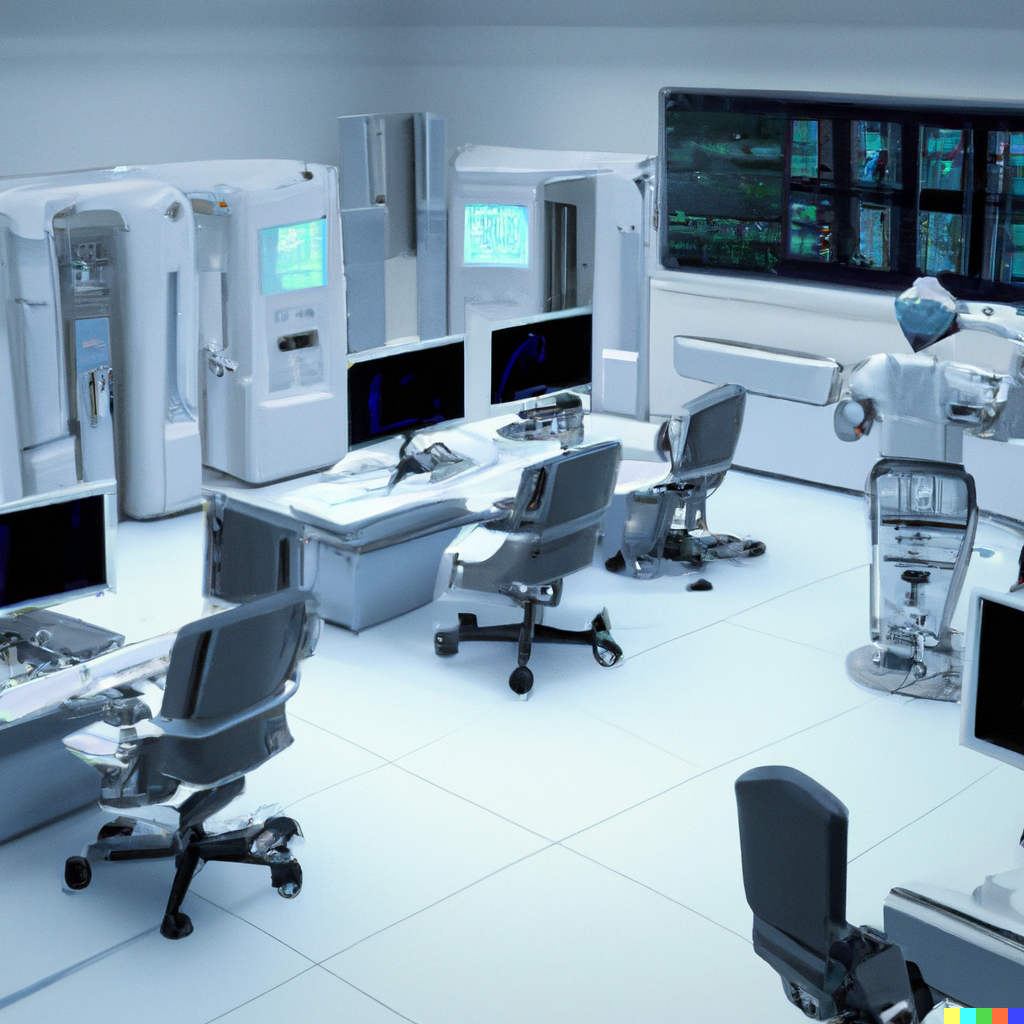
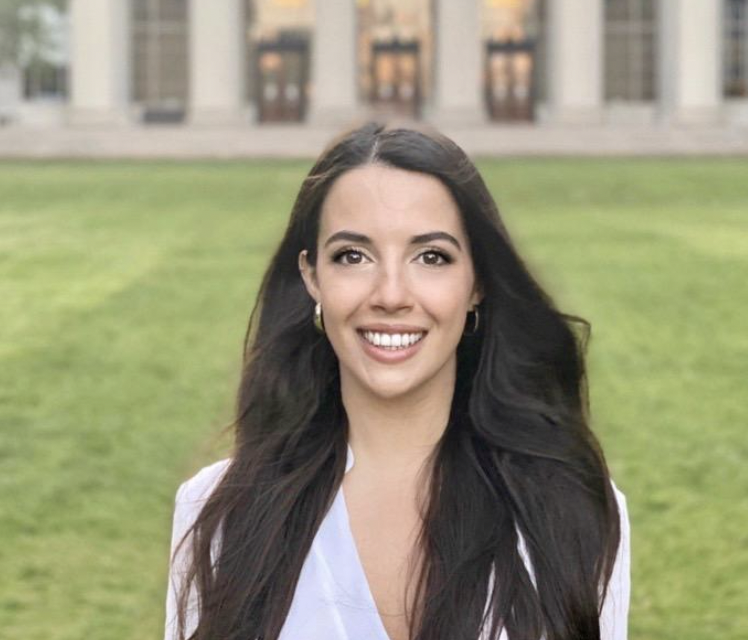
🔬 Research Summary by Simona Tiribelli, an Assistant Professor in Ethics at University of Macerata, Director of AI Ethics at the Institute for Technology and Global Health, and Head for AI Ethics of the WHO-ITU AI4H FG on Outbreaks
[Original paper by Benedetta Giovanola and Simona Tiribelli]
Overview: The increasing implementation of ML algorithms in healthcare has made the need for fairness in healthcare ML algorithms (HMLA) a very urgent task. However, while the debate on fairness in the ethics of AI has grown significantly in the last decade, the concept of fairness as an ethical value has not yet been sufficiently explored thus far. This paper draws on moral philosophy to fill this gap. It shows how an ethical inquiry into the concept of fairness helps highlight shortcomings in the current conceptualization of fairness in HMLA and better redefine the AI ethics principle of fairness to design fairer HMLA.
Introduction
Is the current conceptualization of the AI ethics principle of fairness adequate to design fair HMLA? To answer this question, the authors of this paper first provide an overview of the discussion of fairness in HMLA and show that the concept of fairness underlying this debate is framed in purely distributive terms and overlaps with non-discrimination, understood as bias-free HMLA. They question whether the idea of fairness so understood is adequate for the discussion on fairness in HMLA or whether the latter calls for a more complex concept of fairness that requires more than just non-discrimination and an exclusively distributive dimension and that includes features and criteria that extend beyond the consideration of biases. Drawing insights from moral philosophy, they propose a more complex account of fairness as an ethical value based on a renewed reflection on the concept of respect. In particular, they argue that fairness as an ethical value has both a distributive and a socio-relational dimension. It comprises three components: fair equality of opportunity, difference principle, and equal right to justification. Finally, they show how each of the components highlighted points to criteria that ought to be respected to operationalize fairness via HMLA.
Key Insights
Fairness in HMLA: non-discrimination and absence of bias
While fairness is one of the most discussed topics in the debate on HLMA, the authors argue how fairness is mainly conceptualized as non-discrimination, which is in turn framed as a state of absence of biases in HLMA and operationalized via the removal of four specific kinds of biases, respectively, on model design, training data, interactions with clinicians and interactions with patients. Moreover, the discrimination triggered by HMLA is mainly understood as being intertwined with unfair distribution. At the same time, fairness in HMLA is generally considered to be reached when ‘distributive justice options’ are considered: a model is considered to be fair when its outcomes, performances, or effects on patients do not produce discrimination among groups. Therefore, the widespread idea is that by eliminating biases in HLMA, it would be possible to mitigate or fix algorithmic discrimination and develop fair HMLA. This understanding of fairness translates into solutions to ensure fairness in HMLA that mainly coincide with neutral or parity models designed to produce non-discriminatory predictions by constraining biases with respect to members of protected groups. Nevertheless, beyond the shown incompatibility between ‘distributive justice options,’ this understanding of fairness has been criticized for relying excessively on technical parity and dataset neutrality achievable through the elimination of references to protected groups’ identities, which is deemed controversial in the health domain, where variables such as gender and ethnicity are crucial for accurate predictions. Authors question whether focusing on bias removal alone can guarantee fair HLMA. To answer this question, the authors examine whether this concept of fairness is adequate or whether a more complex ethical inquiry into fairness is needed to understand what fairness as an ethical value truly demands.
Fairness as an ethical value: fair equality of opportunity, the difference principle, and equal right to justification
The authors draw on moral philosophy to investigate the concept of fairness as an ethical value, basing their argument on a renewed reflection on the idea of respect. First, they clarify that, although strictly interconnected, fairness does not overlap with non-discrimination. Discrimination hinders fairness, since it is based on the misrecognition of people’s moral equality and implies that some of them are treated in a cruel or humiliating therefore disrespectful way. However, fairness also includes other dimensions and constitutive components. The first two components of fairness are fair equality of opportunity (FEO) and the difference principle (DP), strongly claimed within liberal-egalitarian theories of justice, starting with the Rawlsian one. FEO and DP regulate the distribution of benefits and burdens of social cooperation and the management of socio-economic inequalities in a way not only to prevent discrimination but to create the conditions allowing the exercise of individual agency, with attention to the conditions of the least advantaged, not only to access but also to enjoy opportunities.
They express a distributive dimension of justice based on the need to respect people as recipients of distribution and as subjects capable of agency. A third element is an equal right to justification (ERJ), claimed by social justice leading scholars such as Rainer Forst. ERJ expresses the ethical demand that no relationship should exist that cannot be adequately justified towards those involved; it points to the need for intersubjective relations and structures that protect every person’s status and capability to make up their minds on issues of concern. ERJ expresses a socio-relational dimension of fairness: it rests on a principle of mutual justification based on the import of respecting each person as a subject capable of (and entitled to) offer and request justification. Indeed, the question of the ERJ is also a question of power, namely, who decides what.
Fairness revised in HLMA
The authors show from a theoretical and practical standpoint how the components of fairness highlighted point to conditions that ought to be operationalized to implement fairness effectively in HLMA. For example, FEO and DP specify the requirements that ought to be met to promote a fair distribution of resources and access to opportunities via HMLA. Ensuring FEO and DP in HMLA requires the design of compensatory tools that, rather than only fixing pernicious biases, are thought and used to detect and mitigate social disparities that are deeply rooted in our society, hampering individuals’ capacity to fairly enjoy opportunities, with specific attention to the least advantaged (see the paper to expand theory and technical suggestions on how to operationalize these conditions).
While the ERJ expresses the duty of tech designers and decision-makers to promote the design and implementation of HMLA that can guarantee persons’ right to know how they are profiled and algorithmically treated (e.g., based on what information) and to social support (structures and assistance) when a request of justification is not fulfilled or contestation is claimed against HMLA’s outcomes. Ensuring ERJ can help mitigate asymmetries of power, allowing people to act against epistemic injustice HMLA can produce and to participate in the modeling of HMLA as novel health determinants.
Between the lines
This paper contributes to the AI ethics debate on fairness at both conceptual and practical levels. At the conceptual level, the paper unpacks the concept of fairness from an ethical standpoint by filling a dearth of literature and clarity on what fairness as a core AI ethics principle demands, highlighting dimensions and components of fairness ignored in the debate on HMLA. This is valuable as disambiguating ethical values informing AI ethics principles prevents using the latter as mere labels, thus from risks of ethics washing and bashing.
At the practical level, authors provide helpful suggestions for the design of fair HMLA, inviting future research to not only focus on bias-mitigation techniques but also to develop novel technical and policy-oriented tools that can promote the fundamental dimensions and components of fairness highlighted. Finally, the revision of the AI ethics principle of fairness in HMLA proposed shows that HMLA can contribute to a fairer healthcare ecosystem and the broad promotion of a fairer society. As far as HLMA can contribute both to a fairer distribution of opportunities for all, especially for the worst-off, to the creation of a society of equals, and to respecting every person, HMLA contributes to the social good of society, where the social good entails that every person is recognized as equal and given fair opportunities and power in knowledge and action.