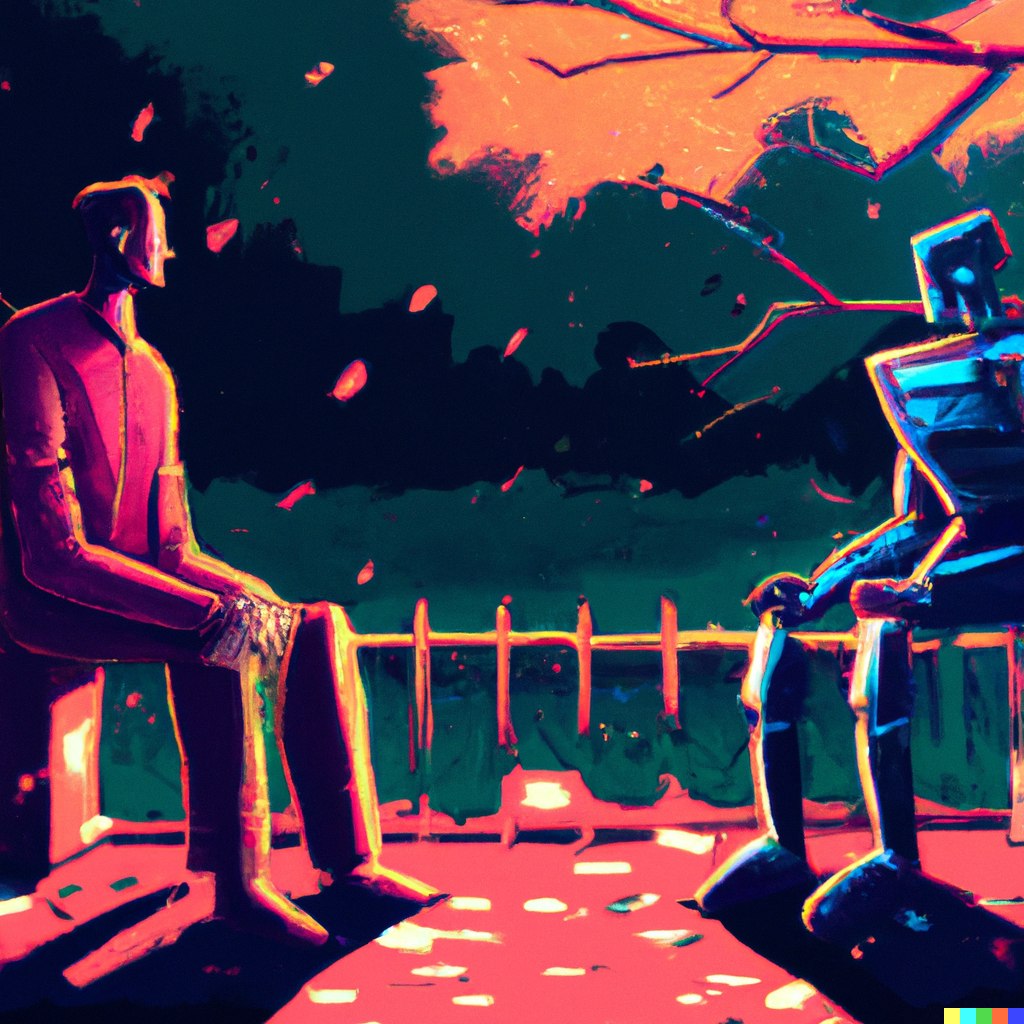
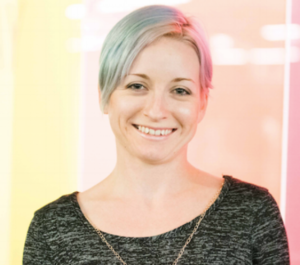
🔬 Research Summary by Jennafer Shae Roberts, a researcher and writer for the non-profit Accel AI Institute. She focuses on the ethics of artificial intelligence coming from an anthropology background.
[Original paper by Jennafer Shae Roberts and Laura Montoya]
Overview: Ethics in Artificial Intelligence (AI) can emerge in many ways. This paper addresses developmental methodologies, including top-down, bottom-up, and hybrid approaches to ethics in AI, from theoretical, technical, and political perspectives. Examples through case studies of the complexity of AI ethics are discussed to provide a global perspective when approaching this challenging and often overlooked area of research.
Introduction
How can we make ethical AI when our world is so terribly unethical?
This research is about digging into the many facets of ethics in AI and how these perspectives are developed to gain an understanding of the current landscape of it. This is important because if nothing is done to create ethical AI, it will replicate questionable and sometimes outright harmful ideals, translating to autonomous outputs and actions learned from our less-than-perfect society.
As researchers, we combed through papers and articles on the subject. We built upon the ideas of other researchers, such as Wallach et al., who first wrote about top-down, bottom-up and hybrid AI morality and included technical and theoretical angles. We added the political perspective to acknowledge where power is situated and how this comes into play when designing ethical AI systems.
Ethics in AI works differently in varying parts of the world, suggesting that a one-size-fits-all approach won’t work for all applications or use cases, and won’t affect everyone equally. However, having conversations about ethics in AI could radiate out and lead us to face societal ethics that are outdated and sometimes outright wrong.
Key Insights
Could a focus on ethics in AI lead to an Ethics Revolution?
Given the inherent complexity of ethics and morality in AI, we utilized the top-down, bottom-up, and hybrid framework to address technical, theoretical, and political perspectives.
Top-down | Bottom-up | Hybrid | |
---|---|---|---|
Technical | Programmed rules Ex: call center chatbot | Machine learning Ex: Reinforcement learning, etc | Has a base of rules or instructions, but then also is fed data to learn from as it goes Ex: autonomous vehicles employ some rule-based ethics, while also learning from other drivers and road experience |
Theoretical | Rule-utilitarianism and deontological ethics, principles, ie fairness Ex: the Golden Rule, the Ten Commandments, consequentialist or utilitarian ethics, Kant’s moral imperative and other duty based theories, Aristotle’s virtues, and Asimov’s laws of robotics. | Experience-based, case-based reasoning Ex: learn as you go from real-world consequences | Personal moral matrix combining rules and learned experience Ex: having some rules but also developing ethics through experiences |
Political | Corporate and political powers Ex: company’s list of principles | People power Ex: groups online of individuals calling for ethics in AI | A combination of ethics from those in power who take into account ethics called for by the people Ex: employees collaborating with their corporation on ethical AI issues |
What do we mean by top-down, bottom-up, and hybrid in the context of ethics in AI?
Top-down signifies that there is some sort of rules coming from a power source at the top. Whether technical programming, theoretical philosophy, or political powers from governments or big tech companies, top-down ethics for AI can look good on paper, such as lists of principles, however, in practice, these can be too broad to be action-guiding and can cause disparities and inequalities which ultimately undermine ethics. (Roberts and Montoya, 2022)
Bottom-up ethics, broadly speaking, is a method of learning from experience. This can be good and bad, as the world is flawed, and sometimes what seems ethical is not actually in line with commonly held values.
Hybrid ethics in AI attempts to take the best of both top-down and bottom-up approaches and create something that is more well-roundedly ethical. However, many examples of what we would label as hybrid ethics in AI still need a lot of work, such as with contact tracing apps for COVID-19 or self-driving vehicles that balance the rules of the road and what they learn from actual drivers.
How do we define the technical, theoretical, and political perspectives of ethics in AI?
Technical top-down and bottom-up ethics in AI primarily concerns how AI learns ethics. Machines are good at narrow tasks, such as memorization or data collection. However, AI systems can fall short in areas such as objective reasoning, which is at the core of ethics. Teaching ethics to AI is extremely difficult, both technically and socially.
Theoretical ethics concerns philosophers and ethicists throughout the ages, who have much to argue about when it comes to right and wrong, which varies greatly across cultures. Furthermore, ethics has been historically made for people, which doesn’t directly translate to AI.
Political ethics highlights where the power and decision-making are coming from, which then has an effect that radiates outward and influences systems, programmers, and users alike. We don’t only refer to politics as coming from the government but also from big corporations and from the bottom-up, coming from crowdsourcing, protests, and other forms of people power. (Roberts and Montoya, 2022)
Case Studies and what we learned
Taking this framework, we applied it to real-world scenarios of applied AI that were ethically debatable. One of the examples was about contact tracing apps for COVID-19 and how differently these worked in places with different levels of government and community compliance. Another example traced an NGO which went to an Indigenous African community to use machine learning to calculate water access, which inadvertently caused harm to the community, and trust was lost.
Contrary to what science fiction would leave us to believe about what to fear with AI, some of the most troubling ethical conundrums are when AI replicates oppressive systems, mainly disadvantaging those already marginalized and replicating racism, sexism, classism, etc. Utilizing this framework, ethics in AI can be addressed from all angles and hopefully not only create more ethical AI but a more ethical world.
Between the lines
While completing this research and engaging with ethics in AI, the lack of societal ethics cannot be ignored. It is often easy to agree on something wrong, but agreeing on solutions is a lot more challenging. We hope that at least those who intend to build and deploy ethical AI systems will consider all angles and blind spots, including those who might be marginalized or harmed by the technology, especially when it aims to help. Most AI is utilized for consumerism and capitalism, such as algorithms that decide what ads to show us as we scroll. These are ethically shifty areas. On the far end, and what we omitted from this research, is AI being used for warfare or to purposefully harm or control populations. This is an extremely unethical use of technology. As Ani Difranco says, ‘Every tool is a weapon, if you hold it right.’ However, tools can also be used to build miraculous things and change the world. We hope by exploring different angles of ethics in AI that, it can continue to improve ethically as the technology develops.
References
[1] Allen, C., Smit, I., & Wallach, W. (2005). Artificial Morality: Top-down, Bottom-up, and Hybrid Approaches. Ethics and Information Technology, 7(3), 149–155. https://doi.org/10.1007/s10676-006-0004-4
Wallach, W., Allen, C., & Smit, I. (2008). Machine morality: bottom-up and top-down approaches for modeling human moral faculties. AI & SOCIETY, 22(4), 565–582. https://doi.org/10.1007/s00146-007-0099-0