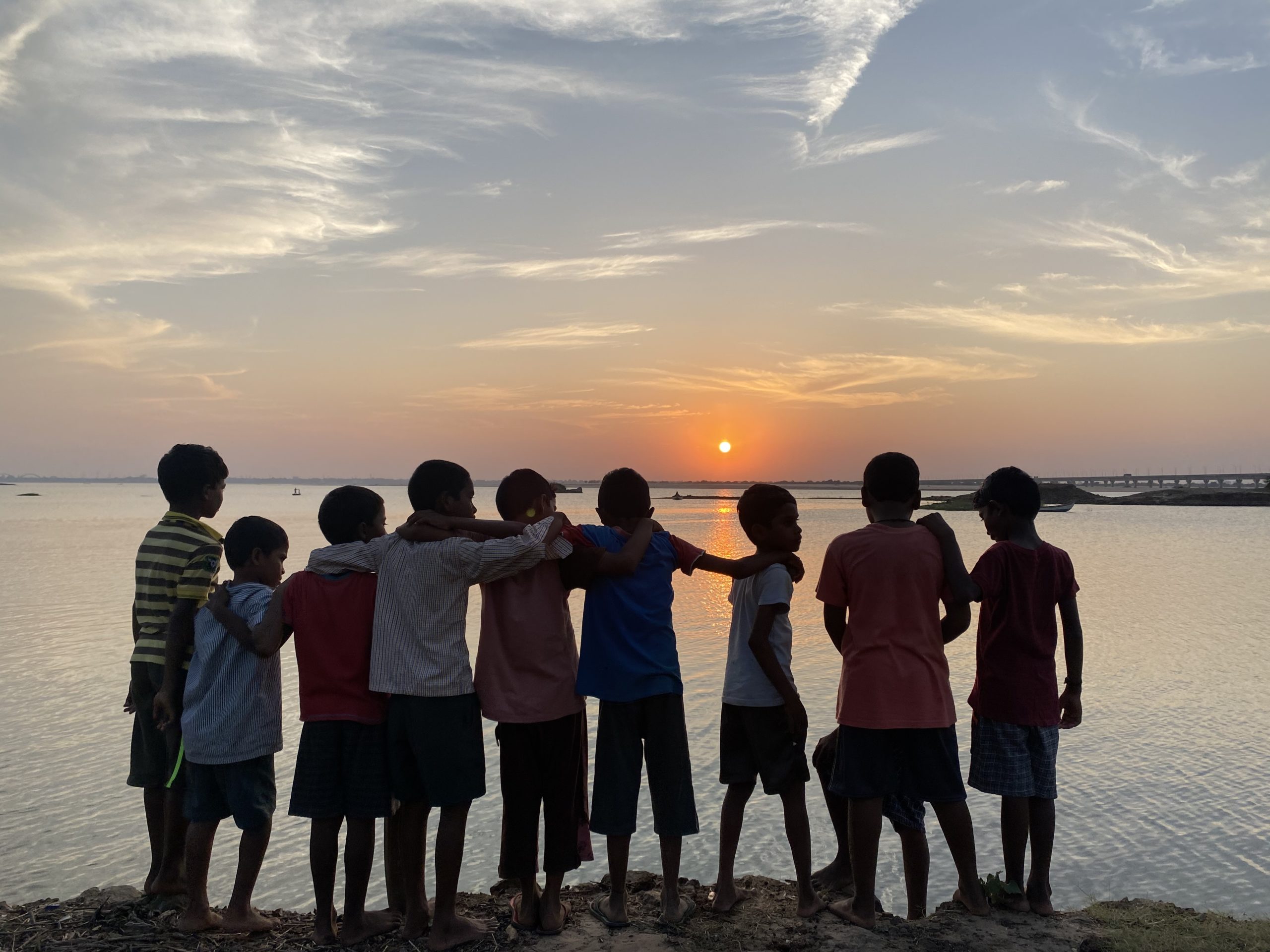
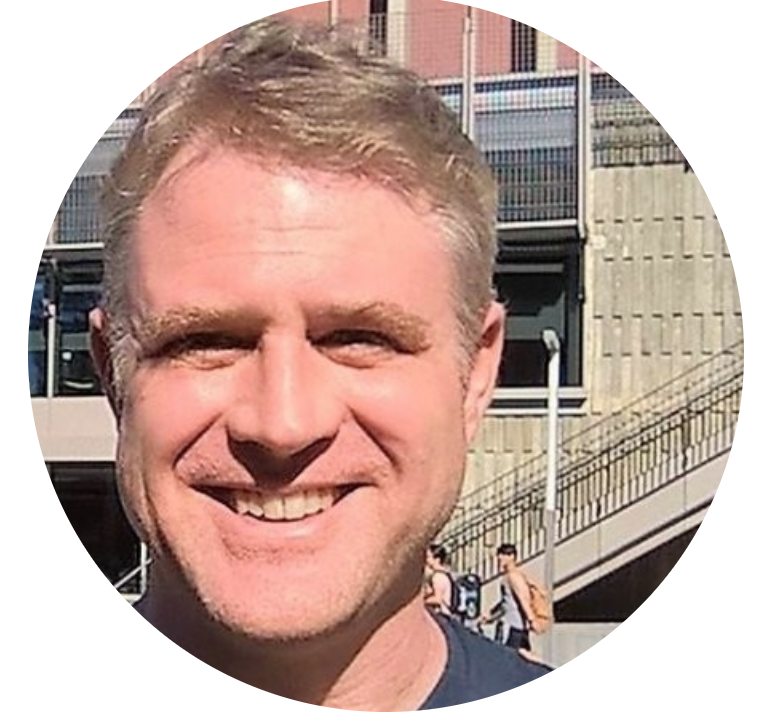
🔬 Research summary by Robert de Neufville who is the Director of Communications of the Global Catastrophic Risk Institute and a “superforecaster” with Good Judgment Inc.
[Original paper by Robert de Neufville and Seth D. Baum]
Overview: The development of safe and socially beneficial AI will require collective action, in the sense that outcomes will depend on the efforts of many different actors. This paper is a primer on the fundamental concepts of collective action in social science and a review of the collective action literature as it pertains to AI. The paper considers different types of AI collective action situations, different types of AI race scenarios, and different types proposed solutions to AI collective action problems.
Introduction
The development of safe and socially beneficial AI will require many different people working together. Social scientists have extensively studied different types of “collective action” situations that require actors to cooperate in some way to achieve the best outcomes for the group as a whole. How difficult it will be to achieve the best outcomes may depend on structural factors, like the extent to which the interests of individuals diverge from the interests of the group as a whole, the nature of the goods involved, and the degree to which they hinge on the efforts of a single actor or on some combination of different actors.
In this paper, we first present a primer on the theory of collection action and relate it to the different types of AI collective action situations. The paper looks in particular at AI race scenarios, which have been a major focus of the literature on AI collective action literature. AI races could hasten the arrival of beneficial forms of AI, but could be dangerous if individual actors rush development in order to be the first to develop a particular AI technology. Second, we review the three primary types of potential solutions to AI collective action problems: government regulation, private markets, and community self-organization.
Key Insights
Collective Action and AI issues
The impact of AI on society will ultimately depend on the actions of many different people and groups. In some cases, the interests of individual actors will align with the interests of society as a whole, so that good outcomes will result from individual actors pursuing their own interest. In other cases, some actors will be able to benefit individually from acting against the interest of society. In these cases, AI outcomes may depend on the extent to which the interests of individuals and society as a whole can be reconciled.
In public choice theory, collective action is required where outcomes depend on the actions of different people with different interests. Many aspects and applications of AI will require collective action. In particular, collective action will be needed (1) to reach agreement on rules and standards, (2) to develop AI that is broadly beneficial rather than merely profitable or otherwise advantageous for particular developers, and (3) to avoid competition or conflict that could lead to AI be developed or used in a way that is unsafe.
In recent years, a large but disparate literature has looked at the challenges of collective action with respect to AI. One important distinction is between coordination problems like the development of common AI platforms, in which individual and collective interests mostly align, and competitive situations like competitive AI races, in which individual and collective interests diverge. In general, collective action is easier to achieve when the interests of individuals align with the interests of the group. The type of collective action problem can in turn depend on whether the goods involved are “excludable” (that is, can be restricted to particular consumers) or “rivalrous” (that is, is used up when its benefits are enjoyed). Typically, the interests of individuals and the group are easy to align when goods are excludable—because their use can be limited to those who have paid for them in some sense—and non-rivalrous—because their supply is not limited. Another important issue is the degree to which addressing a collective action situation depends primarily on the effort of a single actor or requires many actors to contribute something.
One type of collective action situation that has received a lot of attention in the literature is AI race scenarios. AI races could be dangerous if individual actors’ interest in winning the race is at odds with the general interest in developing AI that is safe and socially beneficial. The paper looks at both near-term and long-term AI races. The literature identified in this paper focuses in particular on near-term races to develop military AI applications and long-term AI races to develop advanced forms of AI like artificial general intelligence and artificial superintelligence. The two types of races are potentially related since near-term races could affect the long-term development of AI.
Finally, the paper evaluates three different types of potential solutions to collective action problems: government regulation, private markets, and community self-organization. All three types of solution can address collective action problems, but no single approach is a silver-bullet solution to the entire range of collective action problems. It may be better to pursue a mix of different types of solution to address AI collective action in different ways and at different scales. Governance regimes will also need to account for other factors, like the extent to which AI developers are transparent about their technology.
Between the lines
The collective action issues raised by AI are increasingly pressing. Collective action will be necessary to ensure that AI serves the public interest rather than simply serving the narrow interests of those who develop it. Collective action will also be necessary to ensure that AI is developed with appropriate risk management protocols and adequate safety measures. The institutions we develop now to help resolve the AI collective action problems that arise today could have long-lasting and far-reaching consequences. The literature on AI collective action situations is still young; a great deal more work on designing systems to govern specific AI collective action problems still remains to be done.