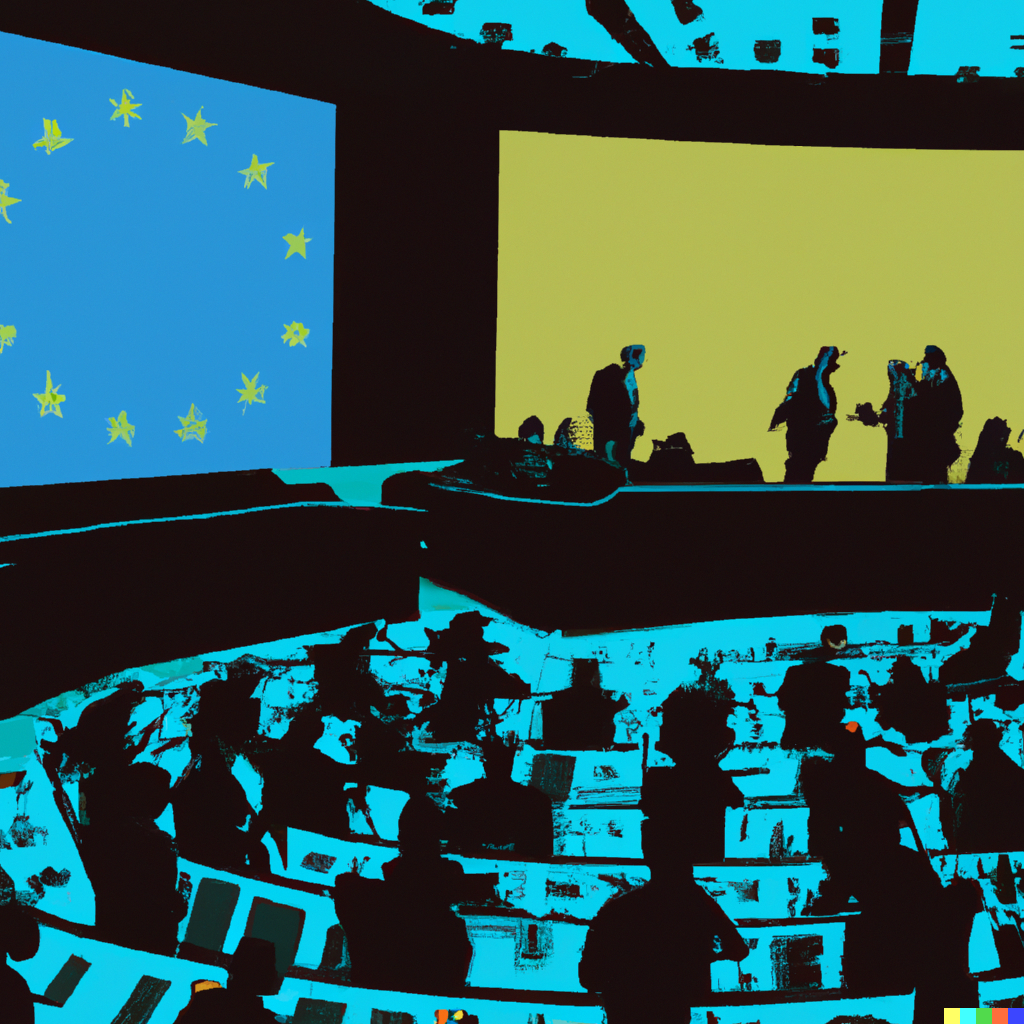
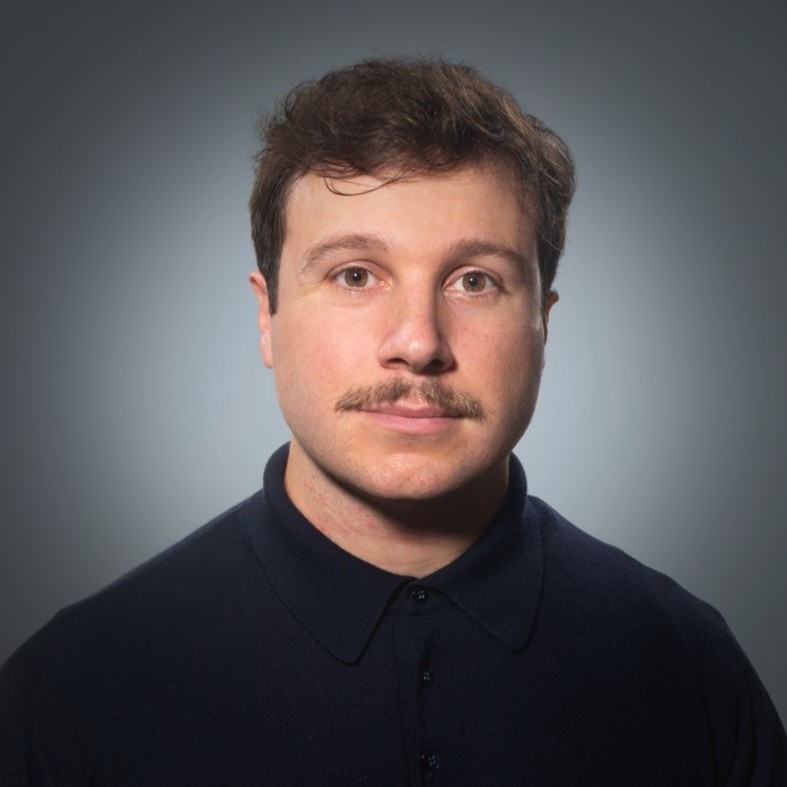
✍️ Column by Carlos Muñoz Ferrandis, RAIL Initiative, HuggingFace.
Overview: Now that the debates around the EU AI Act are getting into their final stages (the Trilogue has already started), Hugging Face and other co-signatories (i.e., Creative Commons, GitHub, Open Future, LAION, and Eleuther AI) have raised their voices through a position statement standing for a clearer framework for open source and open science in the EU AI Act.
Open source has been increasingly relevant for the EU’s digital economy these past years. Research sponsored by the European Commission has outlined the core role of open source (OS) for the EU’s innovation policy and, more precisely, in critical industrial contexts such as standardization or AI. A study from the European Commission rightly states:
“Throughout the years, openness in AI emerged as either an industry standard or a goal called for by researchers or the community.”
Whereas OS is seen as an innovation booster, generative AI and its massive adoption have considerably upset the initial plan for the overall EU AI regulatory approach. It was not easy to anticipate that a new set of artifacts different from code would be the new open wave (i.e., machine learning models).
The regulatory debate has been directly impacted by constant AI product releases, such as Large Language Models (“LLMs”) in the market. The scope of the EU AI Act started with AI systems (2021), then AI systems and General purpose AI systems (2022), to finally now, AI systems, General purpose AI systems, and Foundation Models (2023).
Will the EU AI Act kill open-source AI?
Policymakers are committed to making things right to promote open access and sharing of AI in the EU. EU policymakers got from the first draft of the EU AI Act in 2021, where no mention was made of “open source” AI, to a proposed set of amendments devoted to “free and open source” AI components by the European Parliament.
What’s the scope of the “free and open source” exemption?
The exemption takes a component-based approach with ML models, datasets, and/or code. Probably it went unnoticed, but this has already been quite a step forward, moving from “AI systems” and no mention of open source to “AI components” when thinking about open source under the regulation:
(12a) “To foster the development and deployment of AI, especially by SMEs, start-ups, academic research but also by individuals, this Regulation should not apply to such free and open-source AI components except to the extent that they are placed on the market or put into service by a provider as part of a high-risk AI system or of an AI system that falls under Title II or IV of this Regulation. (…)
(12b) Neither the collaborative development of free and open-source AI components nor making them available on open repositories should constitute a placing on the market or putting into service.”
Are all open ML models exempted?
No. According to the last set of amendments provided in the European Parliament (i.e., not definitive), this will depend on the intended use (i.e., including the open source ML model as part of a high-risk AI system or not) or on the type of ML model (i.e., foundation model). Thus, it can happen that an open ML model is used as part of a system to run an electricity central (i.e., high risk as per Annex II AI Act). Therefore the deployer of this model will have to comply with the requirements for high-risk AI systems and provide information about the model.
Will the developer of the OS model, in case it’s a different stakeholder, be required to share information with the deployer? Not very clear. In principle, the OSS developer will be exempted (recital 12c):
“The developers of free and open-source AI components should not be mandated under this Regulation to comply with requirements targeting the AI value chain and, in particular, not towards the provider that has used that free and open-source AI component.”
Does it mean that OS AI will be exempt from regulation?
No. However, the applicable legal regime should be carefully crafted and articulated with already existing community self-governance approaches.
For instance, model cards are one of the simplest yet most effective open documentation tools, which are becoming the standard in the AI industry for documenting AI. There are still no formal harmonized standards; some will probably come in the next years. Hugging Face has its own specification and has recently decided to develop the concept of a Governance Card along with ServiceNow for open/collaborative AI development projects. Cohere, Meta, and Microsoft also have their own formats of AI cards, which might depend on the AI product and the product’s audience (ML engineer, end customer, or authority). Meta’s and Microsoft’s cards are even steered toward pseudo-regulatory compliance.
High-quality model cards can provide a considerable amount of information which is required by some of the EU AI Act provisions (e.g., Article 13, Annex IV). We are progressively developing open and simple community tools exploring the intersection between documentation tools and regulatory compliance, an example is RegCheck. The latter is an experiment to automate compliance with the EU AI Act based on model cards’ information. Collaborative development and open access to AI governance tools are core steps toward democratizing trustworthy AI.
What about foundation models?
Due to their potential, foundation models shared on an open basis will not benefit from the “free and open source” exemption, as of now and through the lens of the European Parliament. The challenge with the concept of “foundation” models is that these are becoming a commodity, and in a few years, the concept of a “foundation model” will rather become a “commons model.”
Moreover, from the lens of the policymaker, one thing is to consider these models “powerful,” and another different one is to set “power” as the main metric to assess the regulatory scope for this type of model. Instead, a more tangible set of metrics should inform the regulatory constraints on these models. See also the thorough analysis provided by Open Future.
Are the requirements on OS foundation models a critical burden to deploy them?
Some of these requirements are unclear and might be expensive, especially for research labs and SMEs (e.g., to set a quality management system), but not others, such as model or dataset documentation (much needed).
How will restrictions on using high-risk AI systems, GPAIs, and foundation models impact open-source AI licensing?
Nowadays, ML models are still mainly released with open-source licenses such as Apache 2.0 or MIT. However, in their approaches to the AI Act, the Council of the EU and the European Parliament want to require restrictions on the conditions of using GPAIs and foundation models. Consequently, this could potentially impact the open licensing practices of some ML models, such as LLMs.
Closely related to it, the European Parliament’s last set of proposed amendments include the development of voluntary standard contractual clauses to govern information sharing and compliance across the AI value chain (taking inspiration from Article 34 of the EU Data Act). New license agreements will likely emerge, Responsible AI licenses, such as the BigCode OpenRAIL-M, LLaMA 2 Community License Agreement, and AI2 Impact License, are tending toward this direction by requiring, e.g., documentation sharing when users share the LLM.
Open access to foundation models and training datasets might enable transparency and auditability. Open training datasets become platforms for more collaborative and scalable data audits, which otherwise would not be feasible for a single AI authority, as research projects such as BigScience have proven.
Regarding copyrighted material used as training data, Article 28b(4)c requires LLM developers to provide a list of copyrighted materials used in their training datasets. Even though it is still not clear the scope and application of this provision, the Dataset Search recently released under BigCode would fit this provision’s expectations. Dataset Search enables users of StarCoder, an LLM for code generation, to check whether the output generated by the LLM matches existing code in the training dataset, and in case so, track the provenance of it and check the license in the open source repository where the code is hosted. Other community-oriented collaborations such as Hugging Face and Spawning one on dataset opt-in and opt-out are also helpful.
We’re now at the cross-path between top-down and bottom-up approaches to AI governance, and we need to leverage it
The last set of amendments proposed by the European Parliament explicitly includes “free and open source AI components” and “model cards” as governance tools enabling better documentation practices in AI. This results from an intersection happening nowadays between top-down (EU AI Act) and bottom-up (community self-governance) approaches to AI governance. The European Parliament has identified and validated that model cards and data cards are increasingly used nowadays as the leading documentation tool to inform users about the core technical characteristics of the AI system or its components.
The cross-path between top-down and bottom-up approaches to govern AI opens collaboration opportunities between policymakers and the AI community. These collaborations have the potential to bring a cohesive approach to AI governance and should be conceived as governance interfaces between policymakers and the AI community. These interfaces will take many forms, such as governance or standardization sandboxes, tooling catalogs (e.g., OECD Catalogue of Tools for Trustworthy AI), or constant dialogues and education about practical niche topics leading to better-informed and more dynamic AI policymaking.
Upcoming AI regulations should prioritize the leverage of already existing governance tools and the power of the community as a core part of the regulatory process. A more open and collaborative approach to AI governance will generate the necessary economic incentives to optimize regulatory compliance.
Appendix – EU AI Act recommended documentation sources to follow + specific provisions
Documentation source: This webpage gathers different EU institutions’ regulatory proposals under the EU AI Act.
EU AI Act drafts:
- EU AI Act’s first draft provided by the Europan Commission on April 2021
- General Approach to the EU AI Act provided by the Council of the EU in November 2022
- The final set of amendments to the EU AI Act to be voted on in June provided by the European Parliament in May 2023
Specific provisions of interest:
- Micro, small, and medium-sized enterprises are defined in the Annex of Commission Recommendation 2003/361/EC
- Within the proposed amendments by European Parliament:
- Definitions of AI system, General Purpose AI system, and Foundation model in Article 3
- Recitals (12)a-c on the open source exemption and mention model/data cards
- Article 2(5d) on the scope of the AI Act and the open-source exemption
- Articles 28(2)a, 28a, and Recitals 60a-d on voluntary standard contractual clauses to be developed by the European Commission
- Article 28b on foundation models’ requirements
- Article 53a(2)d, f and 53a(3) on regulatory sandboxes and favorable provisions to SMEs
- Within the General Approach to the EU AI Act by the Council of the EU:
- Title I.A on General Purpose AI Systems
- Article 69 on Codes of Conduct
- Article 13 on transparency and provision of information to users (which can be articulated with the information provided in model cards and data cards)
Disclaimers
This is an informative blog article where the author expresses opinions about the EU AI Act. In no way are the interpretations or opinions made here that will finally be agreed upon by the EU institutions. Please do not take this as legal advice.
Notwithstanding the definition of open source under OSI’s stewardship, as well as Creative Commons, RAILs, or other licensing initiatives, for the sake of simplicity, we’ve decided to use “open source AI” to refer to open development and licensing dynamics in AI. We do not aim to claim any definition of “open” and acknowledge and respect all the aforementioned.