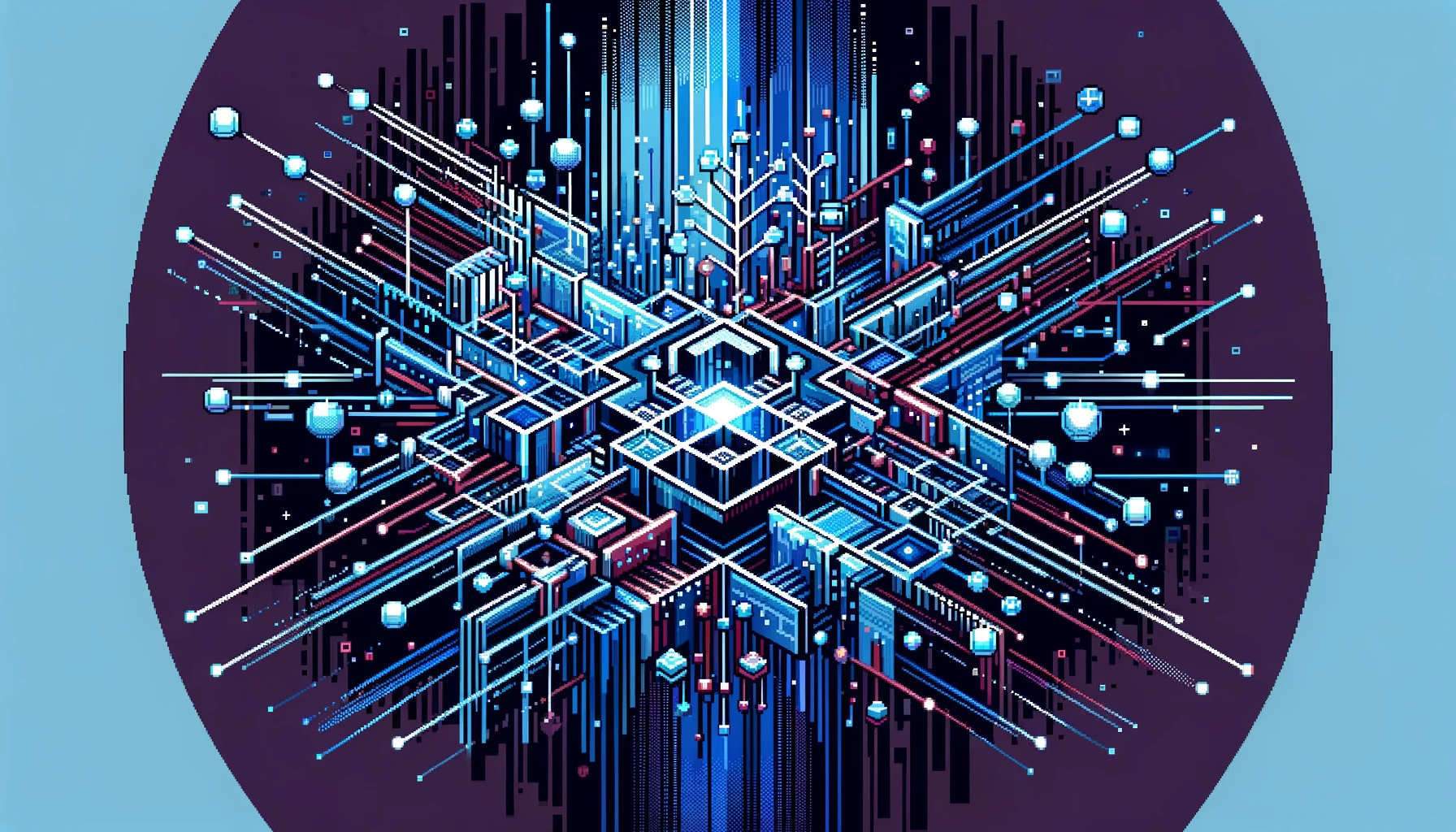
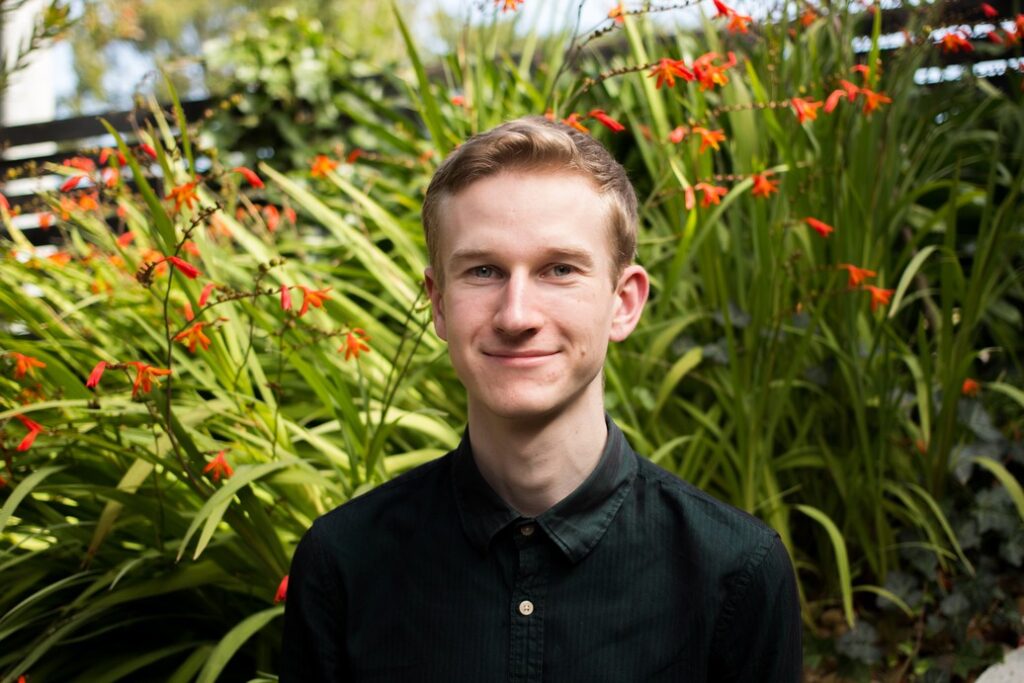
🔬 Research Summary by Luke Thorburn, a PhD student at King’s College London, where he works on the design of algorithms to mitigate conflict risks.
[Original paper by Aviv Ovadya and Luke Thorburn]
Overview: There is widespread concern about social divisions leading to political violence and a reduced capacity to respond to collective societal challenges. This paper explores how incentives for “bridging” — the building of mutual understanding and trust across divides — can be incorporated into algorithmic systems, particularly as “bridging-based ranking” in recommender systems on social media. It includes many concrete examples and open questions.
Introduction
Social media platforms have been implicated in conflicts of all scales, from urban gun violence to the storming of the US Capitol building on January 6 and civil war in South Sudan. Scientifically, it is difficult to tell how much social media can be blamed for one-off incidents. But in much the way that climate change increases the risk of extreme weather, evidence suggests that current algorithms (which mostly optimize for engagement) raise the political “temperature” by disproportionately surfacing inflammatory content. This may make people angrier, increasing the risk that social differences escalate to violence.
This blue sky paper surveys how incentives for “bridging” — the building of mutual understanding and trust across divides — can be incorporated into algorithmic systems that mediate human communication and attention. We give concrete examples of bridging across three domains: recommender systems on social media, collective response systems, and human-facilitated group deliberation, and develop a framework to help translate ideas between these seemingly disparate domains. We focus particularly on the potential of “bridging-based ranking” to bring the benefits of offline bridging into recommender systems on social media. Throughout, we list open questions.
Key Insights
The bridging goal
Conflict is an important part of society and, in many cases, a key driver of political and social change. For this reason, we suggest that the goal of bridging is not to eliminate conflict or disagreement, but to promote desirable forms of conflict. This is known as conflict transformation. Professional mediators, facilitators and “peacebuilders,” who work with opposing groups, have a detailed understanding of how conflicts escalate. They also know how to structure communication between opposing groups in ways that build mutual understanding and trust. Research on bridging can draw on this, taking insights from conflict management in the physical world and translating them into online settings. In the paper, we propose a general framework that can describe both offline and online systems that play a role in allocating human attention, and help translate insights between them.
How bridging-based ranking works
Recommendation algorithms on social media are the primary example of where bridging could be used online. Current engagement-based algorithms make predictions about which posts are most likely to generate clicks, likes, shares or views – and use these predictions to rank the most engaging content at the top of your feed. This tends to amplify the most polarizing voices, because divisive perspectives are very engaging. In contrast, bridging-based ranking uses a different set of signals to determine which content gets ranked highly. One approach is to increase the rank of content that receives positive feedback from people who normally disagree. This creates an incentive for content producers to be mindful of how their content will land with “the other side”.
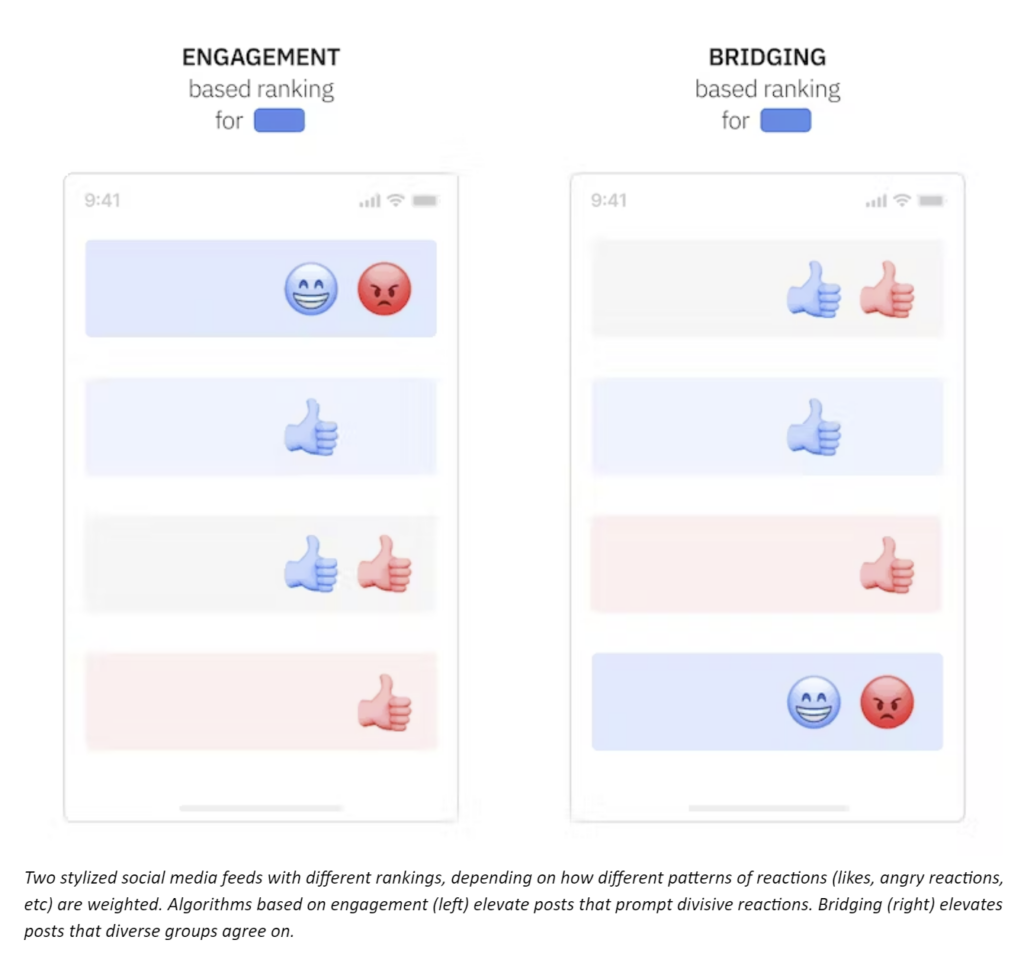
Among the internal Facebook documents leaked by whistleblower Frances Haugen in 2021, there is evidence that Facebook tested this approach for ranking comments. Comments with positive engagement from diverse audiences were found to be of higher quality, and “much less likely” to be reported for bullying, hate or inciting violence. A similar strategy is used in Community Notes, a crowd-sourced fact checking feature on X, to identify notes that are helpful to people on both sides of politics, and in Polis, an online platform for collecting public input, used by several governments to inform policymaking on polarized topics.
How to quantify bridging
This pattern of “diverse approval” is the most widely implemented approach to bridging, and the one currently most supported by evidence. But there are many other possible approaches to quantifying the bridging goal so that it can be used in algorithms. The paper surveys four approaches. One is to identify “motifs” — patterns of interaction, like the idea of “diverse approval” described above — which are thought to promote bridging. Others include surveying users, building algorithmic classifiers to detect bridging content, and using formal metrics designed to capture the state of conflict or polarization in a model of human relationships. Such quantitative signals and metrics can be used to optimize and evaluate algorithms, including recommender systems.
Challenges, limitations, risks
The idea of bridging systems is not free from challenges or controversy and should not be considered a panacea. There are many open questions, both practical and ethical. Which divides should be bridged? Are there unintended consequences – for example, amplifying mainstream views at the expense of minority viewpoints? How might bridging-based ranking be gamed by bad actors? To what extent are the objectives of bridging and engagement in tension with one another? How can decisions about the design and incentives of mass communication technologies be made democratically? Research and real-world deployments are needed to answer these and other questions.
Between the lines
Why would a profit-driven social media platform ever decide to use bridging-based ranking, when optimizing for engagement increases their ad revenue?
Fundamentally, we just don’t yet know the extent to which the goals of bridging and engagement are in tension. If you talk to people who work at social media platforms, they will tell you that when well-intended changes to the algorithm are tested, user engagement sometimes drops initially, but then slowly rebounds over time, ultimately ending up with more engagement. The problem is, platforms normally get cold feet and cancel experiments before they can observe such long-term benefits. Bridging might also have benefits for platforms beyond engagement, reducing the cost of content moderation, building goodwill with regulators, and avoiding reputation and legal damage.
There is only so much algorithmic changes can do to address societal conflict, a result of complex factors such as physical violence, economic inequality, and historical injustices. But by recognizing that digital platforms are reshaping society, we have an obligation to guide that process in an ethical, humanistic direction that brings out the best in us. To this end, there is increasing momentum behind the idea of bridging-based ranking, which was this year mentioned by the Editorial Board of The Washington Post and included in the USC Neely Center’s Design Code for Social Media.
To be kept up to date with this work, sign up for the mailing list at bridging.systems.