agreed with. However, false-negative rates are higher for women than men. In the official portrait dataset, men are identified correctly 85.8% of the time, while 75.5% of the time for women. In the found Twitter dataset, the accuracy is much lower and more biased: 45.3% for men, and only 25.8% for women.
The system labels congresswomen as girls, and overly focuses on their hairstyle, color of their hair while returning high-status occupational labels such as white-collar workers, businessperson, and spokesperson to congressmen. In terms of occupation, it returns labels such as television presenters to congressional female members, a more female-associated professional category than businesswomen. They conclude that from all possible correct labels, “GCV selects appearance labels more often for women and high-status occupation labels more for men.” Images of women received 3 times more labels categorized as physical traits and body. Images of men receive about 1.5 times more labels categorized as occupation. In the found Twitter dataset, congressional women are substantially categorized as girls. The authors found similar biases in Amazon and Microsoft systems and noted that Microsoft’s system does not produce high accuracy labeling.
This research is particularly needed as it shows systematically how image recognition technology should not be used in social science research for gender research projects. Furthermore, the research team provides a template for researchers to evaluate any vision recognition system before deploying it in their research. One question that remains for the wider public is whether vision recognition systems should not be deployed in daily and commercial practices at all. If they were to be used, how could an individual or an organization evaluate whether they would amplify social biases through such technology?
Original paper by Carsten Schwemmer, Carly Knight, Emily D. Bello-Pardo, Stan Oklobdzija, Martijin Schoonvelde, and Jeffrey W. Lockhart: https://journals.sagepub.com/doi/pdf/10.1177/2378023120967171