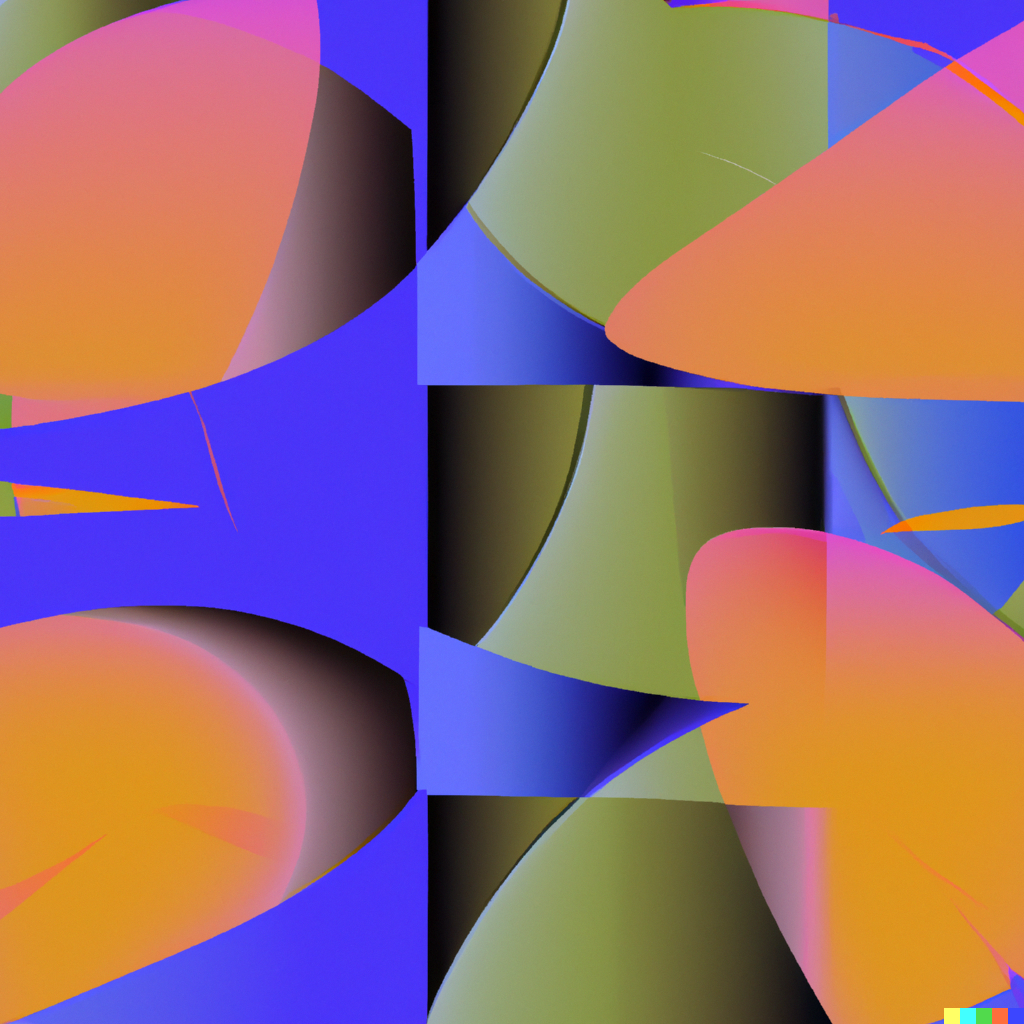
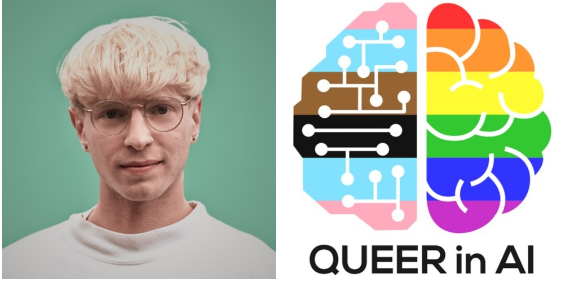
🔬 Research Summary by Nathaniel Dennler and Queer in AI.
Nathan is a Ph.D. candidate at the University of Southern California and a member of the Queer in AI organization; their personal work is in adapting robot behaviors to end-users.
Queer in AI works to raise awareness of queer issues in AI/ML and to foster a community of queer AI researchers.
[Original paper by Organizers of Queer In AI, Nathan Dennler, Anaelia Ovalle, Ashwin Singh, Luca Soldaini, Arjun Subramonian, Huy Tu, William Agnew, Avijit Ghosh, Kyra Yee, Irene Font Peradejordi, Zeerak Talat, Mayra Russo, Jess de Jesus de Pinho Pinhal]
Overview: AI systems are increasingly being deployed in various contexts; however, they perpetuate biases and harm marginalized communities. An important mechanism for auditing AI is allowing users to provide feedback on systems to the system designers; an example of this is through “bias bounties,” that reward users for finding and documenting ways that systems may be perpetuating harmful biases. In this work, we organized a participatory workshop to investigate how bias bounties can be designed with intersectional queer experiences in mind; we found that participants’ critiques went far beyond how bias bounties evaluate queer harms, questioning their ownership, incentives, and efficacy.
Introduction
Imagine you make a short video to post on social media detailing a day in your life on vacation. You may wake up, get food, and go to the beach. You may soon be shocked that your post is automatically flagged as violating the community guidelines because you describe your favorite restaurant as LGBTQ-friendly.
As AI systems become more prevalent, the potential to encounter AI biases and harms grows. One process that companies have employed in an effort to combat biases is a bias bounty.
The idea behind a bias bounty is that users of AI systems become bounty hunters. They enter a competition where they are tasked with locating biases that AI systems exhibit by interacting with the systems. An example of this is the bias bounty that Twitter ran to audit their saliency cropping algorithm. Participants in the bounty submitted scenarios where the cropping algorithm exhibited biased behavior (e.g., cropping out non-English characters) and were rewarded for winning submissions.
However, these bias bounties have several limitations–particularly for queer users. In bias bounties, the public rarely has a voice in what makes a winning submission, nor do companies provide mechanisms for interrogating their internal data and systems. Moreover, bounties are seldom transparent enough for participants to identify how system design choices may have led to biased behavior, let alone allow participants to challenge the political structures embedded in systems.
In our work, we hosted a workshop at the ACM FAccT conference where queer researchers in AI fairness could critique bias bounties. We analyzed the discussions and found four key topics to consider when designing bias bounties: Queer Harm, Control, Accountability, and Limitations.
Key Insights
We hosted two sessions of our workshop: one in-person session and one online session. Across the two sessions, we had nine discussion groups of 3-5 people per group. We performed an iterative thematic analysis across these nine discussion groups to understand the themes that workshop attendees addressed.
Queer Harms
Workshop participants discussed how people’s queer identities interact with their usage of AI systems. In particular, queer identities cannot be converted to fixed categorical representations. Queer identities evolve over time and develop as people learn more about themselves and how they experience the world.
Because queer identities can be unique, participants commented on how ensuring everyone is properly considered is difficult. One concern is that queer people are often not a majority of users. If bias bounties only address the most common or widespread instances of bias, queer people’s concerns may go unaddressed.
In addition to not feeling like a majority, participants discussed how two aspects of queer identity are important to consider when collecting user information. First, queer identities resist categorization. Often, queer people are presented with a list of options to identify themselves, but it lacks their true identifiers. This leads to their erasure. The second aspect of queer identity is that it is constantly refined over time. As people learn more about themselves, their identities may change, and AI systems must have ways to incorporate these changes.
Furthermore, even the mere act of participating in bias bounties can pose harm to queer people. For example, participants noted how being recognized for finding biases could potentially out someone, especially if the biases reflect their queer identity. Another issue of participating in bounties is that queer users can be exposed to psychologically-harmful instances of queerphobia.
Control
Another question in the workshop arose several times: who controls bias bounties? Participants were wary that relying solely on the users that companies intend their systems to serve may lead to only already-privileged groups being heard.
A key concern was around the community guidelines used to run bias bounties. These standards might make it impossible for queer people to report biases. For example, some platforms consider the discussion of sexuality and sexual content to not align with community guidelines.
Moreover, participants were concerned that the people in control of bounties may only allocate resources to fix the problems that affect them; this would make it difficult to have queer biases addressed.
Accountability
Several workshop participants were concerned that the companies who own the AI system being audited during a bias bounty may not be the best director of the bounty. In particular, companies may only run bias bounties to appear to, but not actually, solve issues with their system.
Discussions at the workshop brought up an alternative to company-run bias bounties: community-run bias bounties. By having community-run bias bounties, communities can provide more actionable feedback on AI systems. Communities can also advocate for the red-lighting of inappropriate AI systems entirely before development begins.
Limitations
Participants touched on two fundamental limitations of bias bounties. Bias bounties can only identify biases that should be fixed later. There is no guarantee that biases will be fixed in a timely manner or even fixed at all. The second limitation is that bias bounties may have a high barrier to entry for general populations. Bounties currently require a large amount of technical expertise that may be unrealistic to expect from many users. This requirement limits both the diversity of bounty participants and the diversity of submissions.
Between the lines
Our work found several considerations to be made when auditing AI systems. The discussions with participants revealed that bias bounties can only really address problems that come up after the AI system is deployed. However, there are four places where auditing processes could shape how AI systems are developed.
First, auditing processes could evaluate the applicability of AI systems for certain contexts. Before any technical development is started, a community of queer people could evaluate whether proposed AI solutions could feasibly be helpful and not harmful.
Second, auditing processes could target the data collection phase. These processes evaluate that the data being collected to be used for AI systems is being done reasonably. In particular, the data collection allows queer identities to exist outside of categorization and allows them to change over time.
Third, auditing processes can examine how the AI system is being developed. At this stage, it is important to be able to understand how the AI system may change what users can do. For example, does the AI system prohibit users from doing things that they find meaningful?
Finally, auditing processes can examine the AI system post-deployment. This is where bias bounties help the most. They can identify where small changes should be made in AI systems but cannot assess systems as efficiently at earlier stages of development.
To address audits at all phases of AI system development, our workshop attendees stressed the importance of community ownership. By introducing a third-party, workshop attendees hoped to moderate the power disparities between the companies that develop AI systems and marginalized communities that use these systems. By having companies engage deeply with marginalized communities through co-design efforts and continuous openness to criticism, we want to move closer to building equitable AI systems.