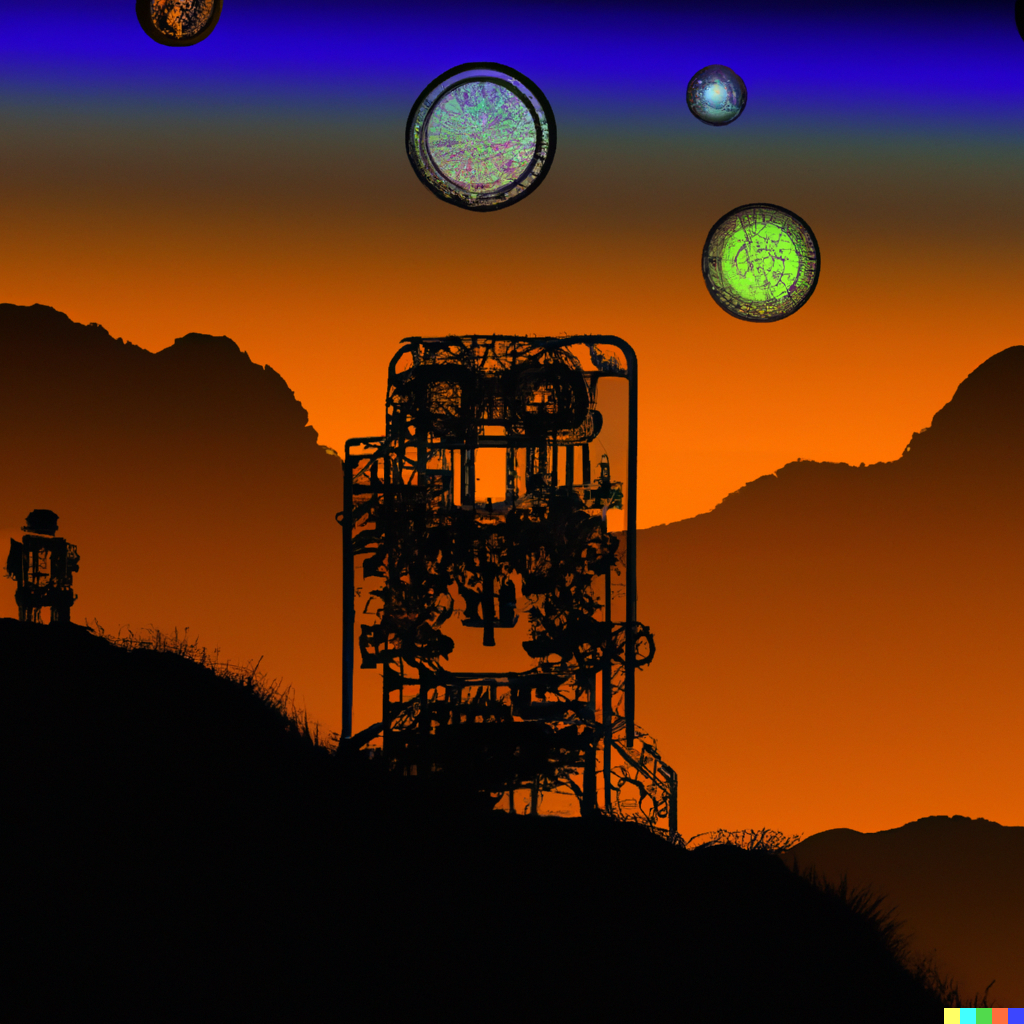
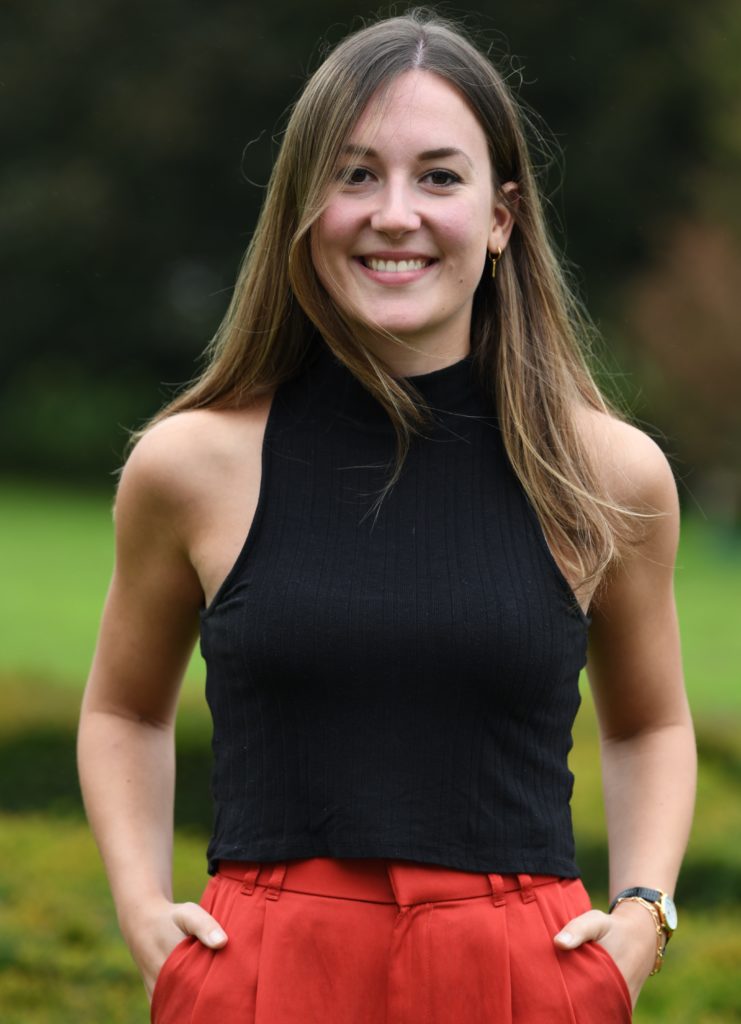
✍️ Column by Rosalie Waelen, a philosopher and AI ethicist, doing her Ph.D. at the University of Twente in the Netherlands.
Overview: When thinking about the ethics of AI, one usually considers AI’s impact on individual freedom and autonomy or its impact on society and governance. These implications are important, of course, but AI’s impact on the environment is often overlooked. This fourth column on the ethics of computer vision, therefore, discusses computer vision in light of environmental sustainability.
The sustainability of computer vision
Advancements in computer vision over the last decade, like all other recent advancements in AI, are usually ascribed to the use of deep learning algorithms. However, deep learning algorithms were only so successful at computer vision tasks because of increased computing power and the availability of large datasets of labeled images (ImageNet, in particular). A lot of computations are needed for a deep learning model to learn from large datasets. Each computation cycle costs energy. Furthermore, large datasets (or ‘big data’) must be stored in data centers. Running data center servers costs energy too, and the need to cool these servers can lead to high water consumption. So, because computer vision models are based on deep learning and because deep learning requires a lot of data and computing power, advancements in computer vision come with significant environmental costs.
It is difficult to find numbers on the exact ecological footprint of specific computer vision systems or the worldwide computer vision industry. Still, other studies into the sustainability of AI can give an idea. First of all, the yearly carbon footprint of data centers is estimated to be 100 megatons of CO2 emissions, which is around 2% of all global carbon emissions. Secondly, according to a study by Strubell et al. (2020), training a single NLP model can cost up to 313 lbs of CO2. Better AI systems imply larger training datasets, and larger training datasets, in turn, imply more computations and, thus, higher energy costs. For instance: GPT-3 was trained on 500 billion words instead of 40 billion words for GPT-2. In other words, the numbers regarding AI’s environmental impact can be expected to increase drastically in the next years.
In addition to the environmental cost of storing and processing data, one should consider the materials needed to create data servers and develop the hardware involved in computer vision applications, such as cameras.
Computer vision for sustainability
While the sustainability of computer vision itself is to be questioned, there are also numerous proposals for using computer vision to support sustainability efforts. This confuses terms like ‘sustainable AI’ or ‘sustainable computer vision’. To avoid such confusion, it is useful to draw a distinction between the ‘sustainability of AI’ and ‘AI for sustainability’ (van Wynsberghe, 2021).
One example of computer vision for sustainability is using computer vision to support the agricultural industry in meeting the (future) demand for sustainable food production. Computer vision could do this by monitoring crops, checking produce quality, or enabling robotics to harvest or package foods (Kakani et al., 2020). Other proposals include computer vision to track weather patterns and climate change, computer vision tools for monitoring fires or storms, and computer vision tools for waste management (Exxact, 9 December 2021).
Studies that suggest that computer vision can be used to support sustainability goals tend to overlook the environmental cost of developing and using computer vision models. In a recent article, Falk and van Wynsberghe (2023) acknowledge this is a common problem in the AI for sustainability literature. They challenge the label ‘AI for sustainability’, and suggest that it should be used exclusively when a technology contributes to a sustainable goal and the sustainability of the technology itself is considered.
AI ethics and environmental ethics
AI ethics and environmental ethics are two distinct fields of applied ethics. However, given the environmental costs involved in AI development and use, there is clearly an important overlap between the fields.
A common topic in environmental and animal ethics is the extension of the so-called moral circle to include the rights or well-being of animals, plants, or ecosystems. For AI ethics, expanding the moral circle would mean that AI’s impact on humans and society and its effect on other entities should be considered. However, considering the sustainability of AI is not just for the sake of the environment. It benefits humans too.
Van Wynsberghe (2021) suggests that it is time for a third wave of AI ethics, which puts AI’s environmental impact and sustainable development at its core. Questions for such a third wave of AI ethics are: What are the environmental costs of AI? How can these costs be reduced or avoided? Are the environmental costs of a system proportional to the benefits it brings? Under what conditions can we call AI sustainable?
Conclusion
Computer vision’s environmental impact is often overlooked in debates about the ethical implications of computer vision tools or their potential to support sustainability efforts. Perhaps this is the case because computer vision’s energy consumption is not very visible to the user. However, as I pointed out in this blog, the environmental impact of this technology is significant and likely to increase in the upcoming years.
Taking the ecological footprint of computer vision solutions seriously might sometimes imply refraining from using the technology altogether. Fortunately, a complete rejection of computer vision and other AI tools is not the only answer. There are various possible strategies to reduce the footprint of these technologies – such as building data centers underground or in other naturally cool places, choosing hardware more carefully, and increasing the efficiency of deep learning models (for more on this, see, for example, Sze et al., 2020).
References
Strubell, E., Ganesh, A., & McCallum, A. (2020). Energy and Policy Considerations for Modern Deep Learning Research. The Thirty-Fourth AAAI Conference on Artificial Intelligence.
Van Wynsberghe, A. (2021). Sustainable AI: AI for sustainability and the sustainability of AI. AI and Ethics, 1. 213-218.
Falk, S. & van Wynsberghe, A. (2023). Challenging AI for sustainability: what ought it mean? AI and Ethics.
Sze, V., Chen, Y. H., Yang, T.J., & Emer, J. (2020). Efficient processing of deep neural networks. Morgan & Claypool Publishers.
Kakani, V., Nguyen, V. H., Kumar, B. P., Kim, H., & Pasupuleti, V. R. (2020). A critical review on computer vision and artificial intelligence in food industry. Journal of Agriculture and Food Research.
Author unknown (9 December, 2021). How computer vision can help fight climate change. Exxact. Blog.