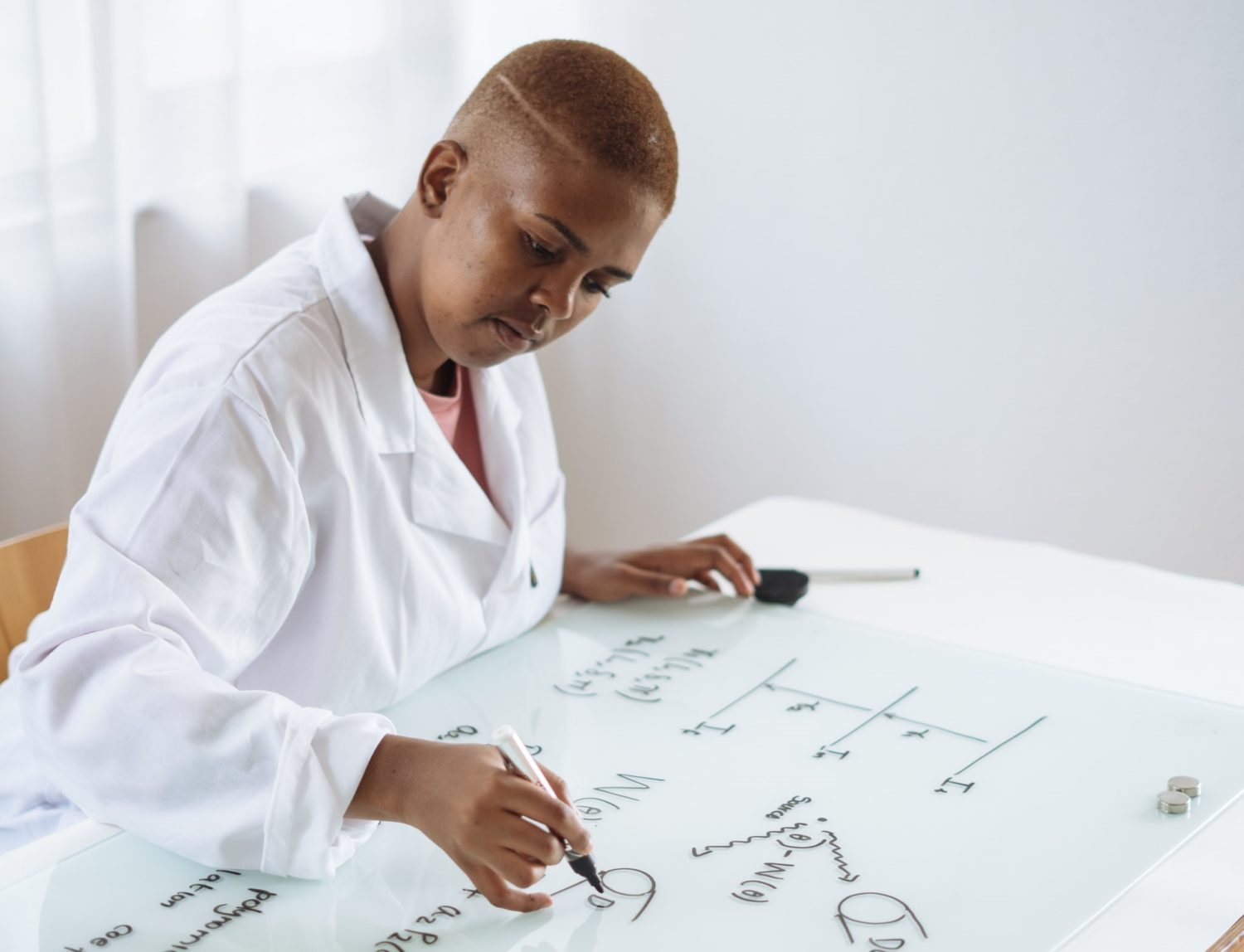
This event recap was co-written by Connor Wright (our Partnerships Manager), Alexandrine Royer (our Educational Program Manager), and Muriam Fancy (our Network Engagement Manager), who co-hosted our “Can We Engineer Ethical AI” virtual meetup in partnership with NEOACM earlier in February.
Room 1
We focused on the following 2 questions:
- Can licensing AI developers address most of the challenges of implementing AI ethics? How can AI ethics be inculcated into āprofessional ethicsā of engineers?
- What kind of metrics can be established that can measure the success of an ethical AI systems or tool?
Amongst the various discussions that could arise, one that immediately surfaced was a question about why we focus so much on AI ethics, AI bias, AI fairness and not such themes that run through technology in general? There are a multitude of ethical questions involved in the medical use of technology (such as if a pacemaker fails, who takes the blame?) so, why has AI gained the spotlight? From my view, I came up with 3 reasons:
- It affects more than just the target audience. AI is a novelty in multiple ways, but especially in the sense that it can negatively affect those who are situated outside of the data set, unlike normal tech. In this way, a pacemaker failing mainly affects the person who bears the technology, whereas if an AI system fails (such as straining already present social disparities), it affects more than those that are directly involved.
- AI is notoriously hard to regulate. Given its wide reach in terms of potential effects, making a law that covers all bases is made even more difficult. With so many social stratas, populations and potential harms to consider, itās no wonder that AI has caught the eye of many in the tech industry.
- AI is moving at an unparalleled pace compared to other forms of tech. It moves so quickly that the millions of dollars spent on acquiring a certain data set in 2018 goes down the drain in 2021 due to the data now being too out of date to be of any use.
Given AIās prominence, companies then started implementing their own ways of trying to tackle the problem and govern ethical AI behaviour. One of the ways can then be seen in companiesā use of codes of ethics.
Codes of ethics
In frank terms, the room came to the conclusion that, as one of our participants mentioned, codes of ethics in their current form are āpoppycockā. The ethical practices set up by organisations are seen as nice goals to have, but not really able to be acted upon, and I would agree. To put it succinctly, ethical guidelines are necessary but not sufficient. Guiding principles are required, but their influence over corporate practice and feasibility must also be considered. In this way, whether we could actually trust the corporate ability to come up with adequate codes of ethics that encourage genuine ethical intentions as opposed to regulations that suit the business, was raised by the room.
Questions were then pondered about what separates AI ethics from other relatively successful ethical practices, such as what medical ethics provides to doctors alike. In this regard, the room moved towards trying to answer just that.
AI and medical ethics
At first glance, it could be beneficial to compare the two given the frequent cross section between tech and the medical field. However, as is summarised in this research summary, medical ethics is a whole different ball game. Unlike in AI ethics, medical ethics boasts a duty of care to those affected while being judged on the outcome of their work. In AI ethics, the room brought up how AI provides its developers and practitioners a potential curtain to hide behind in being able to attribute some responsibility to the algorithmās own doings, rather than how they designed it, which isnāt present in the medical ethics field.
However, I observed how there was a disagreement within the discussion on this very point. Here, AI isnāt to be seen as different from the medical field seeing as if something goes wrong, you blame the doctor and not the technology used, so you should blame the developer and not the AI for a technological fault that leads to harm. As a result, our next big theme of the discussion appeared, the polemic topic of pushing for licensing for AI practitioners.
Licensing AI practitioners
Currently, there is no requirement for AI practitioners to be licensed. In this way, the room rightly observed a lack of understanding within the AI ethics debate on actually being able to tell if AI practitioners are actually complying with the ethical measures established in their place of work (no certification to prove it). While this is a potentially interesting idea to pursue, I believe, as the room mentioned, that there are several hoops to jump through first.
Even if this was a possibility, questions come to mind if this licensing is to be done state by state, city by city, or company by company. We already see a great divergence in state laws, so implementing a licensing system that stretches for AI practitioners in any context of the world or even just in the same country, is a tough feat indeed. Furthermore, one participant brought up how a side effect of licensing could be an increase in the price of education, given that such a certification would be a requirement and thus necesitate adequate funding. In this way, a discussion about exploring potential the locus of responsibility within the current AI landscape ensued.
Responsibility
The room was well prepared on how difficult it is to pinpoint responsibility within the AI process, a difficulty Iām all too aware of. AI going wrong may not be as a consequence of a developerās mistake, and a resultant slippery slope may ensue if we continuously blame humans involved in the process for problems they potentially canāt control nor did they foresee. Furthermore, the sheer number involved in the design and implementation process means that responsibility is often quite diffuse. This is why when such AI tech malfunctions, the finger usually points towards the company itself and not a single employee.
Nevertheless, participants still strove to highlight the, at the very least, possibility of tracing back those involved in the design of an AI system. Such a system is not born out of thin air and, as previously mentioned, involved plenty of people that can be identified. However, such identification will only be useful should those involved strive to change what led to their mistake,
a sole actor trying to do the right thing isnāt enough. To carry this out, the importance of a shared understanding of what is expected of such practitioners is required. Here enters the importance of a shared AI language, rightly surfaced by the room.
The importance of a shared language
A lack of agreed upon definitions make the ability to effectively regulate the AI space difficult, especially in the aforementioned codes of ethics. In this sense, itās unclear what terms such as āqualityā, āAIā, āethicalā and āsafeā actually mean, which could vary from company to company. As a result, determining whether an AI system is appropriately āsecureā starts to become localised to the codes of ethics that the company it derives from implements, whereby their definition of āsecureā could differ from another company. With engineering being a discipline with a particularly strong record of having well defined terms, this proves even more frustrating. Given this, many in the room concluded that before we can enter into AI ethics, more clarity on what is expected is required.
Room 2
The focus of room 2 was on understanding the roles and responsibilities of implementing ethical frameworks through the entire lifecycle of AI. The room discussed how these philosophical principles that define our reality to better analyze why engineering AI ethics continues to be such a pervasive problem.
By briefly discussing the four questions for the conference, the room came the following conclusions:
- Human beings operate on a spectrum, to classify data and analysis with a binary methodology will continue to perpetuate discriminatory practices in AI
- Furthermore, what defines human beings is our consciousness. Our consciousness contains our biases and therefore defines the filters we use to see the world. The disregard for how our consciousness drives our understanding of reality is to assume that technology can remain to be objective, which is untrue.
- Finally, to effectively engineer AI ethics frameworks in AI systems, we have to have diverse teams that exist across all stakeholders groups that are designing and deploying AI
To reach these conclusions the room organically discussed all four questions, outlined below:
- What can we do about vagueness and ambiguity in “ethics in AIā? How do we filter out the hype involved with “ethics in AI”?
- Can ethics be implemented as a component in computer systems?
- Can licensing AI developers address most of the challenges of implementing AI ethics? How can AI ethics be inculcated into āprofessional ethicsā of engineers?
- What kind of metrics can be established that can measure the success of an ethical AI systems or tool?
First discussing the risk of the hype on the issue of AI ethics and how that is negatively impacting how we discuss AI. Members noted that the issue isnāt the lack of discourse on AI ethics or even developing frameworks that should be deployed in AI, rather is how can we effectively include these frameworks in computer systems. The discussion was then framed on the issue on sourcing data, addressing how we can effectively source and classify data so that our practices of designing these systems are complying with the AI ethics frameworks that are currently existing.
Keeping Humans-in-the-Loop
However, before diving deeper into this topic, the group noted that AI systems should be used as tools, rather than objective governance systems that can deploy large scale decisions without a human in the loop. Due to this necessity of having a human in the loop, the group continued to question whether it is effective to launch these systems on such a large scale because of how much humans differ in their definition of ethics. Can these systems effectively capture the demographic it is serving? The answer most often will be no, which leads to the question of whether we should be deploying these systems?
Noting that AI can be used and designed for social good, there are opportunities for these systems to create societal impact. The moderators and the participants in the room questioned how we can reach this point? To which the group noted the importance of diversity of disciplines needed to design and deploy AI systems. A team of engineers cannot solely be responsible for the ethical oversight of AI systems. As one member aptly pointed out, we cannot treat AI systems, nor the engineers behind them, as all-knowing. An additional issue raised was that engineers are only made aware of ethics at a later stage in their careers. The room further pondered whether different ethical frameworks would thus be better suited to the varying stages of an AI systemsā lifecycle, not just during the initial design stage. If so, where would government regulation begin?
AI Ethics Taxonomy and Crowdsourcing
Certain attendees noted that third-party audits might be a more actionable way of ensuring socially-beneficial AI over vague government guidelines. Considering the wide-ranging AI applications, one attendee suggested developing an auditable taxonomy to describe different ethical violations by systems is preferable to setting in place ethical frameworks. A taxonomy could integrate a scale of the social and/or political harm caused by an algorithm.
The conversation shifted towards question 4 and the complexities of selecting the right metrics and institutions to evaluate AI systems. Members raised a potential solution of crowdsourcing as a form of third-party review. Many in the room agreed that multiplying stakeholder engagement in AI ethics review, including civil society, would avoid overly accounting for the opinions of a vocal, small percentage of people, what was referred to as āmob ruleā. Several in the room noted the lack of a proactive approach towards AI systems, as incidents of AI systems causing harm are only dealt with once they arise.
To resolve this problem, members suggested that AI developers label the potential risks and biases of their systems, natural language processing being one example raised, and make them visible to users. The room concluded that building trust in systems went back to the need of having a wide range of perspectives and individuals be consulted prior to the development of a system, especially when specific AI systems, such as emotional recognition, are so culturally contingent. Members in the room further agreed that AI systems cannot treat humans as existing in binaries, and instead need to be seen across a spectrum. Indigenous ontologies are one way of reimaging the workings and functions of AI systems.